Practical data prediction for real-world wireless sensor networks
IEEE Transactions on Knowledge and Data Engineering(2015)
摘要
Data prediction is proposed in wireless sensor networks (WSNs) to extend the system lifetime by enabling the sink to determine the data sampled, within some accuracy bounds, with only minimal communication from source nodes. Several theoretical studies clearly demonstrate the tremendous potential of this approach, able to suppress the vast majority of data reports at the source nodes. Nevertheless, the techniques employed are relatively complex, and their feasibility on resource-scarce WSN devices is often not ascertained. More generally, the literature lacks reports from real-world deployments, quantifying the overall system-wide lifetime improvements determined by the interplay of data prediction with the underlying network. These two aspects, feasibility and system-wide gains, are key in determining the practical usefulness of data prediction in real-world WSN applications. In this paper, we describe Derivative-Based Prediction (DBP), a novel data prediction technique much simpler than those found in the literature. Evaluation with real data sets from diverse WSN deployments shows that DBP often performs better than the competition, with data suppression rates up to 99% and good prediction accuracy. However, experiments with a real WSN in a road tunnel show that, when the network stack is taken into consideration, DBP only triples lifetime—a remarkable result per se, but a far cry from the data suppression rates above. To fully achieve the energy savings enabled by data prediction, the data and network layers must be jointly optimized. In our testbed experiments, a simple tuning of the MAC and routing stack, taking into account the operation of DBP, yields a remarkable seven-fold lifetime improvement w.r.t. the mainstream periodic reporting.
更多查看译文
关键词
Wireless sensor networks, data prediction, time series forecasting, energy efficiency, network protocols
AI 理解论文
溯源树
样例
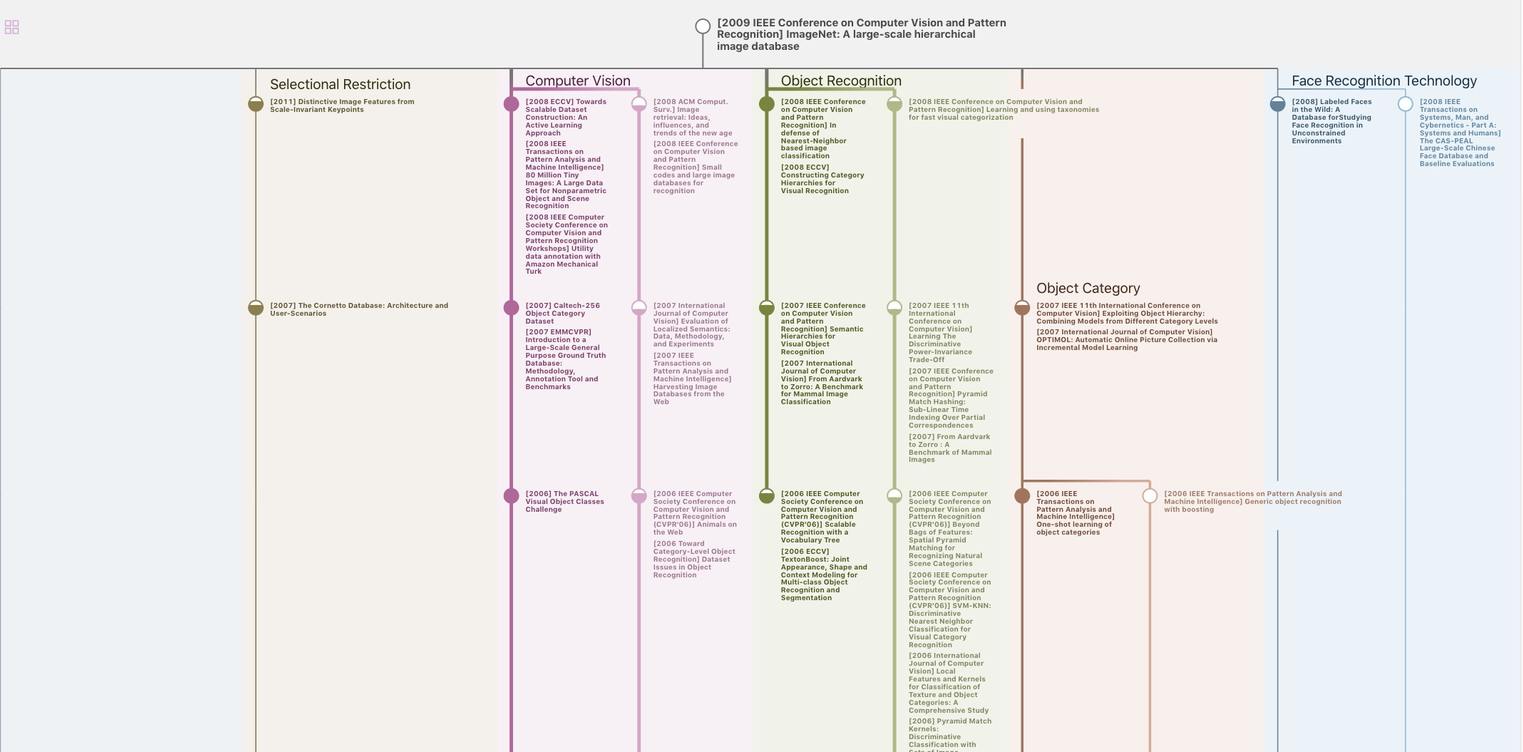
生成溯源树,研究论文发展脉络
Chat Paper
正在生成论文摘要