Scalable MCMC for Mixed Membership Stochastic Blockmodels
JMLR Workshop and Conference Proceedings(2016)
摘要
We propose a stochastic gradient Markov chain Monte Carlo (SG-MCMC) algorithm for scalable inference in mixed-membership stochastic blockmodels (MMSB). Our algorithm is based on the stochastic gradient Riemannian Langevin sampler and achieves both faster speed and higher accuracy at every iteration than the current state-of-the-art algorithm based on stochastic variational inference. In addition we develop an approximation that can handle models that entertain a very large number of communities. The experimental results show that SG-MCMC strictly dominates competing algorithms in all cases.
更多查看译文
关键词
scalable mcmc,membership
AI 理解论文
溯源树
样例
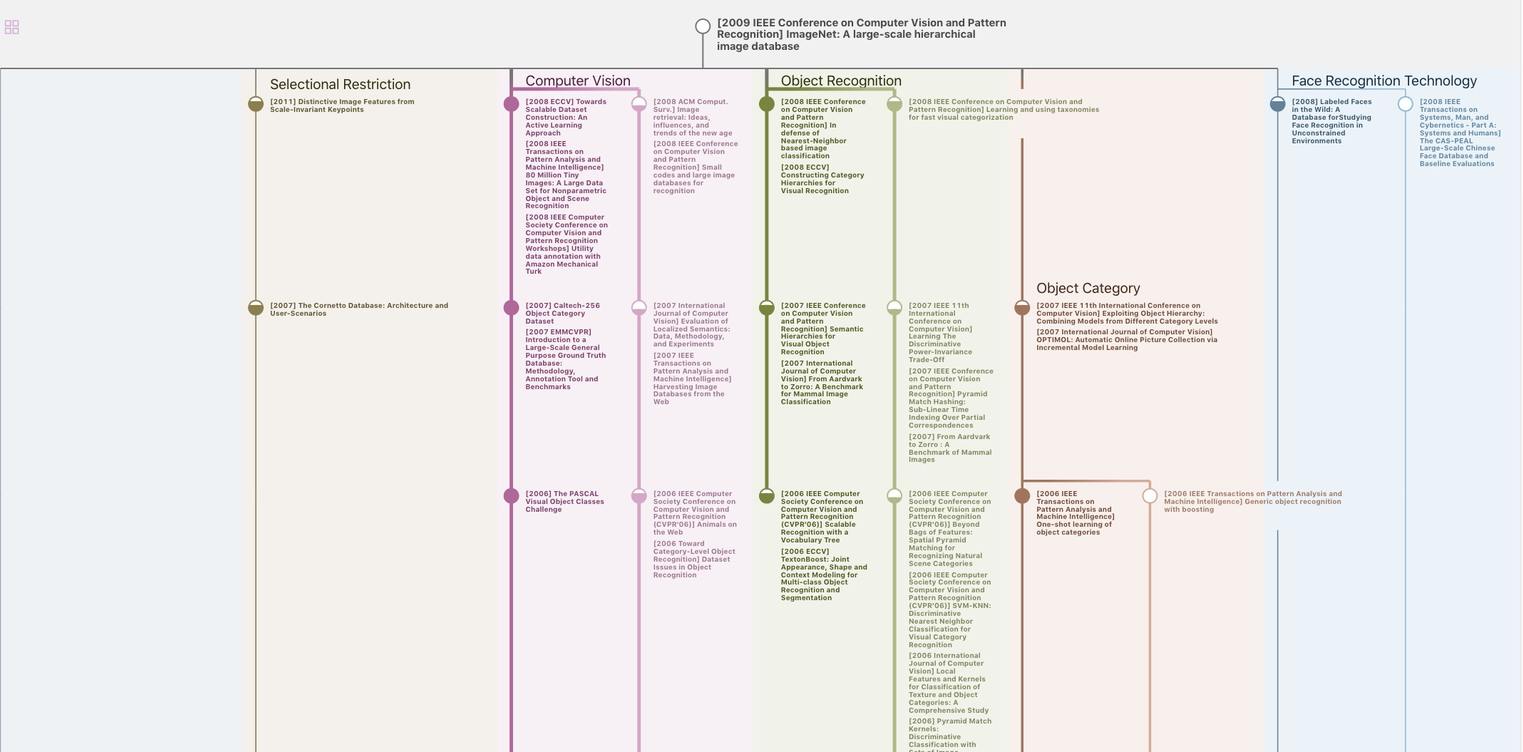
生成溯源树,研究论文发展脉络
Chat Paper
正在生成论文摘要