When two choices are not enough: Balancing at scale in Distributed Stream Processing
2016 IEEE 32nd International Conference on Data Engineering (ICDE)(2016)
摘要
Carefully balancing load in distributed stream processing systems has a fundamental impact on execution latency and throughput. Load balancing is challenging because real-world workloads are skewed: some tuples in the stream are associated to keys which are significantly more frequent than others. Skew is remarkably more problematic in large deployments: having more workers implies fewer keys per worker, so it becomes harder to “average out” the cost of hot keys with cold keys. We propose a novel load balancing technique that uses a heavy hitter algorithm to efficiently identify the hottest keys in the stream. These hot keys are assigned to d ≥ 2 choices to ensure a balanced load, where d is tuned automatically to minimize the memory and computation cost of operator replication. The technique works online and does not require the use of routing tables. Our extensive evaluation shows that our technique can balance real-world workloads on large deployments, and improve throughput and latency by 150% and 60% respectively over the previous state-of-the-art when deployed on Apache Storm.
更多查看译文
关键词
distributed stream processing,load balancing technique,heavy hitter algorithm,hot keys,operator replication,Apache Storm
AI 理解论文
溯源树
样例
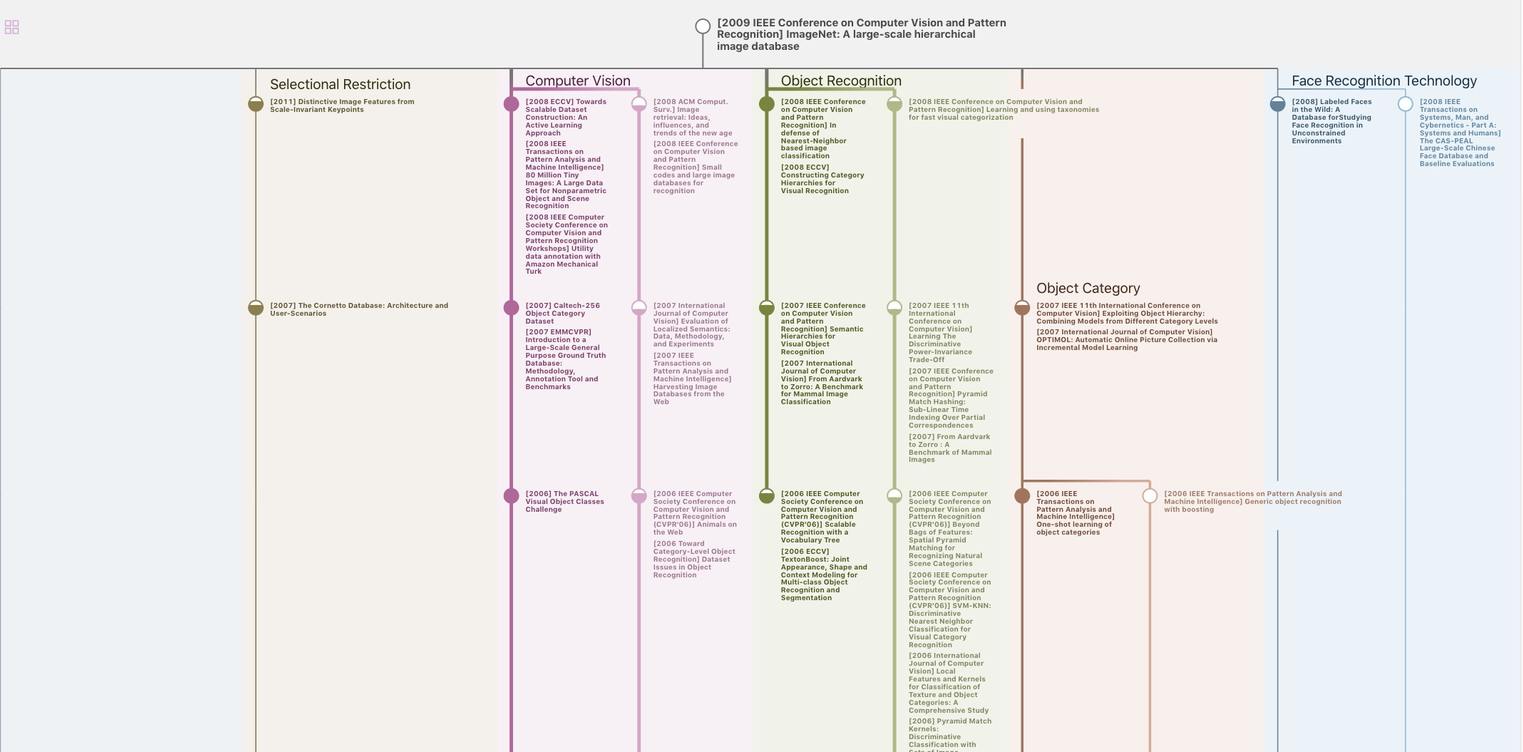
生成溯源树,研究论文发展脉络
Chat Paper
正在生成论文摘要