Probabilistic knowledge representation using the principle of maximum entropy and Gröbner basis theory
Ann. Math. Artif. Intell.(2015)
摘要
An often used methodology for reasoning with probabilistic conditional knowledge bases is provided by the principle of maximum entropy (so-called MaxEnt principle) that realises an idea of least amount of assumed information and thus of being as unbiased as possible. In this paper we exploit the fact that MaxEnt distributions can be computed by solving nonlinear equation systems that reflect the conditional logical structure of these distributions. We apply the theory of Gröbner bases that is well known from computational algebra to the polynomial system which is associated with a MaxEnt distribution, in order to obtain results for reasoning with maximum entropy. We develop a three-phase compilation scheme extracting from a knowledge base consisting of probabilistic conditionals the information which is crucial for MaxEnt reasoning and transforming it to a Gröbner basis. Based on this transformation, a necessary condition for knowledge bases to be consistent is derived. Furthermore, approaches to answering MaxEnt queries are presented by demonstrating how inferring the MaxEnt probability of a single conditional from a given knowledge base is possible. Finally, we discuss computational methods to establish general MaxEnt inference rules.
更多查看译文
关键词
Probabilistic knowledge representation,Principle of maximum entropy,Computer algebra,Gröbner basis,03B42
AI 理解论文
溯源树
样例
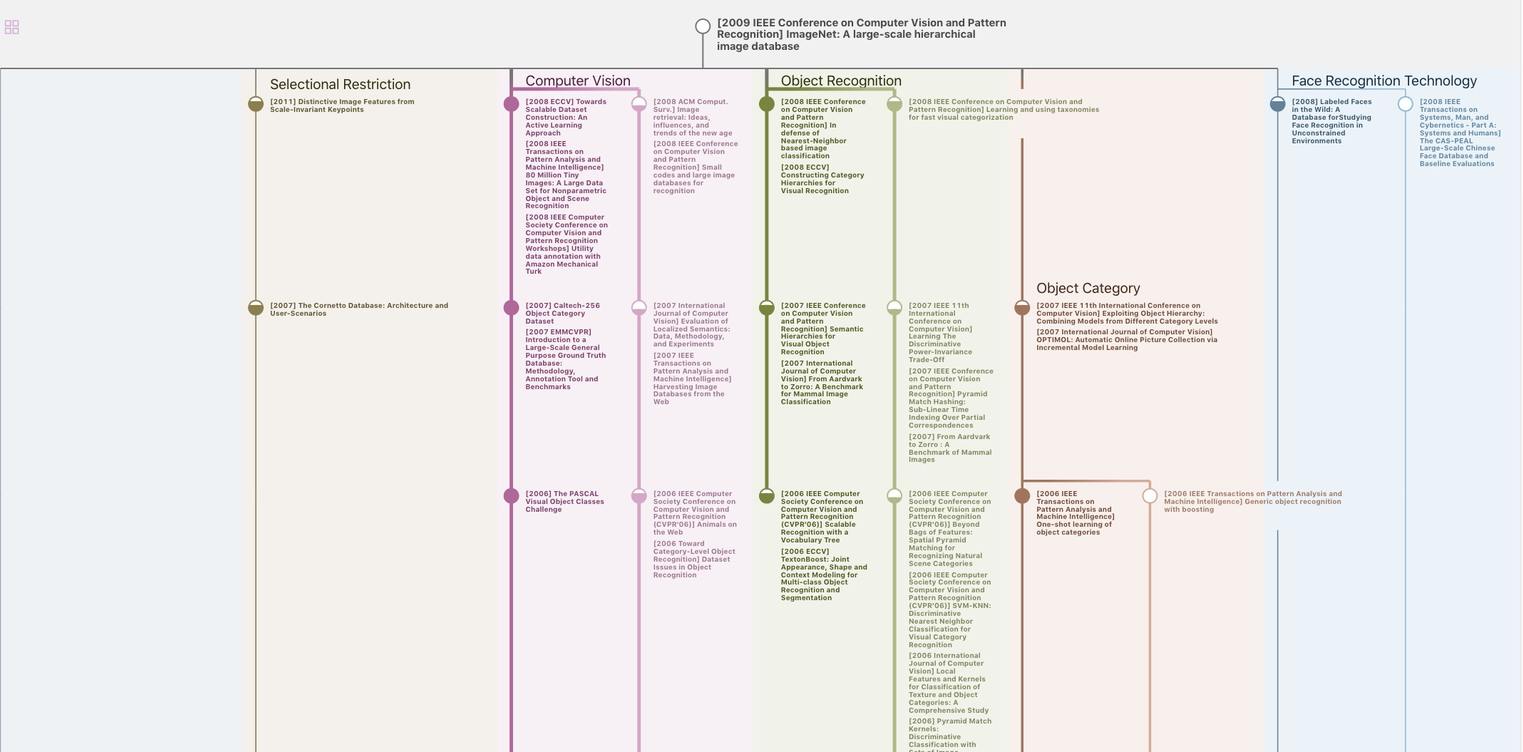
生成溯源树,研究论文发展脉络
Chat Paper
正在生成论文摘要