Recovering rank-one matrices via rank-r matrices relaxation
mag(2014)
摘要
PhaseLift, proposed by E.J. Cand\`{e}s et al., is one convex relaxation approach for phase retrieval. The relaxation enlarges the solution set from rank one matrices to positive semidefinite matrices. In this paper, a relaxation is employed to nonconvex alternating minimization methods to recover the rank-one matrices. A generic measurement matrix can be standardized to a matrix consisting of orthonormal columns. To recover the rank-one matrix, the standardized frames are used to select the matrix with the maximal leading eigenvalue among the rank-$r$ matrices. Empirical studies are conducted to validate the effectiveness of this relaxation approach. In the case of Gaussian random matrices with a sufficient number of nearly orthogonal sensing vectors, we show that the singular vector corresponding to the least singular value is close to the unknown signal, and thus it can be a good initialization for the nonconvex minimization algorithm.
更多查看译文
AI 理解论文
溯源树
样例
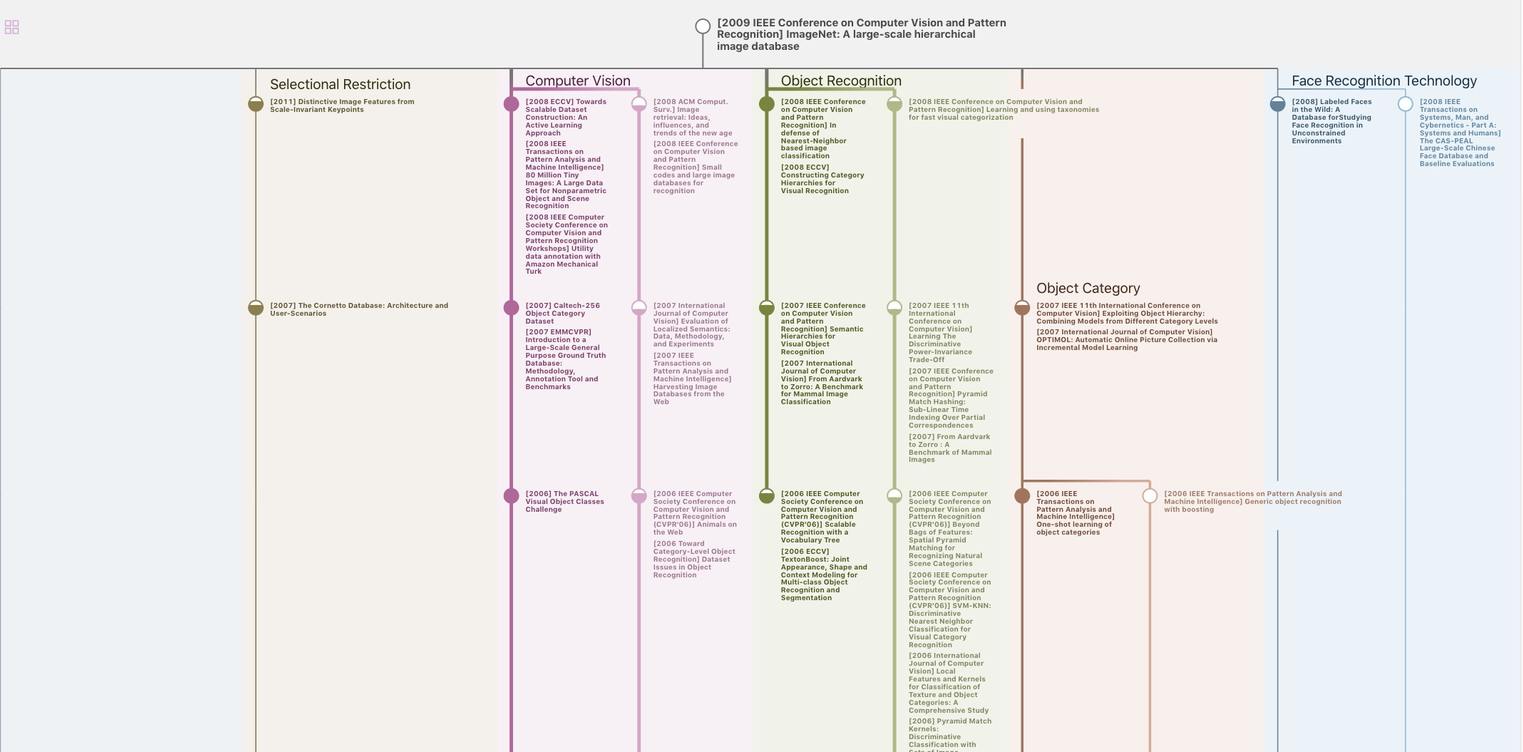
生成溯源树,研究论文发展脉络
Chat Paper
正在生成论文摘要