A fast handwritten numeral recognition framework based on peak densities
2015 IEEE CHINA SUMMIT & INTERNATIONAL CONFERENCE ON SIGNAL AND INFORMATION PROCESSING(2015)
摘要
In this paper, we present a novel framework for handwritten numeral recognition. Considering unconstrained handwritten numerals as numeral feature vectors in the corresponding numeral vector space, we commence by reducing the coordinate dimensionalityof vector space by employing Spectral Regression Discriminant Analysis (SRDA). We then calculate the local density for all numeral classes. For each class, we consider numeral points with local peak densities and large distance from points with higher peak densities as the numeral centers. For the inference tasks, we calculate the average similarity between one testing numeral sample and numeral centers of each digit class. The largest average similarity with numeral centers of one digit class implies that the numeral sample is categorized into this class. In order to validate our framework, experiments with two worldwide standard data sets USPS and MNIST are performed. The experimental results show that the proposed approach achieves high performances in efficiency and robustness for big data analysis.
更多查看译文
关键词
Spectral Regression Discriminant Analysis (SRDA), peak densities, handwritten numeral recognition
AI 理解论文
溯源树
样例
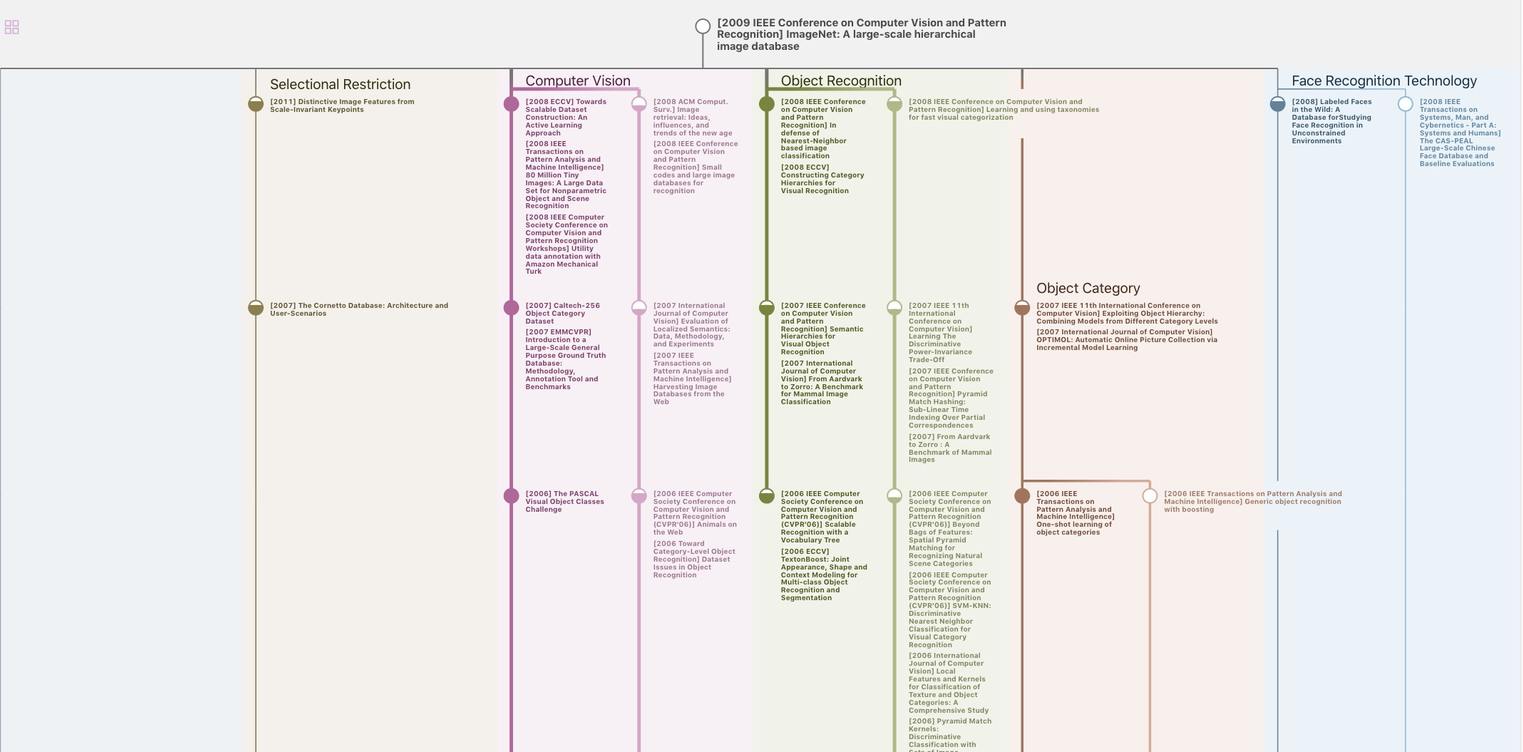
生成溯源树,研究论文发展脉络
Chat Paper
正在生成论文摘要