Action recognition by learning temporal slowness invariant features
The Visual Computer: International Journal of Computer Graphics(2015)
摘要
Deep learning approaches emphasized on learning spatio-temporal features for action recognition. Different to previous works, we separate the spatio-temporal feature learning unity into the spatial feature learning and the spatial/temporal feature pooling procedures. Using the temporal slowness regularized independent subspace analysis network, we learn invariant spatial features from sampled video cubes. To be robust to the cluttered backgrounds, we incorporate the denoising criterion to our network. The local spatio-temporal features are obtained by pooling features from the spatial and the temporal aspects. The key points are that we learn spatial features from video cubes and pool features from spatial feature sequences. We evaluate the learned local spatio-temporal features on three benchmark action datasets. Extensive experiments demonstrate the effectiveness of the novel feature learning architecture.
更多查看译文
关键词
Action recognition, Temporal slowness regularization, Spatio-temporal features, Independent subspace analysis, Support vector machine
AI 理解论文
溯源树
样例
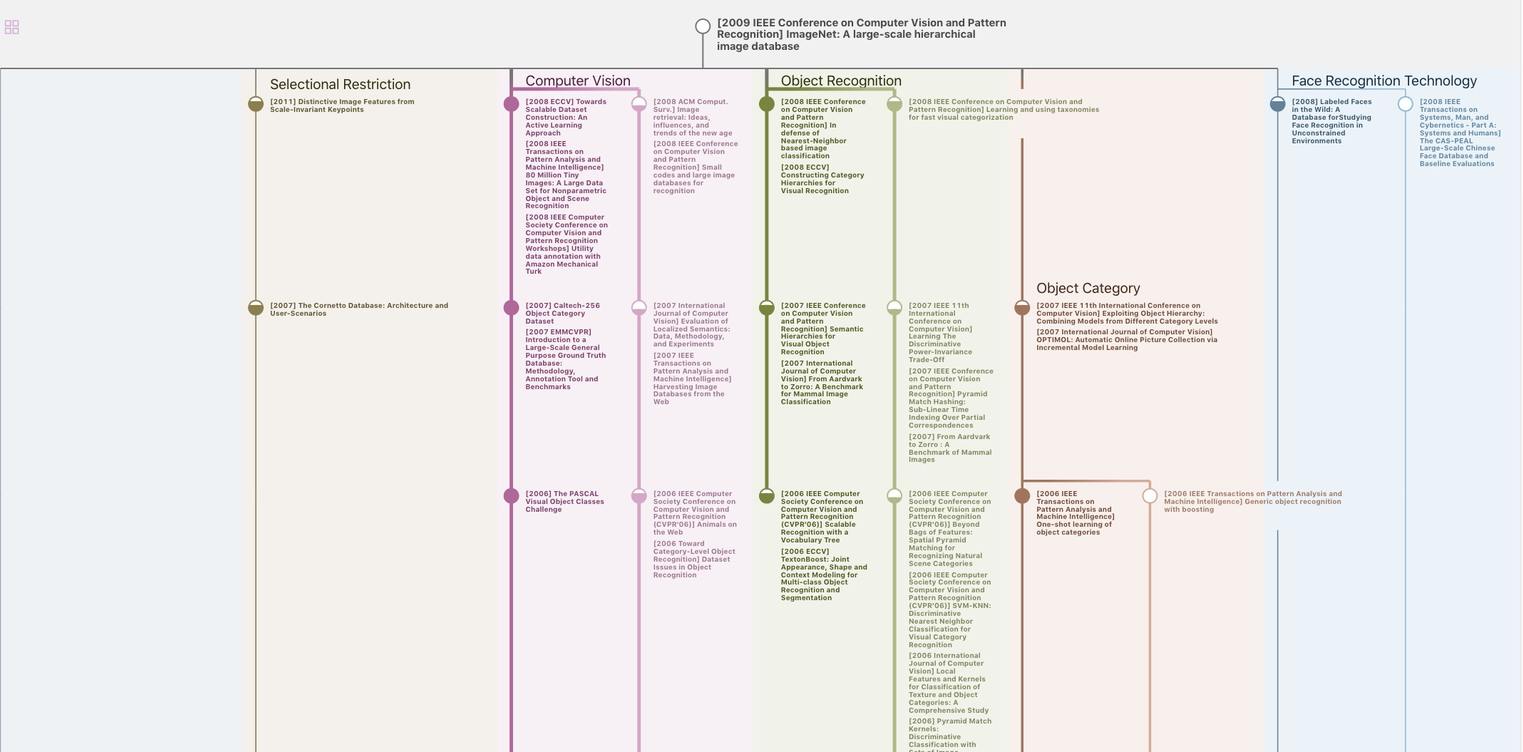
生成溯源树,研究论文发展脉络
Chat Paper
正在生成论文摘要