Discriminative transfer subspace learning via low-rank and sparse representation
IEEE Transactions on Image Processing(2016)
摘要
In this paper, we address the problem of unsupervised domain transfer learning in which no labels are available in the target domain. We use a transformation matrix to transfer both the source and target data to a common subspace, where each target sample can be represented by a combination of source samples such that the samples from different domains can be well interlaced. In this way, the discrepancy of the source and target domains is reduced. By imposing joint low-rank and sparse constraints on the reconstruction coefficient matrix, the global and local structures of data can be preserved. To enlarge the margins between different classes as much as possible and provide more freedom to diminish the discrepancy, a flexible linear classifier (projection) is obtained by learning a non-negative label relaxation matrix that allows the strict binary label matrix to relax into a slack variable matrix. Our method can avoid a potentially negative transfer by using a sparse matrix to model the noise and, thus, is more robust to different types of noise. We formulate our problem as a constrained low-rankness and sparsity minimization problem and solve it by the inexact augmented Lagrange multiplier method. Extensive experiments on various visual domain adaptation tasks show the superiority of the proposed method over the state-of-the art methods. The MATLAB code of our method will be publicly available at http://www.yongxu.org/lunwen.html. © 2015 IEEE.
更多查看译文
关键词
Source domain, target domain, low-rank and sparse constraints, knowledge transfer, subspace learning
AI 理解论文
溯源树
样例
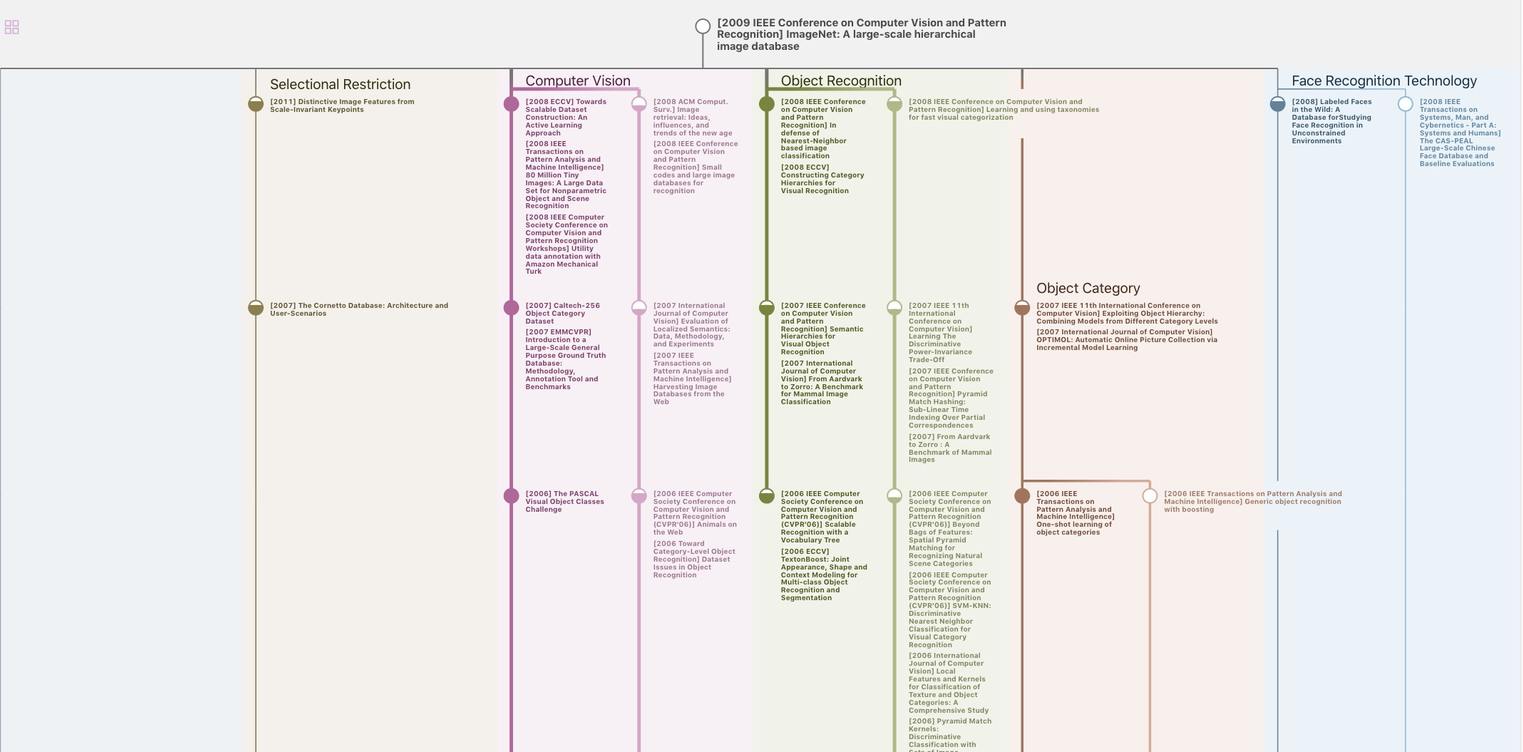
生成溯源树,研究论文发展脉络
Chat Paper
正在生成论文摘要