Multivariate Jackson-type inequality for a new type neural network approximation
Applied Mathematical Modelling(2014)
摘要
In this paper, we introduce a new type neural networks by superpositions of a sigmoidal function and study its approximation capability. We investigate the multivariate quantitative constructive approximation of real continuous multivariate functions on a cube by such type neural networks. This approximation is derived by establishing multivariate Jackson-type inequalities involving the multivariate modulus of smoothness of the target function. Our networks require no training in the traditional sense.
更多查看译文
关键词
Neural networks,Jackson-type inequality,Error estimate,Sigmoidal function
AI 理解论文
溯源树
样例
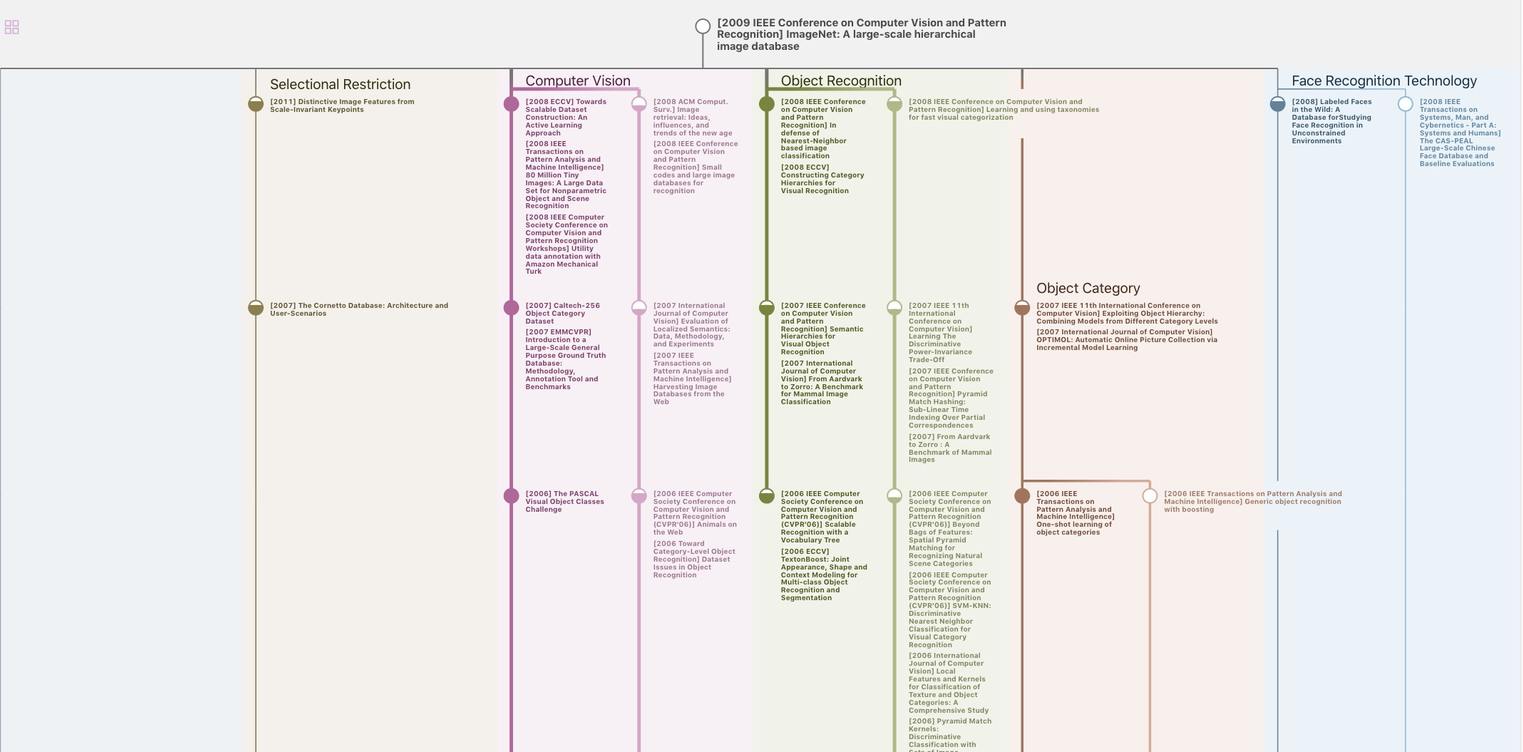
生成溯源树,研究论文发展脉络
Chat Paper
正在生成论文摘要