Exploiting matrix factorization to asymmetric user similarities in recommendation systems
Knowledge-Based Systems(2015)
摘要
lthough collaborative filtering is widely applied in recommendation systems, it still suffers from several major limitations, including data sparsity and scalability. Sparse data affects the quality of the user similarity measurement and consequently the quality of the recommender system. In this paper, we propose a novel user similarity measure aimed at providing a valid similarity measurement between users with very few ratings. The contributions of this paper are twofold: First, we suggest an asymmetric user similarity method to distinguish between the impact that the user has on his neighbor and the impact that the user receives from his neighbor. Second, we apply matrix factorization to the user similarity matrix in order to discover the similarities between users who have rated different items. Experimental results show that our method performs better than commonly used approaches, especially under cold-start condition.
更多查看译文
关键词
Collaborative filtering,Matrix factorization,Recommender systems,User similarity,Asymmetry
AI 理解论文
溯源树
样例
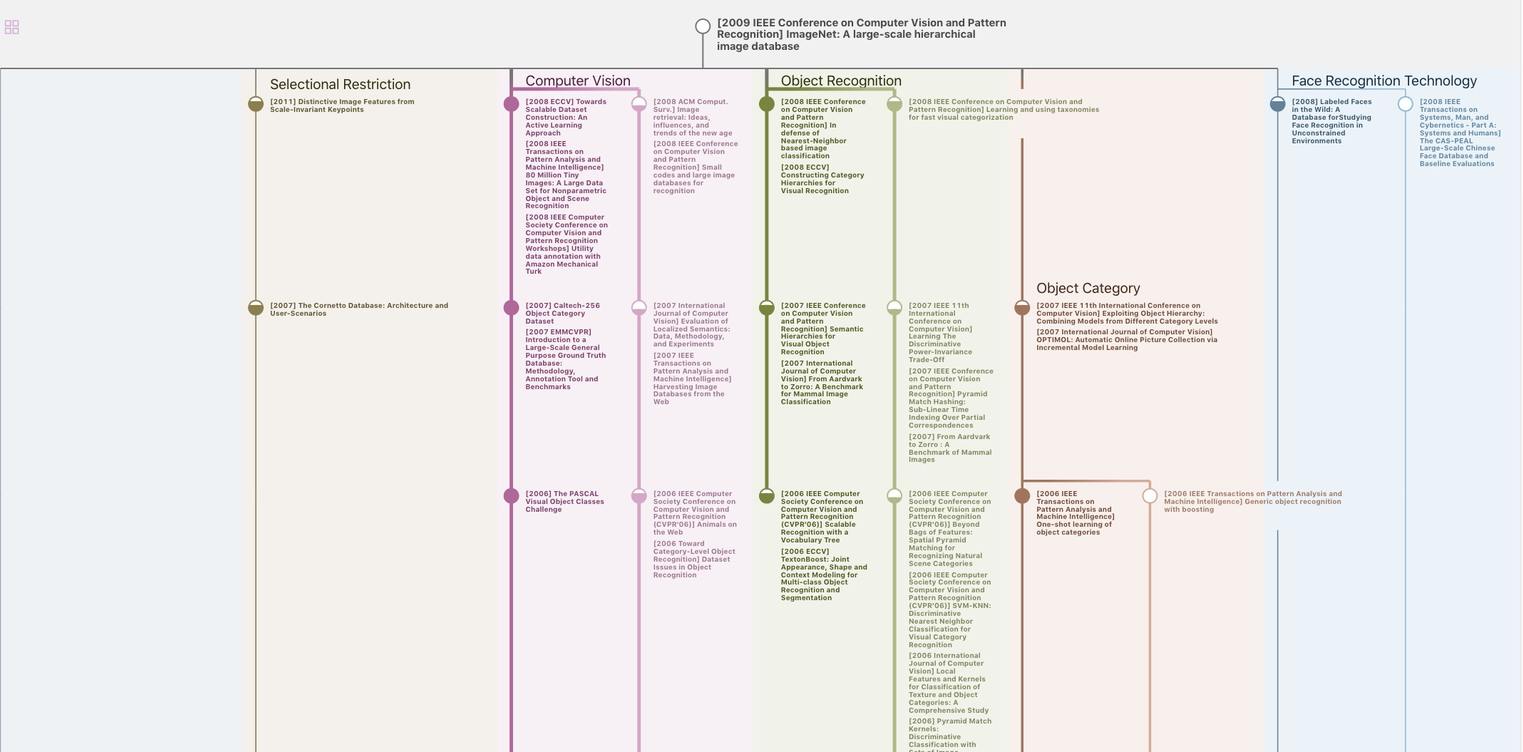
生成溯源树,研究论文发展脉络
Chat Paper
正在生成论文摘要