Joint modeling and reconstruction of a compressively-sensed set of correlated images
Journal of Visual Communication and Image Representation(2015)
摘要
We model a set of images by jointly considering different types of correlations.The joint modeling-based minimization problem is solved efficiently.The proposed algorithm is extended for reconstruction of color image sets.We explain how and why the parameters are selected in our experiments.Our algorithms outperform many state-of-the-arts CS reconstruction methods. Employing correlation among images for improved reconstruction in compressive sensing is a conceptually attractive idea, although developing efficient modeling strategies and reconstruction algorithms are often the key to achieve any potential benefit. This paper presents a novel modeling strategy and an efficient reconstruction algorithm for processing a set of correlated images, jointly taking into consideration inter-image correlation, intra-image correlation and inter-channel correlation. The approach starts with joint modeling of the entire image set in the gradient domain, which supports simultaneous representation of local smoothness, nonlocal self-similarity of every single image, and inter-image correlation. Then an efficient algorithm is proposed to solve the joint formulation, using a Split-Bregman-based technique. Furthermore, to support color image reconstruction, the proposed algorithm is extended by using the concept of group sparsity to explore inter-channel correlation. The effectiveness of the proposed approach is demonstrated with extensive experiments on both grayscale and color image sets. Results are also compared with recently proposed compressive sensing recovery algorithms.
更多查看译文
关键词
Compressive sensing,Correlated images,Intra-image correlation,Inter-image correlation,Inter-channel correlation,Total variation,Non-local means,Group sparsity
AI 理解论文
溯源树
样例
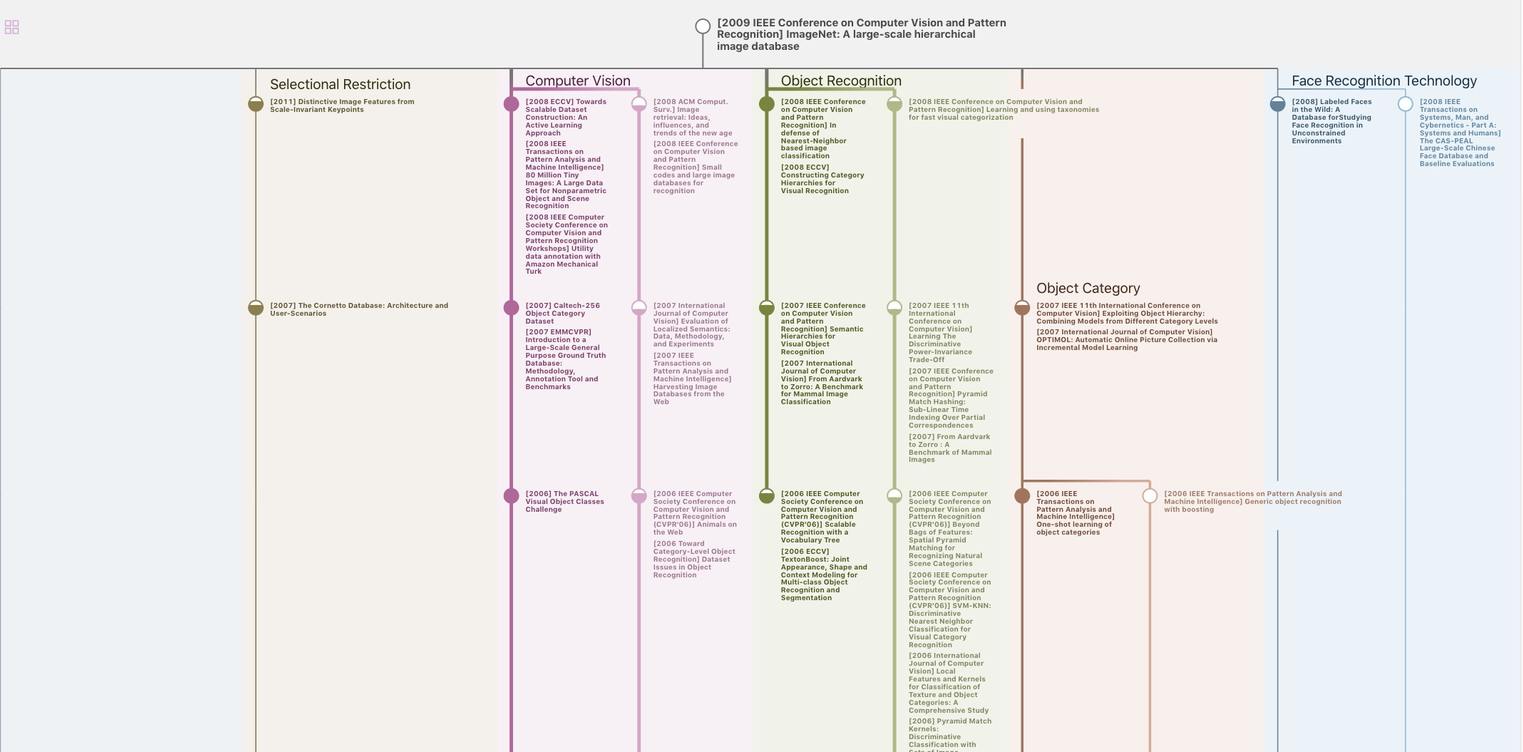
生成溯源树,研究论文发展脉络
Chat Paper
正在生成论文摘要