Markov Chain Sparsification With Independent Sets For Approximate Value Iteration
2015 53rd Annual Allerton Conference on Communication, Control, and Computing (Allerton)(2015)
摘要
The ever-increasing size of wireless networks poses a significant computational challenge for policy optimization schemes. In this paper, we propose a technique to reduce the dimensionality of the value iteration problem, and thereby reduce computational complexity, by exploiting certain structural properties of the logical state transition network. Specifically, our method involves approximating the original Markov chain by a simplified one whose state transition graph contains an independent set of a prespecified size, thus resulting in a sparsification of the transition probability matrix. As a result, value iteration needs to be performed only on the vertex cover of the network, from which the value function on the independent set can be obtained in a one-step process via interpolation. The Markov chain approximation process presented in this paper, for a given choice of independent set, involves minimizing matrix distance defined in terms of Frobenius norm or the Kullback-Leibler distance. This minimum distance then helps us to define a cost that can be minimized through an iterative greedy algorithm to obtain an approximately optimal independent set. Our method provides a tradeoff between accuracy and complexity that one can exploit by choosing the size of the independent set. Numerical results show that for a class of "collision" networks the value function approximation is accurate, even with a large independent set.
更多查看译文
关键词
Markov chain,independent set,Markov decision processes,approximate value function,wireless networks
AI 理解论文
溯源树
样例
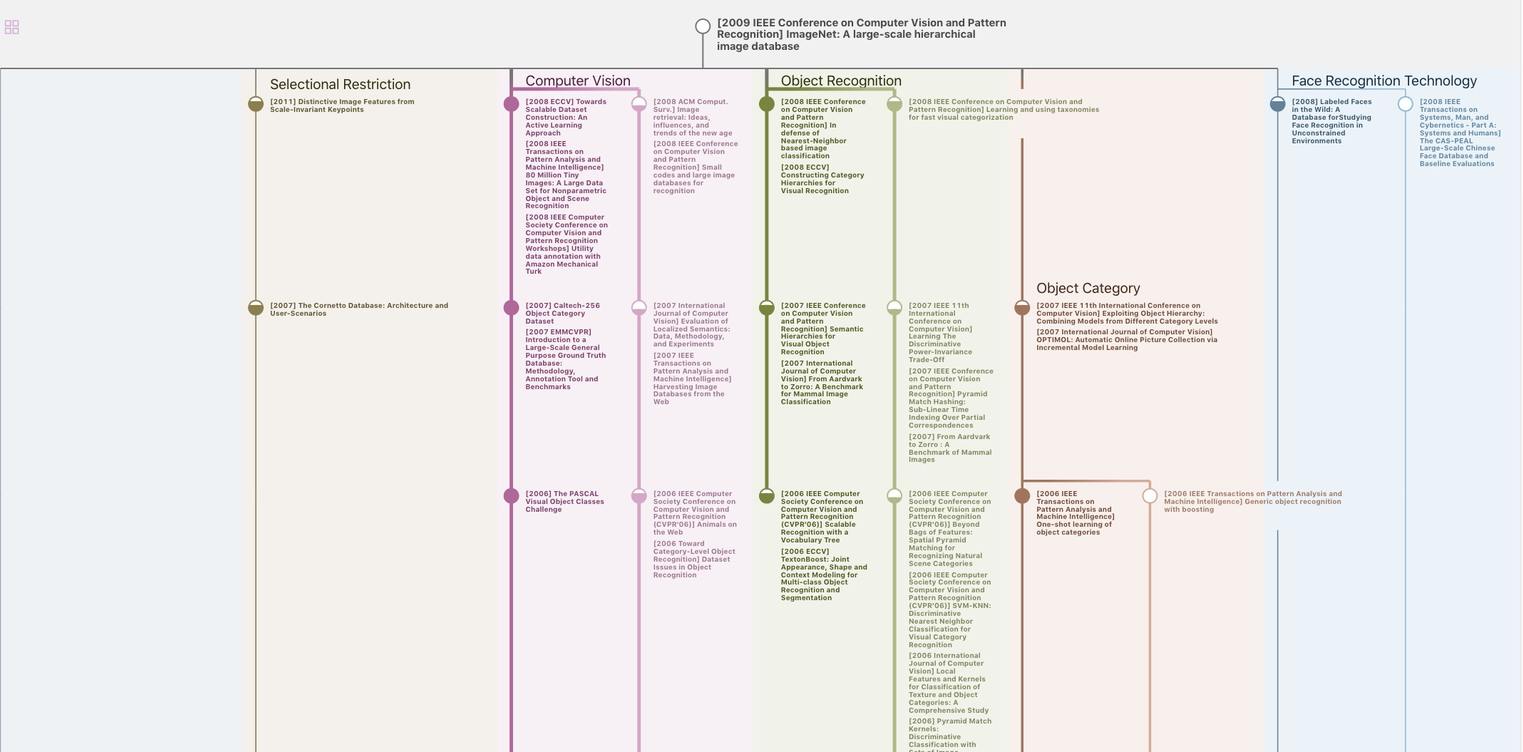
生成溯源树,研究论文发展脉络
Chat Paper
正在生成论文摘要