A low power seizure detection processor based on direct use of compressively-sensed data and employing a deterministic random matrix
2015 IEEE BIOMEDICAL CIRCUITS AND SYSTEMS CONFERENCE (BIOCAS)(2015)
摘要
This work presents a low power multi-channel seizure detection processor based on Compressive Sensing (CS) algorithm. Direct use of compressively-sensed data is proposed for feature extraction and classification in order to reduce computational and data transmission energy due to reduced number of input samples. To further reduce power consumption of the system, using a deterministic random matrix (DRM) is proposed instead of implementing a random number generator circuit (LFSR) for compressive sensing circuit. For feature extraction, simple features are used and for classification Logistic Regression (LR) is employed. Three different architectures are implemented in Virtex-5 FPGA and are compared against each other. For compression rates of 2-16x, the energy consumption of the proposed 22-channel seizure detection processor including CS, feature extractor and classifier is 2.36-0.38 μJ and the detector performance for sensitivity and specificity is 80.7-78.8% and 85.3-83.5%, respectively. The proposed system with 16x compression rate consumes 0.38 μJ which is 6 times lower than the system without using compressive sensing.
更多查看译文
关键词
Compressive Sensing,Seizure Detection,Logistic Regression,FPGA,Energy Efficiency,ASIC
AI 理解论文
溯源树
样例
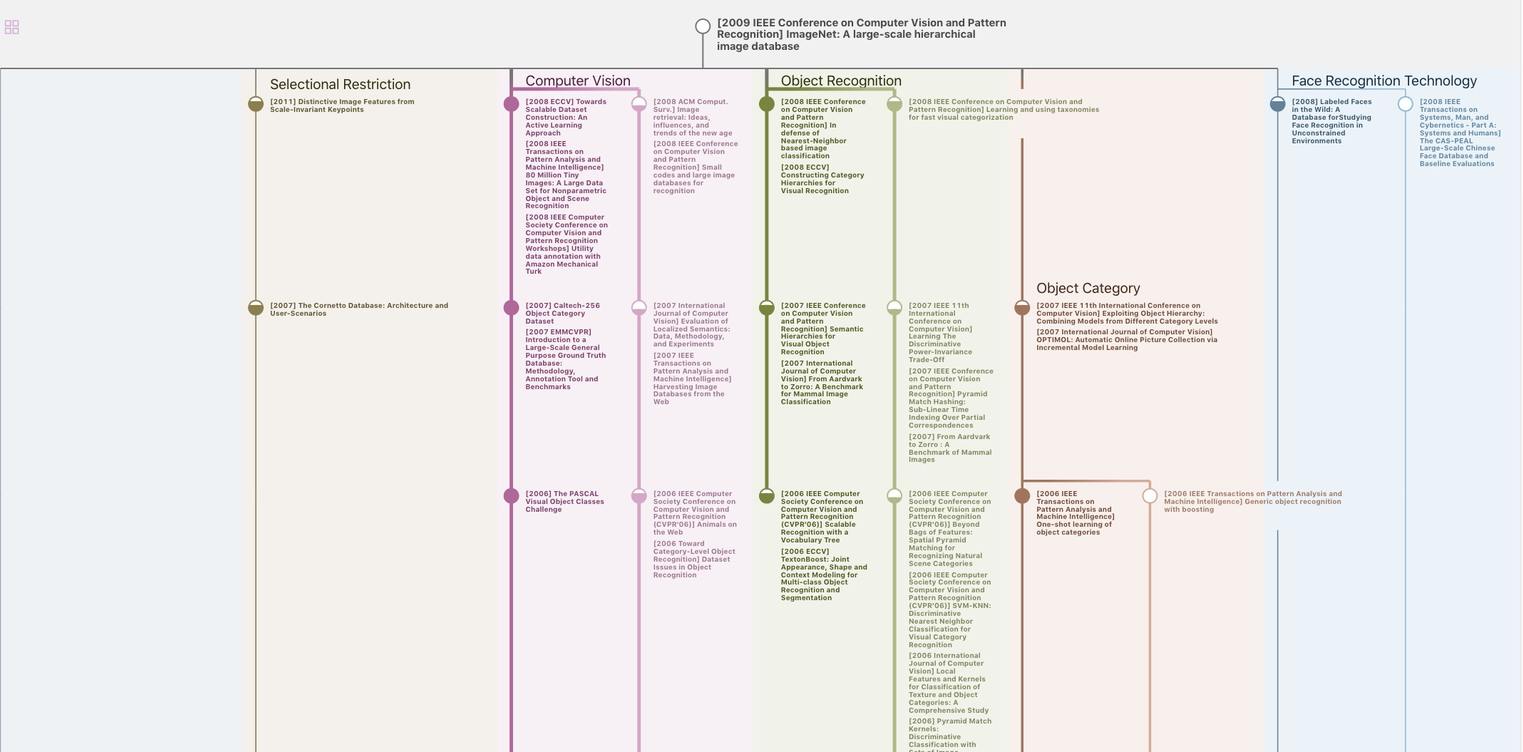
生成溯源树,研究论文发展脉络
Chat Paper
正在生成论文摘要