Unconstrained face verification by optimally organizing multiple classifiers
International Journal of Control Automation and Systems(2014)
摘要
Face verification is still a difficult problem in badly uncontrolled conditions. Motivated by the observation that failure of facial image pair-matching is always caused by inconsistent visual conditions of both total face and face parts, we propose to train multiple classifiers on total face and face parts with respect to different visual consistency conditions. For training these classifiers, multiple similarity measures of multiple features on the matched and mismatched face image pairs are considered as the positive and negative training samples respectively. For a new face image pair, we compute its similarity measures and visual consistency measures (VCM). Relying on these VCMs, we adaptively select VCM-specific classifiers and optimally organize them to form an ensemble by the reliability estimation and adaptively weighting combination. Our contributions are the visual consistency measure and the optimally organized VCM-specific classifier ensemble which can better overcome the influence of uncontrolled factors such as pose, illumination and expression etc. Experiments on the challenging unconstrained face dataset LFW (Labeled Face in the Wild) confirm the good performance of our algorithm with the record 0.8927 compared with state-of-the-art approaches in the same experiment settings.
更多查看译文
关键词
Adaptively weighting combination, face verification, multi-classifier, visual consistency measure
AI 理解论文
溯源树
样例
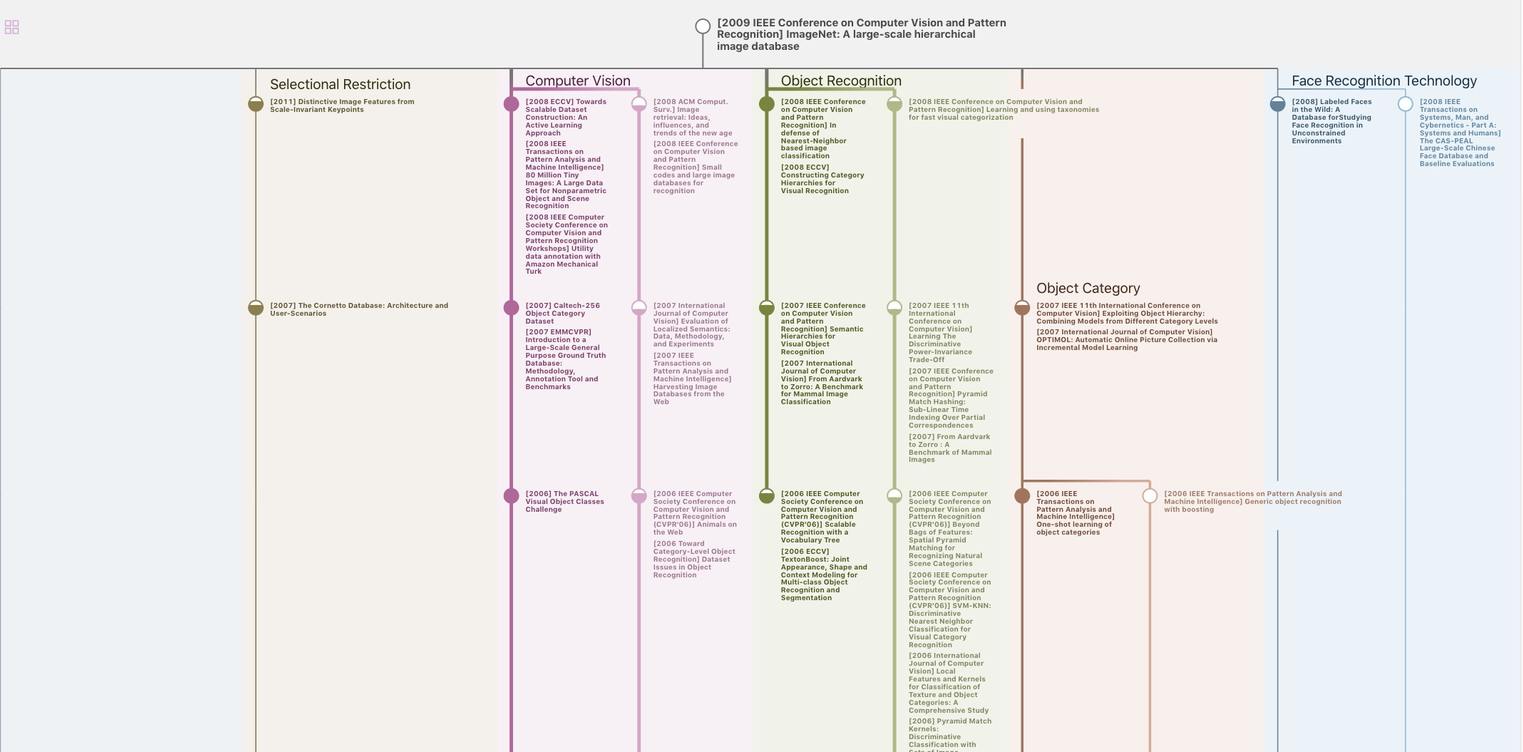
生成溯源树,研究论文发展脉络
Chat Paper
正在生成论文摘要