Just Noticeable Differences In Visual Attributes
2015 IEEE International Conference on Computer Vision (ICCV)(2015)
摘要
We explore the problem of predicting "just noticeable differences" in a visual attribute. While some pairs of images have a clear ordering for an attribute (e.g., A is more sporty than B), for others the difference may be in-distinguishable to human observers. However, existing relative attribute models are unequipped to infer partial orders on novel data. Attempting to map relative attribute ranks to equality predictions is non-trivial, particularly since the span of indistinguishable pairs in attribute space may vary in different parts of the feature space. We develop a Bayesian local learning strategy to infer when images are indistinguishable for a given attribute. On the UT-Zap50K shoes and LFW-10 faces datasets, we outperform a variety of alternative methods. In addition, we show the practical impact on fine-grained visual search.
更多查看译文
关键词
just noticeable differences prediction,visual attributes,relative attribute mapping,attribute space,feature space,Bayesian local learning strategy,UT-Zap50K shoes dataset,LFW-10 faces dataset,fine-grained visual search
AI 理解论文
溯源树
样例
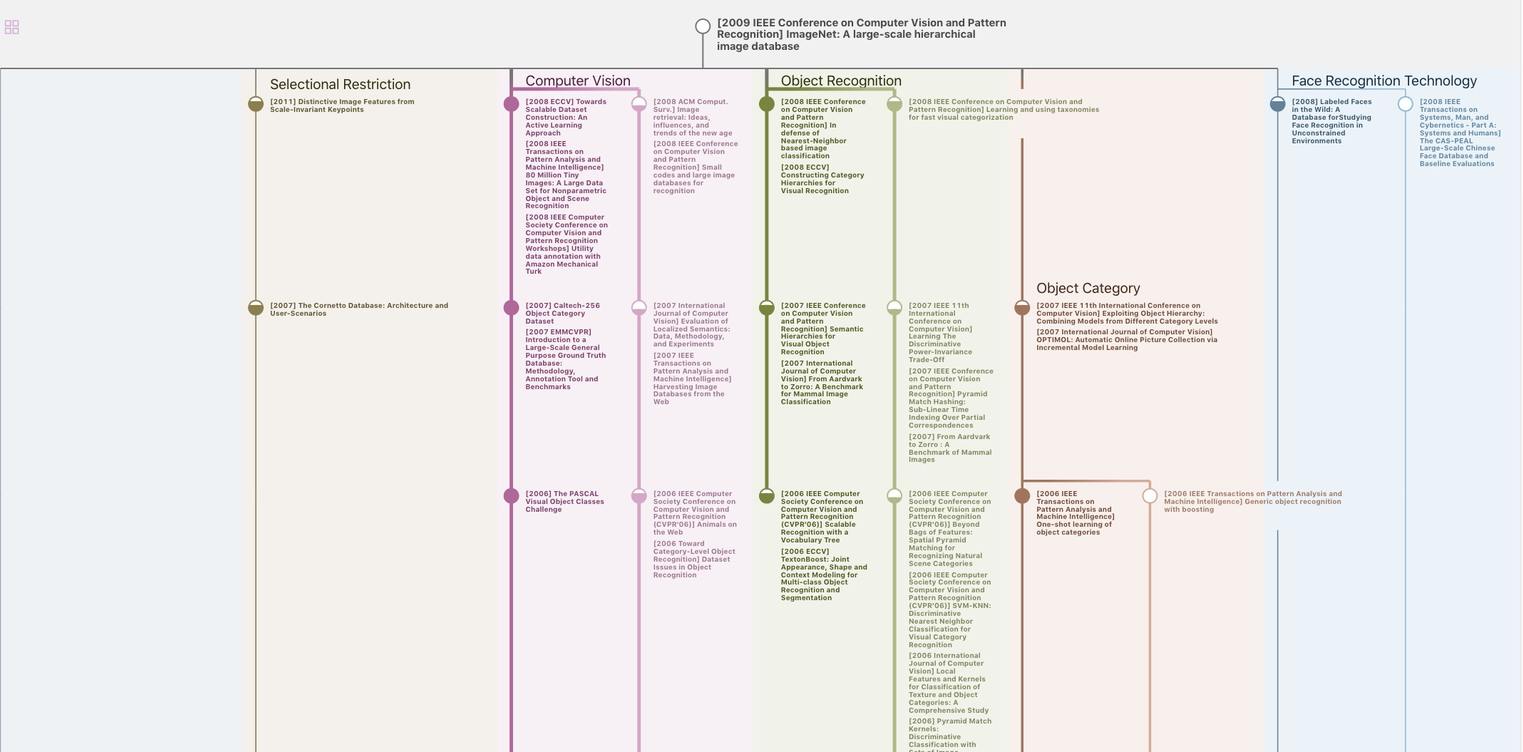
生成溯源树,研究论文发展脉络
Chat Paper
正在生成论文摘要