A non-intrusive model to predict the exible energy in a residential building
WCNC Workshops(2015)
摘要
The building energy consumption represent 60% of total primary energy consumption in the world. In order to control the demand response schemes for residential users, it is crucial to be able to predict the different components of the total power consumption of a household. This work provide a non intrusive identification model of devices with a sample frequency of one hertz. The identification results are the inputs of a model to predict the flexible energy. This corresponds at the different devices could be shift in a predetermined time. In a residential building, the heating and the hot water represent this flexible energy. The Support Vector Machine (SVM) enable an identification around 95% of heating, hot water, household electrical and a ensemble of decision tree provide the prediction for the next 15 minutes.
更多查看译文
关键词
Data intelligence analysis, Microgrid, Advanced Metering Infrastructure, Energy information management, KNIME
AI 理解论文
溯源树
样例
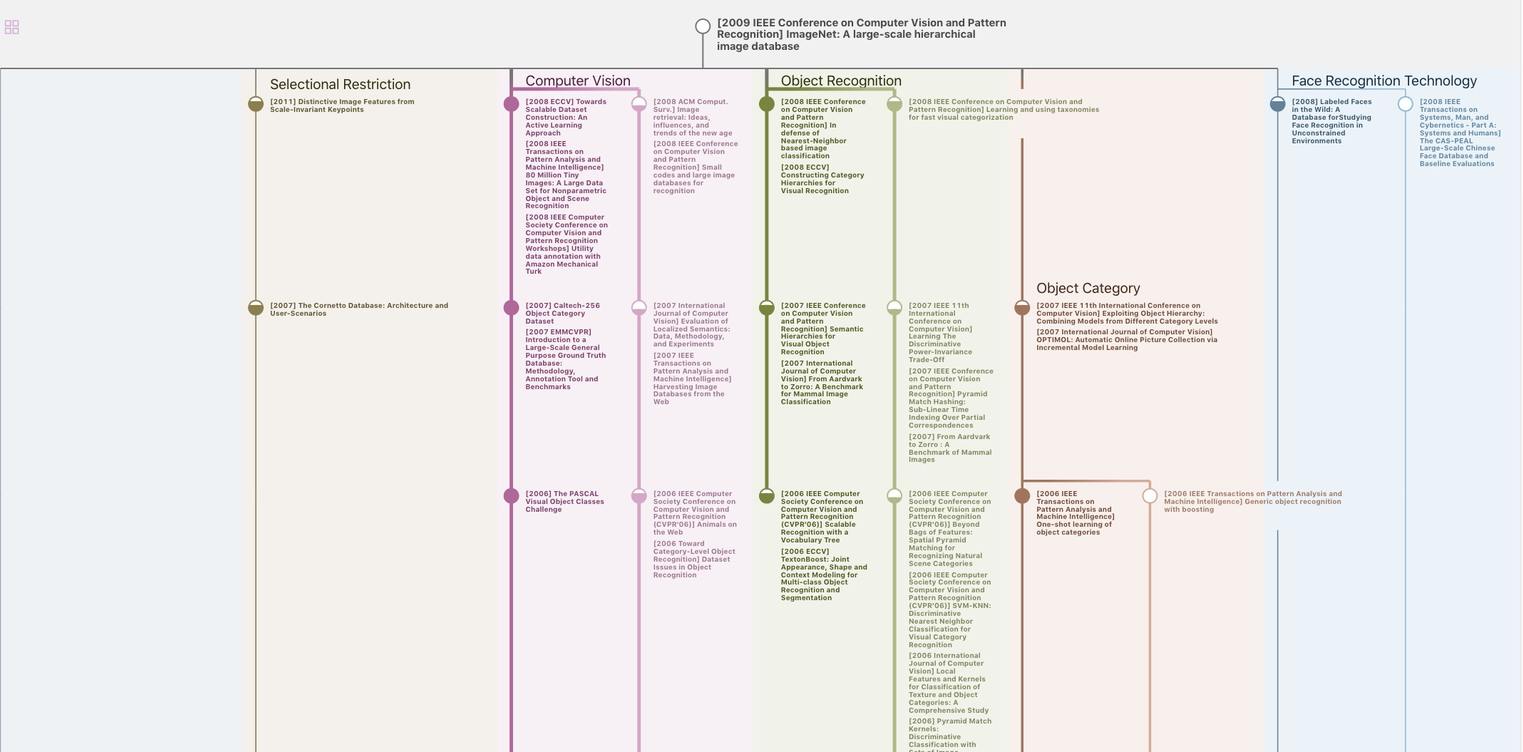
生成溯源树,研究论文发展脉络
Chat Paper
正在生成论文摘要