A Deterministic Analysis for LRR
IEEE Transactions on Pattern Analysis and Machine Intelligence(2016)
摘要
The recently proposed low-rank representation (LRR) method has been empirically shown to be useful in various tasks such as motion segmentation, image segmentation, saliency detection and face recognition. While potentially powerful, LRR depends heavily on the configuration of its key parameter, $lambda$ . In realistic environments where the prior knowledge about data is lacking, however, it is still unknown how to choose $lambda$ in a suitable way. Even more, there is a lack of rigorous analysis about the success conditions of the method, and thus the significance of LRR is a little bit vague. In this paper we therefore establish a theoretical analysis for LRR, striving for figuring out under which conditions LRR can be successful, and deriving a moderately good estimate to the key parameter $lambda$ as well. Simulations on synthetic data points and experiments on real motion sequences verify our claims.
更多查看译文
关键词
face recognition,image motion analysis,image representation,image segmentation,image sequences,matrix algebra,parameter estimation,pattern clustering,LRR method,deterministic analysis,face recognition,image segmentation,low-rank representation method,motion segmentation,outlier detection,parameter estimation,real motion sequences,saliency detection,subspace clustering,synthetic data points,Low-rank representation,low-rank representation,outlier detection,parameter estimation,subspace clustering
AI 理解论文
溯源树
样例
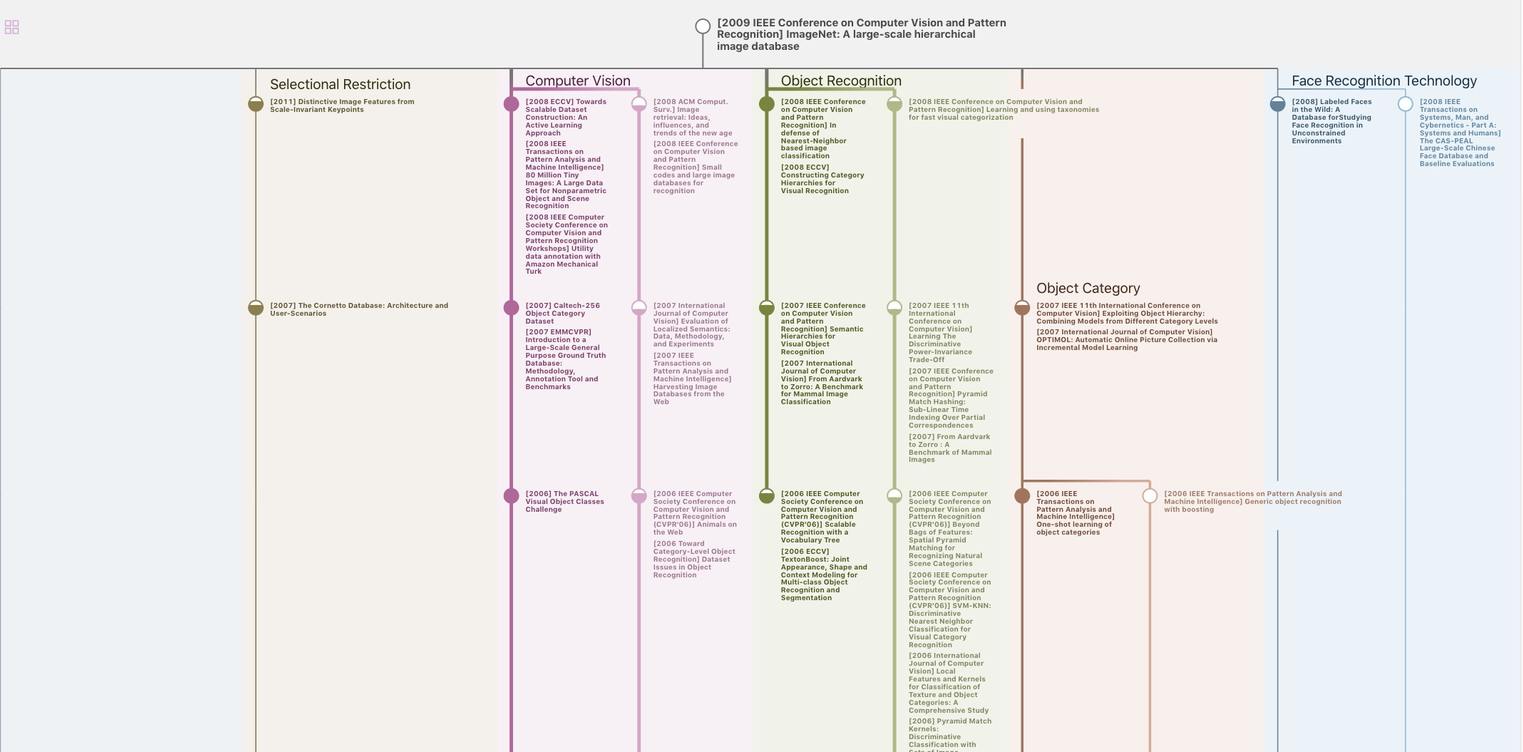
生成溯源树,研究论文发展脉络
Chat Paper
正在生成论文摘要