Semantic Language models with deep neural Networks
Computer Speech & Language(2016)
摘要
We incorporate semantic features into LMs via theory of frame semantics.We use deep autoencoders for noise robust encoding of semantic context.Deep semantic encodings on target words are more noise robust.Semantic LMs (SELMs) have a better recognition performance on target words.SELMs have a better understanding performance on the frame identification task. In this paper we explore the use of semantics in training language models for automatic speech recognition and spoken language understanding. Traditional language models (LMs) do not consider the semantic constraints and train models based on fixed-sized word histories. The theory of frame semantics analyzes word meanings and their constructs by using \"semantic frames\". Semantic frames represent a linguistic scene with its relevant participants and their relations. They are triggered by target words and include slots which are filled by frame elements. We present semantic LMs (SELMs), which use recurrent neural network architectures and the linguistic scene of frame semantics as context. SELMs incorporate semantic features which are extracted from semantic frames and target words. In this way, long-range and \"latent\" dependencies, i.e. the implicit semantic dependencies between words, are incorporated into LMs. This is crucial especially when the main aim of spoken language systems is understanding what the user means. Semantic features consist of low-level features, where frame and target information is directly used; and deep semantic encodings, where deep autoencoders are used to extract semantic features. We evaluate the performance of SELMs on publicly available corpora: the Wall Street Journal read-speech corpus and the LUNA human-human conversational corpus. The encoding of semantic frames into SELMs improves the word recognition performance and especially the recognition performance of the target words, the meaning bearing elements of semantic frames. We assess the performance of SELMs for the understanding tasks and we show that SELMs yield better semantic frame identification performance compared to recurrent neural network LMs.
更多查看译文
关键词
Language modeling,Recurrent neural networks,Frame semantics,Semantic language models,Deep autoencoders
AI 理解论文
溯源树
样例
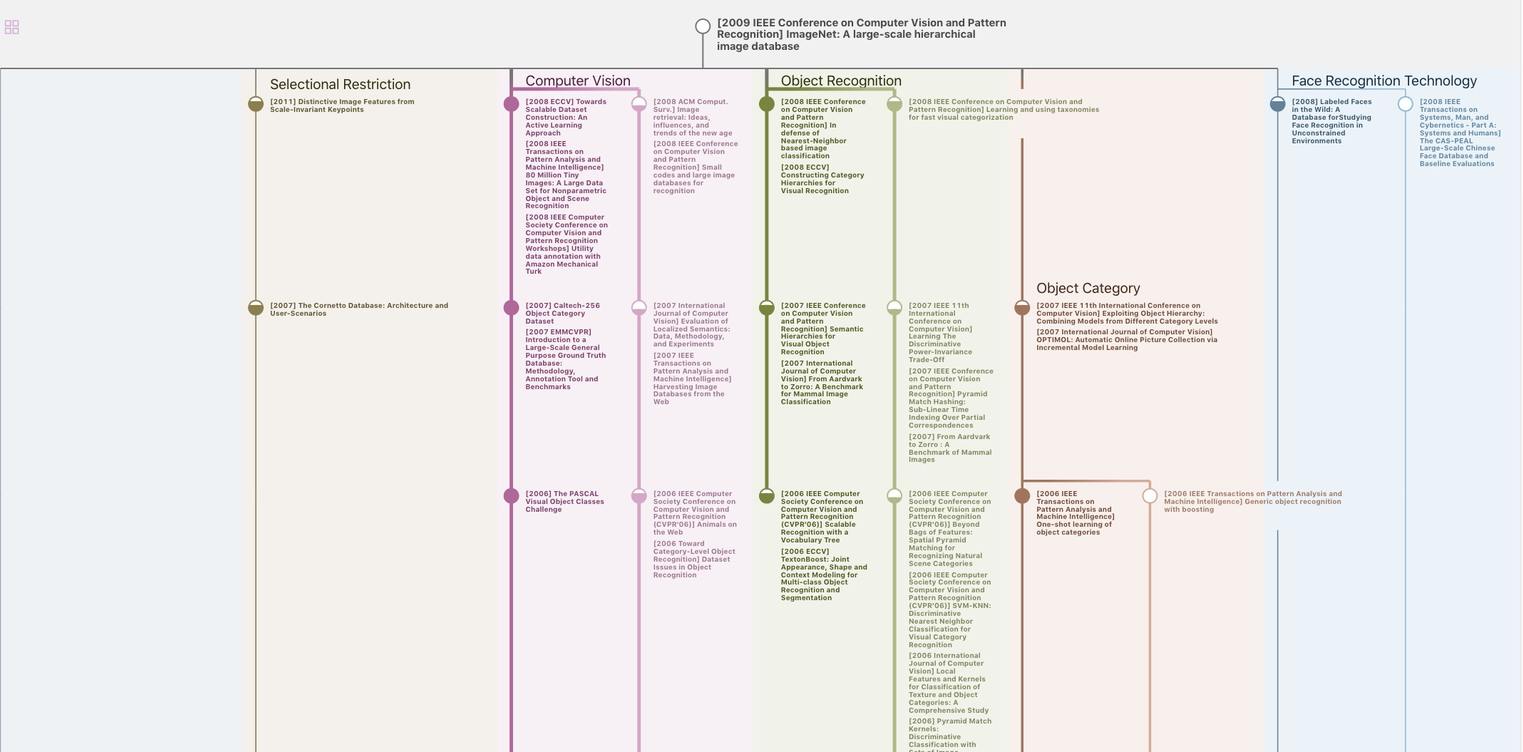
生成溯源树,研究论文发展脉络
Chat Paper
正在生成论文摘要