A Parallel Method for Scalable Anonymization of Transaction Data
International Symposium on Parallel and Distributed Computing(2015)
摘要
Transaction data, such as market basket or diagnostic data, contain sensitive information about individuals. Such data are often disseminated widely to support analytic studies. This raises privacy concerns, as the confidentiality of individuals must be protected. Economization is an established methodology to protect transaction data, which can be applied using different algorithms. RBAT is an algorithm for anonymzitng transaction data that has many desirable features. These include flexible specification of privacy requirements and the ability to preserve data utility well. However, like most economization methods, RBAT is a sequential algorithm that is not scalable to large datasets. This limits the applicability of RBAT in practice. To address this issue, in this paper, we develop a parallel version of RBAT using MapReduce. We partition the data across cluster of computing nodes and implement the key operations of RBAT in parallel. Our experimental results show that scalable economization of large transaction datasets can be achieved using MapReduce and our method can scale nearly linear to the number of processing nodes.
更多查看译文
关键词
MapReduce,Distributed Algorithms,Parallel Processing,Parallel Method,Privacy,Anonymization,Generalization,Scalability,Top-Down Method
AI 理解论文
溯源树
样例
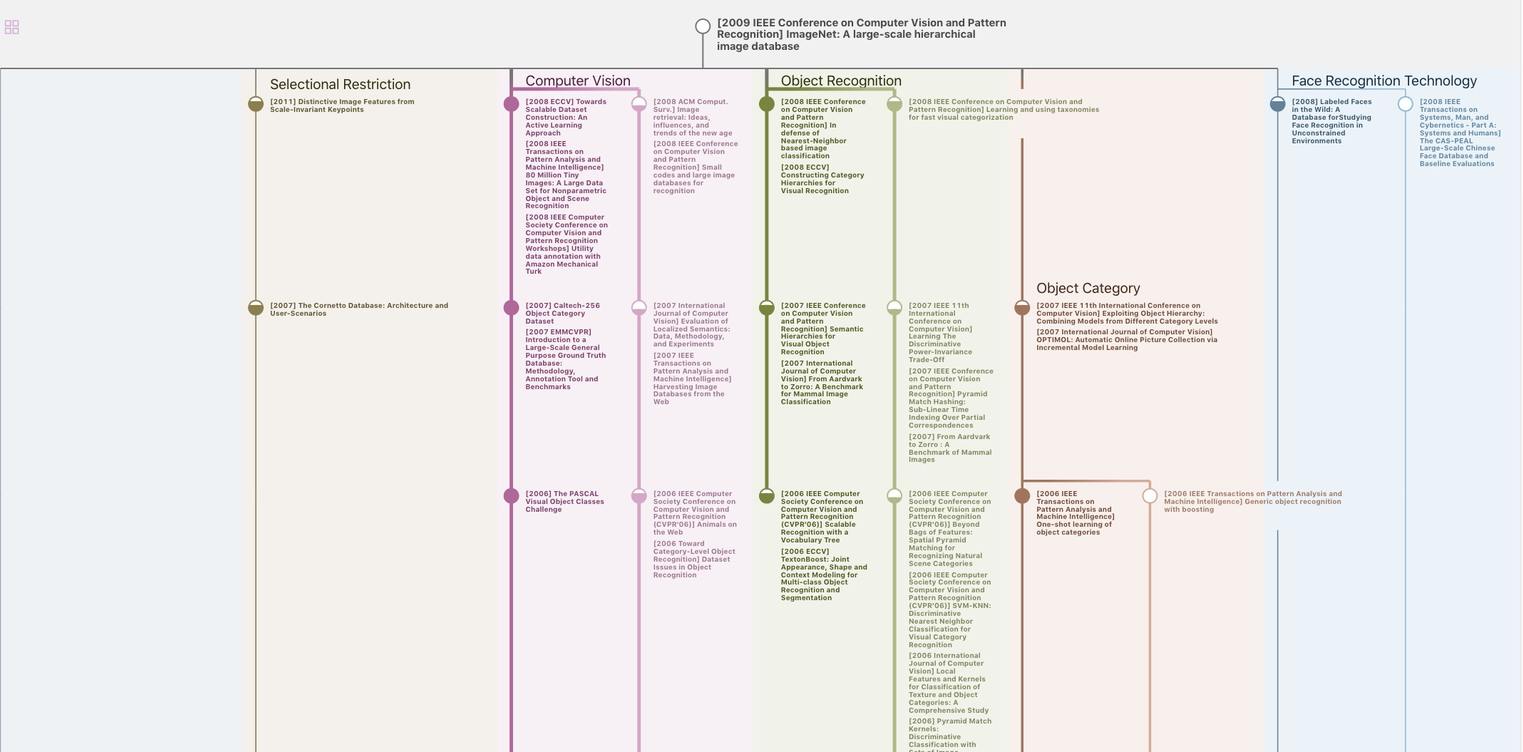
生成溯源树,研究论文发展脉络
Chat Paper
正在生成论文摘要