Hessian regularization based non-negative matrix factorization for gene expression data clustering
EMBC(2015)
摘要
Since a key step in the analysis of gene expression data is to detect groups of genes that have similar expression patterns, clustering technique is then commonly used to analyze gene expression data. Data representation plays an important role in clustering analysis. The non-negative matrix factorization (NMF) is a widely used data representation method with great success in machine learning. Although the traditional manifold regularization method, Laplacian regularization (LR), can improve the performance of NMF, LR still suffers from the problem of its weak extrapolating power. Hessian regularization (HR) is a newly developed manifold regularization method, whose natural properties make it more extrapolating, especially for small sample data. In this work, we propose the HR-based NMF (HR-NMF) algorithm, and then apply it to represent gene expression data for further clustering task. The clustering experiments are conducted on five commonly used gene datasets, and the results indicate that the proposed HR-NMF outperforms LR-based NMM and original NMF, which suggests the potential application of HR-NMF for gene expression data.
更多查看译文
关键词
Hessian matrices,biology computing,genetics,learning (artificial intelligence),matrix decomposition,pattern clustering,HR-based NMF algorithm,Hessian regularization based nonnegative matrix factorization,LR-based NMM,Laplacian regularization,clustering analysis,clustering task,data representation method,gene expression data clustering technique,machine learning,traditional manifold regularization method,weak extrapolating power,
AI 理解论文
溯源树
样例
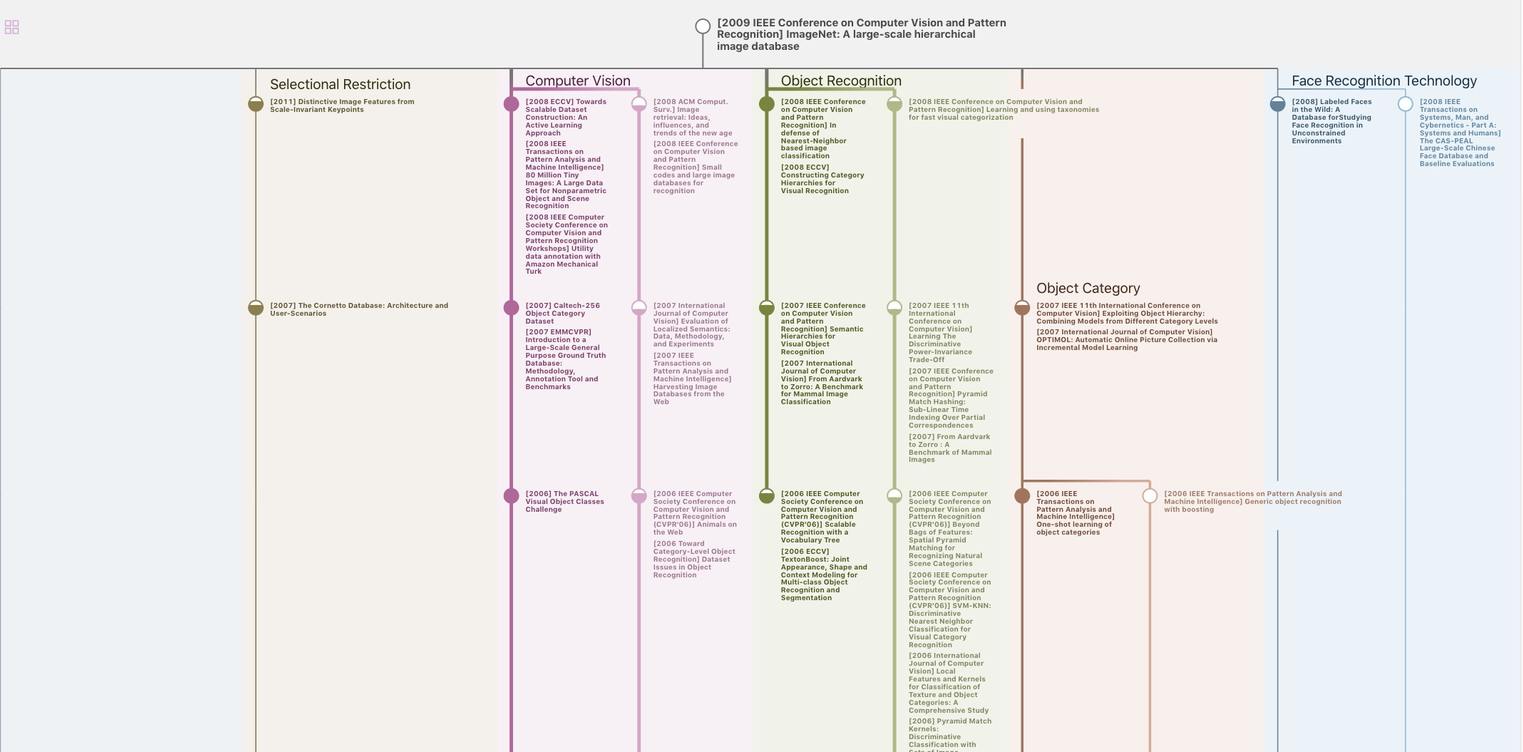
生成溯源树,研究论文发展脉络
Chat Paper
正在生成论文摘要