Feature selection using Joint Mutual Information Maximisation
Expert Systems with Applications(2015)
摘要
Two new feature selection methods are proposed based on joint mutual information.The methods use joint mutual information with maximum of the minimum criterion.The methods address the problem of selection of redundant and irrelevant features.The methods are evaluated using eleven public data sets and five competing methods.The proposed JMIM method outperforms five competing methods in terms of accuracy. Feature selection is used in many application areas relevant to expert and intelligent systems, such as data mining and machine learning, image processing, anomaly detection, bioinformatics and natural language processing. Feature selection based on information theory is a popular approach due its computational efficiency, scalability in terms of the dataset dimensionality, and independence from the classifier. Common drawbacks of this approach are the lack of information about the interaction between the features and the classifier, and the selection of redundant and irrelevant features. The latter is due to the limitations of the employed goal functions leading to overestimation of the feature significance.To address this problem, this article introduces two new nonlinear feature selection methods, namely Joint Mutual Information Maximisation (JMIM) and Normalised Joint Mutual Information Maximisation (NJMIM); both these methods use mutual information and the 'maximum of the minimum' criterion, which alleviates the problem of overestimation of the feature significance as demonstrated both theoretically and experimentally. The proposed methods are compared using eleven publically available datasets with five competing methods. The results demonstrate that the JMIM method outperforms the other methods on most tested public datasets, reducing the relative average classification error by almost 6% in comparison to the next best performing method. The statistical significance of the results is confirmed by the ANOVA test. Moreover, this method produces the best trade-off between accuracy and stability.
更多查看译文
关键词
Feature selection,Mutual information,Joint mutual information,Conditional mutual information,Subset feature selection,Classification,Dimensionality reduction,Feature selection stability
AI 理解论文
溯源树
样例
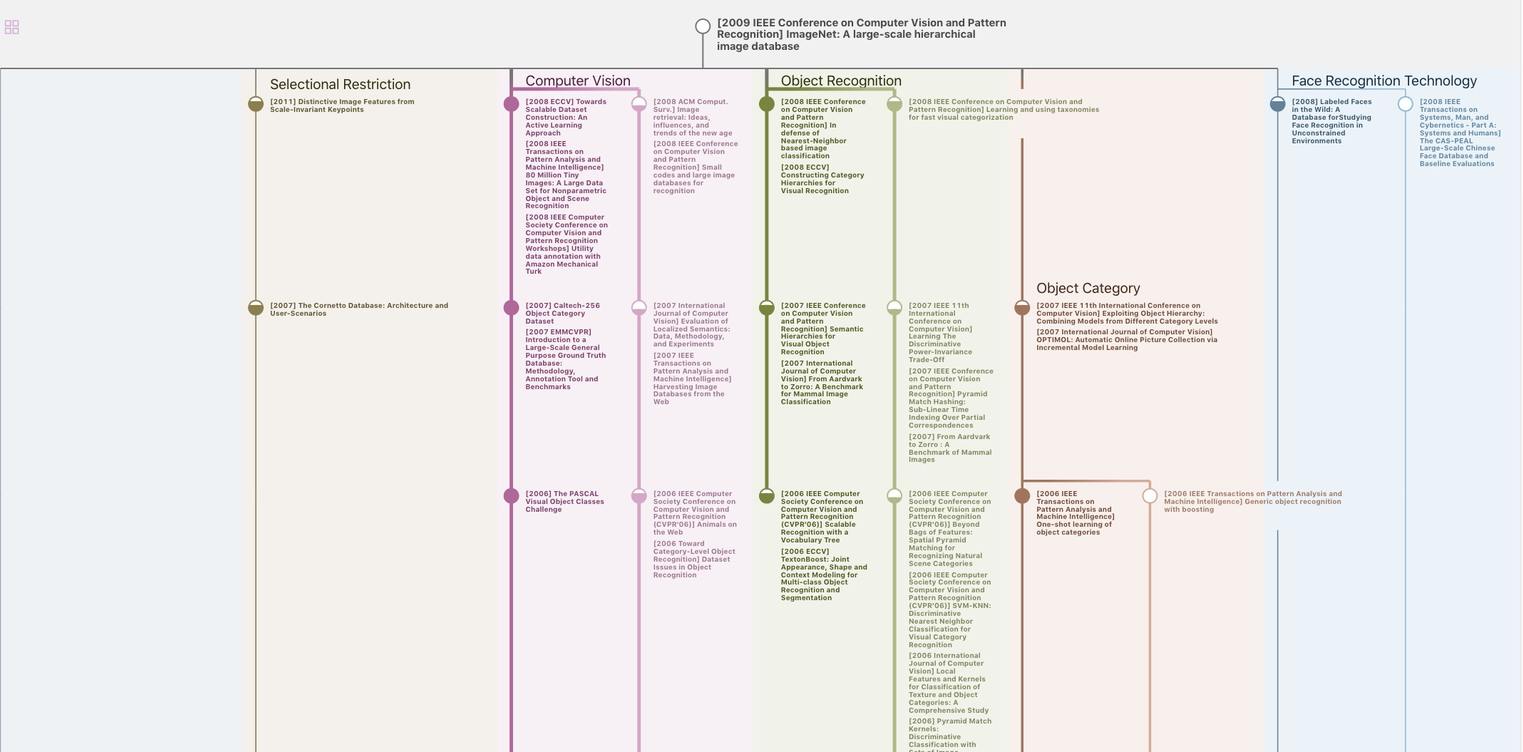
生成溯源树,研究论文发展脉络
Chat Paper
正在生成论文摘要