Resource and Performance Distribution Prediction for Large Scale Analytics Queries.
ICPE(2016)
摘要
Efficient resource consumption and performance estimation of data-intensive workloads is central to the design and development of workload management techniques. Recent work has explored the efficacy of using distribution-based estimation of workload performance as opposed to single point prediction for a number of workload management problems such as query scheduling, admission control, and the like. However, the proposed approaches lack an efficient workload performance distribution prediction in that they simply assume that the probability distribution function (pdf) of the target value is already available. This paper aims to address this problem for an inseparable portion of big data analytics workloads, Hive queries. To this end, we combine knowledge of Hive query executions with the novel usage of mixture density networks to predict the whole spectrum of resource and performance as probability density functions. We evaluate our technique using the TPC-H benchmark, showing that it not only produces accurate pdf predictions but outperforms the state of the art single point techniques in half of experiments.
更多查看译文
关键词
Query performance prediction, Distribution prediction, Hive
AI 理解论文
溯源树
样例
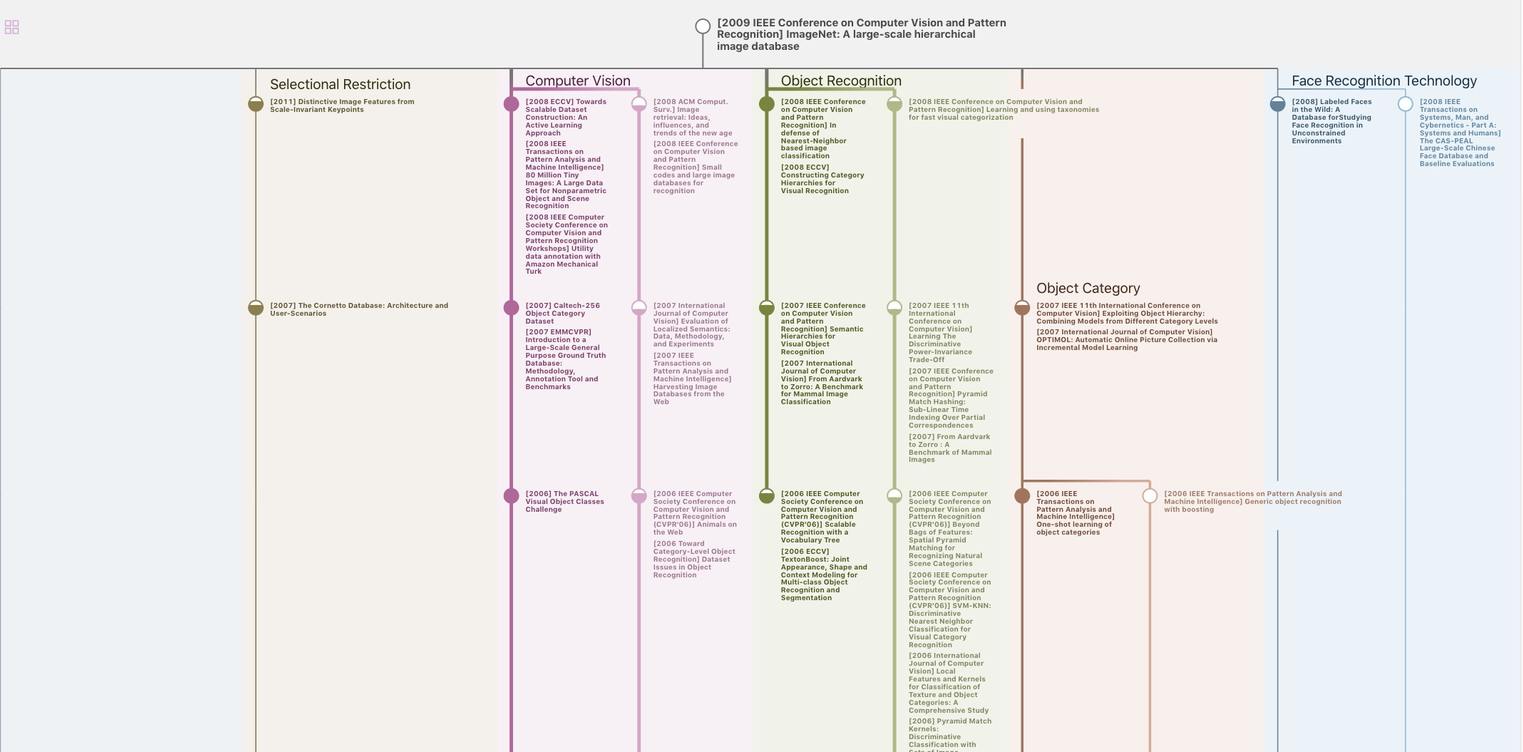
生成溯源树,研究论文发展脉络
Chat Paper
正在生成论文摘要