GPU acceleration of the stochastic grid bundling method for early-exercise options
International Journal of Computer Mathematics(2015)
摘要
In this work, a parallel graphics processing units GPU version of the Monte Carlo stochastic grid bundling method SGBM for pricing multi-dimensional early-exercise options is presented. To extend the method's applicability, the problem dimensions and the number of bundles will be increased drastically. This makes SGBM very expensive in terms of computational costs on conventional hardware systems based on central processing units. A parallelization strategy of the method is developed and the general purpose computing on graphics processing units paradigm is used to reduce the execution time. An improved technique for bundling asset paths, which is more efficient on parallel hardware is introduced. Thanks to the performance of the GPU version of SGBM, a general approach for computing the early-exercise policy is proposed. Comparisons between sequential and GPU parallel versions are presented.
更多查看译文
关键词
computational finance, early-exercise derivatives, basket Bermudan options, high-dimensional pricing, stochastic grid bundling method (SGBM), Monte Carlosimulation, least-squares regression, high performance computing, parallel programming, GPGPU, compute unified device architecture (CUDA)
AI 理解论文
溯源树
样例
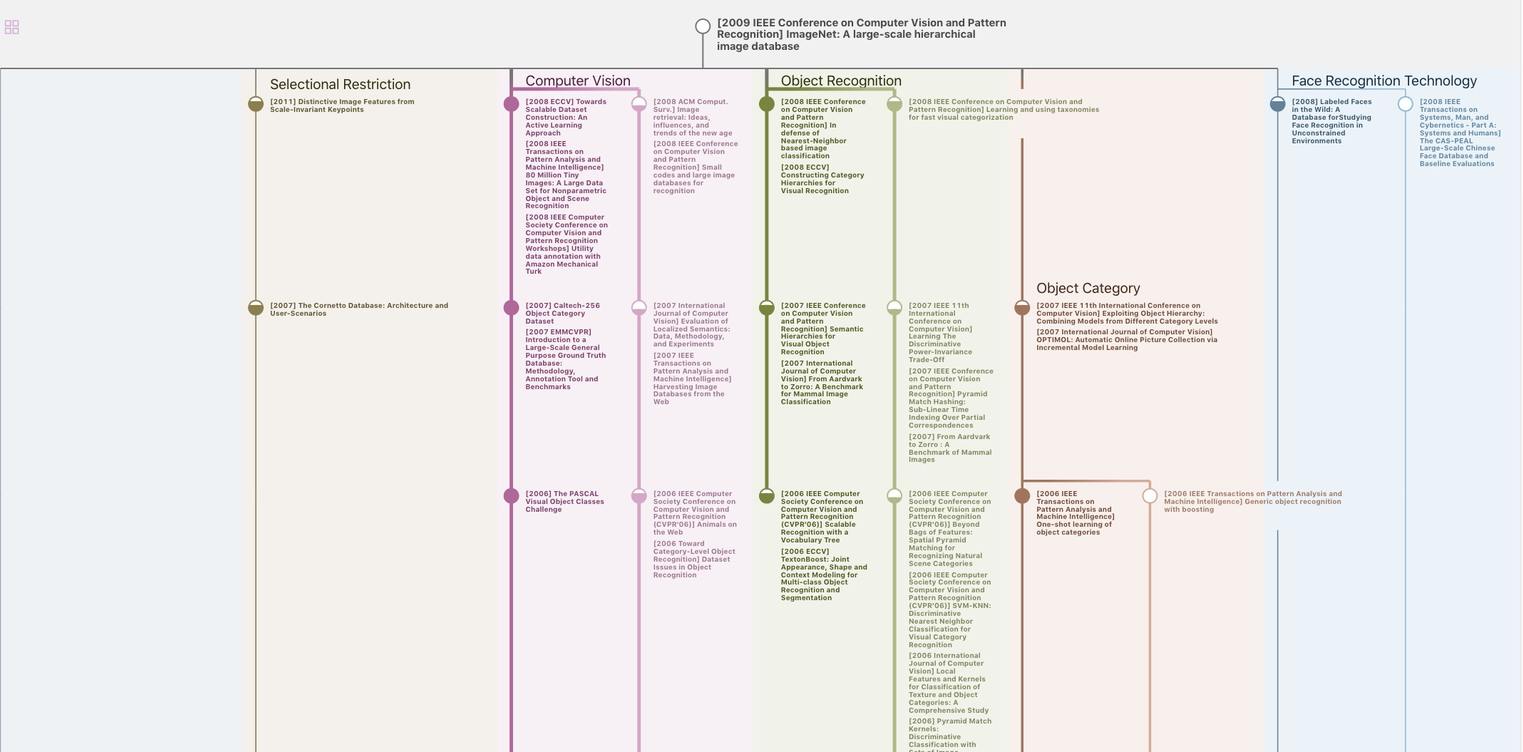
生成溯源树,研究论文发展脉络
Chat Paper
正在生成论文摘要