A Convex Approach For Image Restoration With Exact Poisson-Gaussian Likelihood
Siam Journal on Imaging Sciences(2015)
摘要
The Poisson-Gaussian model can accurately describe the noise present in a number of imaging systems. However most existing restoration methods rely on approximations of the Poisson-Gaussian noise statistics. We propose a convex optimization strategy for the reconstruction of images degraded by a linear operator and corrupted with a mixed Poisson-Gaussian noise. The originality of our approach consists of considering the exact, mixed continuous-discrete model corresponding to the data statistics. After establishing the Lipschitz differentiability and convexity of the Poisson-Gaussian neg-log-likelihood, we derive a primal-dual iterative scheme for minimizing the associated penalized criterion. The proposed method is applicable to a large choice of convex penalty terms. The robustness of our scheme allows us to handle computational difficulties due to infinite sums arising from the computation of the gradient of the criterion. We propose finite bounds for these sums, that are dependent on the current image estimate, and thus adapted to each iteration of our algorithm. The proposed approach is validated on image restoration examples. Then, the exact data fidelity term is used as a reference for studying some of its various approximations. We show that in a variational framework the shifted Poisson and exponential approximations lead to very good restoration results.
更多查看译文
关键词
Poisson-Gaussian noise,convex optimization,proximal methods,primal-dual algorithm,image restoration
AI 理解论文
溯源树
样例
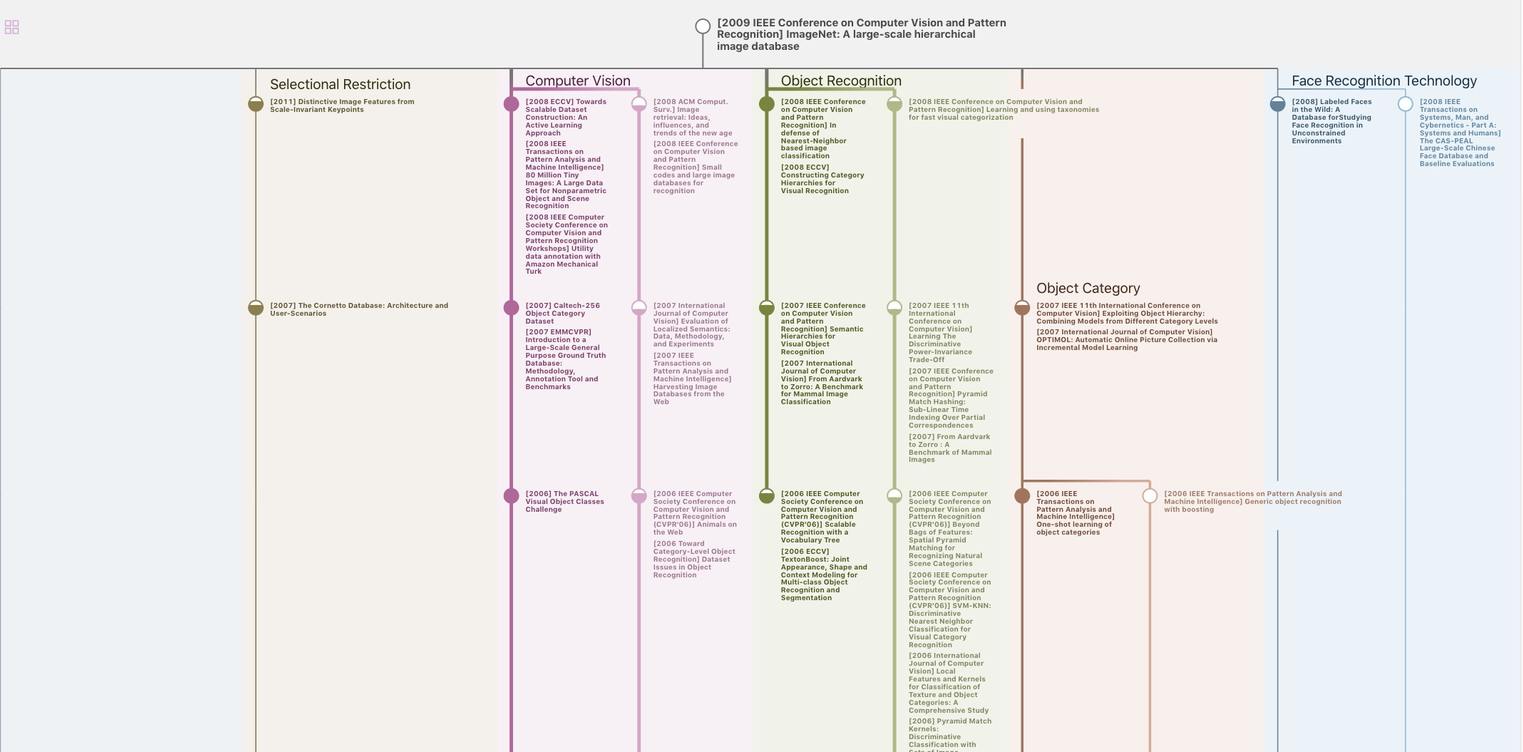
生成溯源树,研究论文发展脉络
Chat Paper
正在生成论文摘要