Adaqac: Adaptive Query Auto-Completion Via Implicit Negative Feedback
SIGIR '15: The 38th International ACM SIGIR conference on research and development in Information Retrieval Santiago Chile August, 2015(2015)
摘要
Query auto-completion (QAC) facilitates user query composition by suggesting queries given query prefix inputs. In 2014, global users of Yahoo! Search saved more than 50% keystrokes when submitting English queries by selecting suggestions of QAC.Users' preference of queries can be inferred during user-QAC interactions, such as dwelling on suggestion lists for a long time without selecting query suggestions ranked at the top. However, the wealth of such implicit negative feedback has not been exploited for designing QAC models. Most existing QAC models rank suggested queries for given prefixes based on certain relevance scores.We take the initiative towards studying implicit negative feedback during user-QAC interactions. This motivates re-designing QAC in the more general "(static) relevance-(adaptive) implicit negative feedback" framework. We propose a novel adaptive model adaQAC that adapts query auto-completion to users' implicit negative feedback towards unselected query suggestions. We collect user-QAC interaction data and perform large-scale experiments. Empirical results show that implicit negative feedback significantly and consistently boosts the accuracy of the investigated static QAC models that only rely on relevance scores. Our work compellingly makes a key point: QAC should be designed in a more general framework for adapting to implicit negative feedback.
更多查看译文
关键词
Query Auto-Completion,Implicit Negative Feedback
AI 理解论文
溯源树
样例
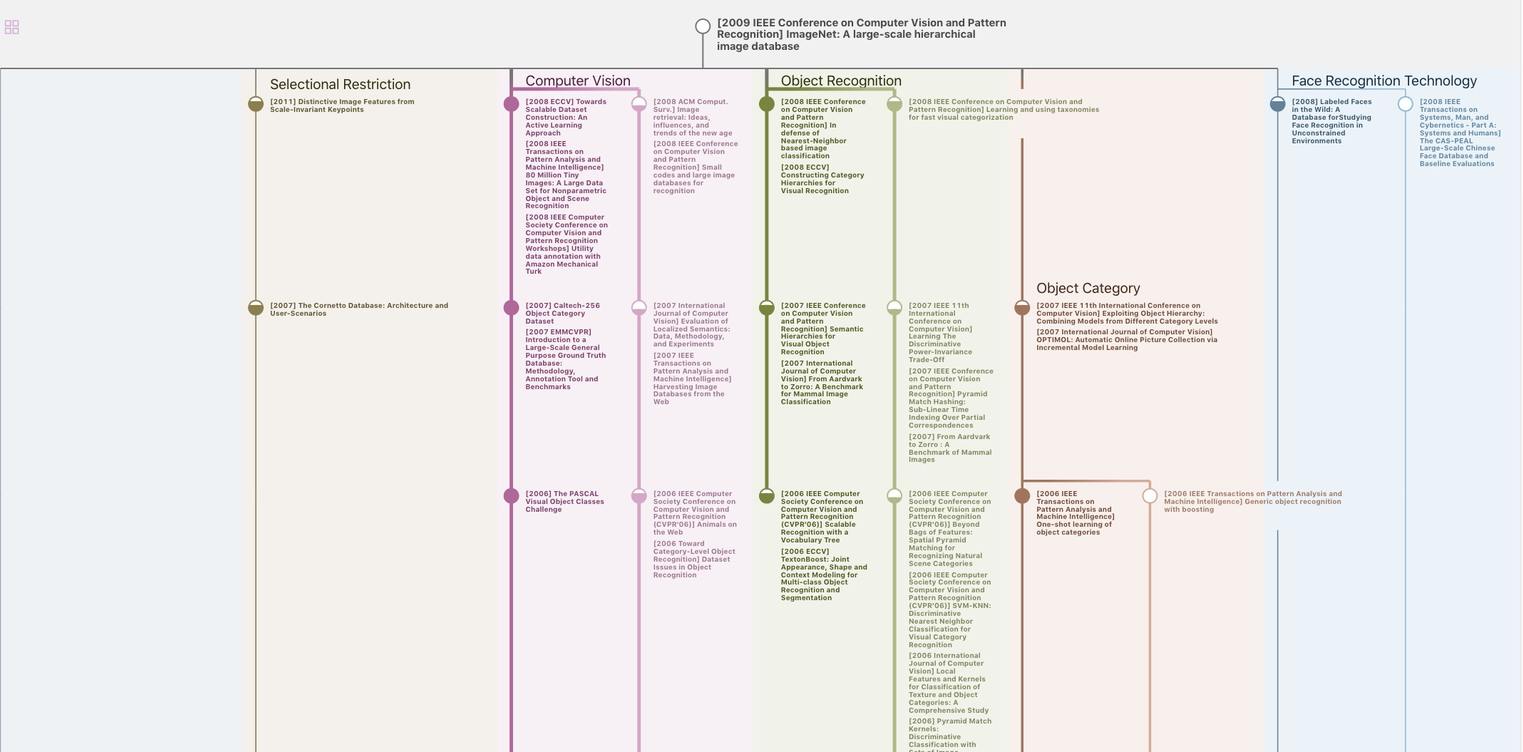
生成溯源树,研究论文发展脉络
Chat Paper
正在生成论文摘要