Resource Distribution Estimation for Data-Intensive Workloads: Give Me My Share & No One Gets Hurt!
ADVANCES IN SERVICE-ORIENTED AND CLOUD COMPUTING (ESOCC 2015)(2016)
摘要
Robust resource share estimation of data-intensive workloads is integral to efficient workload management in a (virtualized) cluster where multiple systems co-exist and share the same infrastructure. However, developing a reliable resource estimator is quite challenging due to (i) heterogeneity of workloads (e.g. stream processing, batch processing, transactional, etc.) in a multi-system shared cluster, (ii) limited (in batch processing) or complete uncertainties (in stream processing) on input data size or arrival rates, and (iii) changing configurations from run to run. To address above challenges, we propose an inclusive framework and related techniques for workload profiling, similar job identification, and resource distribution prediction in a cluster. Our analysis shows that the framework can successfully estimate the whole spectrum of resource usage as probability distribution functions for wide ranges of data-intensive workloads.
更多查看译文
关键词
Resource estimation,Big data workload,Multi-cluster workload management,Distribution prediction,Data-intensive systems
AI 理解论文
溯源树
样例
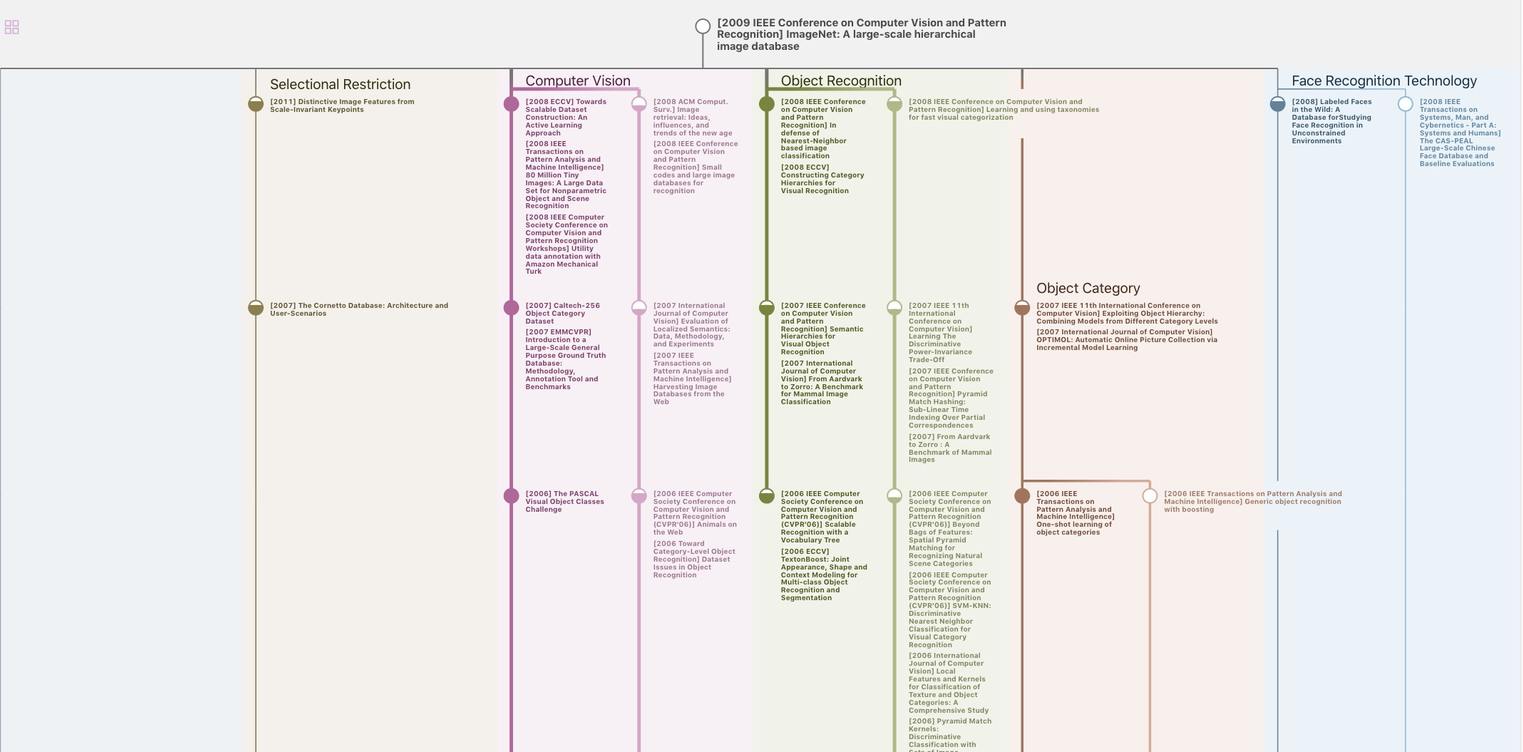
生成溯源树,研究论文发展脉络
Chat Paper
正在生成论文摘要