An LDA-based Topic Selection Approach to Language Model Adaptation for Handwritten Text Recognition.
RANLP(2015)
摘要
Typically, only a very limited amount of in-domain data is available for training the language model component of an Handwritten Text Recognition (HTR) system for historical data. One has to rely on a combination of in-domain and out-ofdomain data to develop language models. Accordingly, domain adaptation is a central issue in language modeling for HTR. We pursue a topic modeling approach to handle this issue, and propose two algorithms based on this approach. The first algorithm relies on posterior inference for topic modeling to construct a language model adapted to the development set, and the second algorithm proceeds by iterative selection, using a new ranking criterion, of topic-dependent language models. Our experimental results show that both approaches clearly outperform a strong baseline method.
更多查看译文
AI 理解论文
溯源树
样例
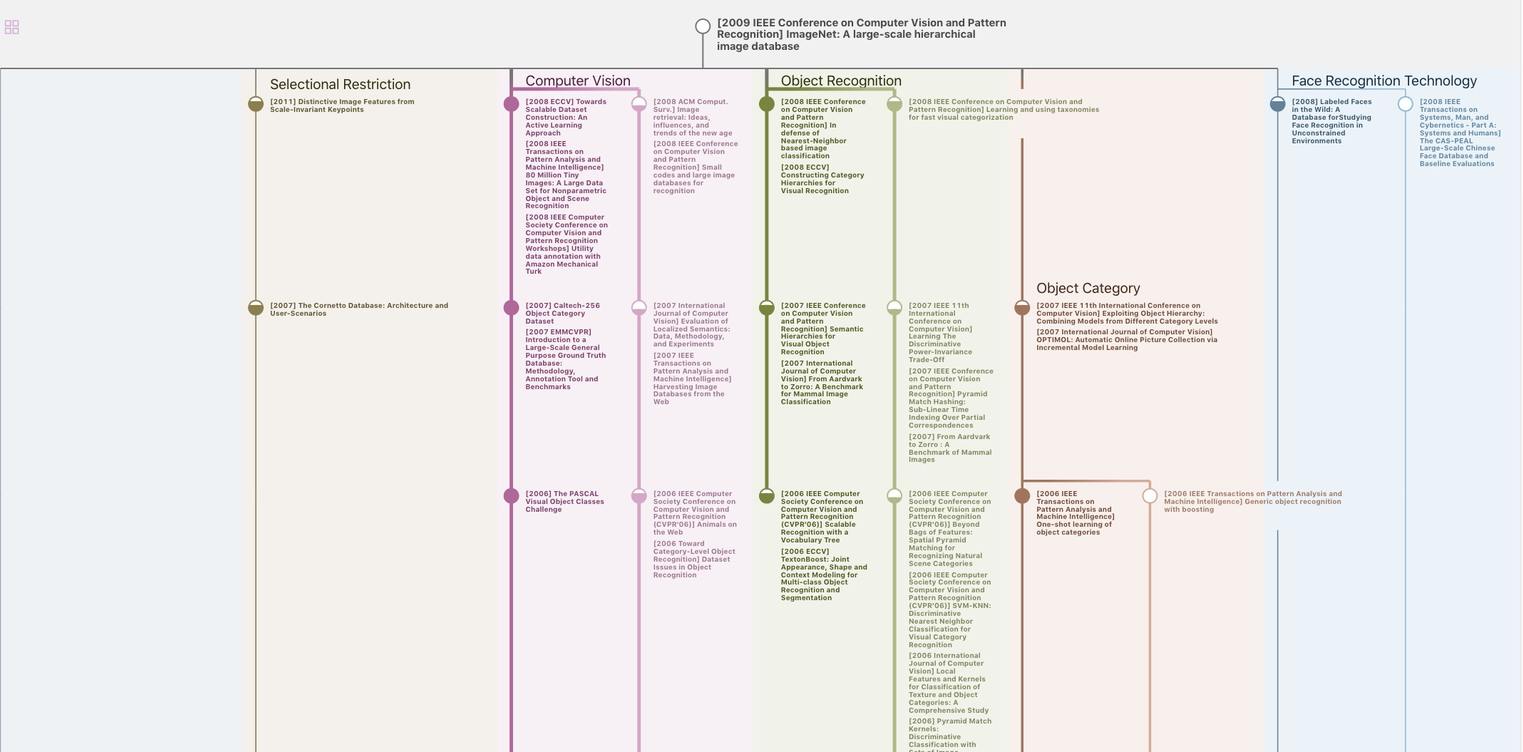
生成溯源树,研究论文发展脉络
Chat Paper
正在生成论文摘要