Particle Learning for Sequential Bayesian Computation – Rejoinder
mag(2010)
摘要
We would like to enthusiastically thank the discussants Mike Pitt, Christian Robert’s multinational team, Paul Fearnhead and Dan Merl for their contributions. Hopefully our comments will make PL’s scope, strengths and weaknesses clear, particularly to those readers interested in sequential parameter Bayesian computation. We would like to organize our comments into the following topics: approximating predictive densities, outliers and model misspecification, sufficient statistics, MC error accumulation, PL and MCMC and resampling. Pitt’s discussion is mainly focused on PL for dynamic models (Carvalho et al., 2010, Lopes and Tsay, 2010). Similarly, several of Robert et al. discussion are based on the mixture of Poisson distributions from Carvalho et al. (2009). Therefore, some readers might benefit from browsing through those papers before engaging in our comments. Fearnhead’s and Merl’s discussion are solely based on our chapter.
更多查看译文
AI 理解论文
溯源树
样例
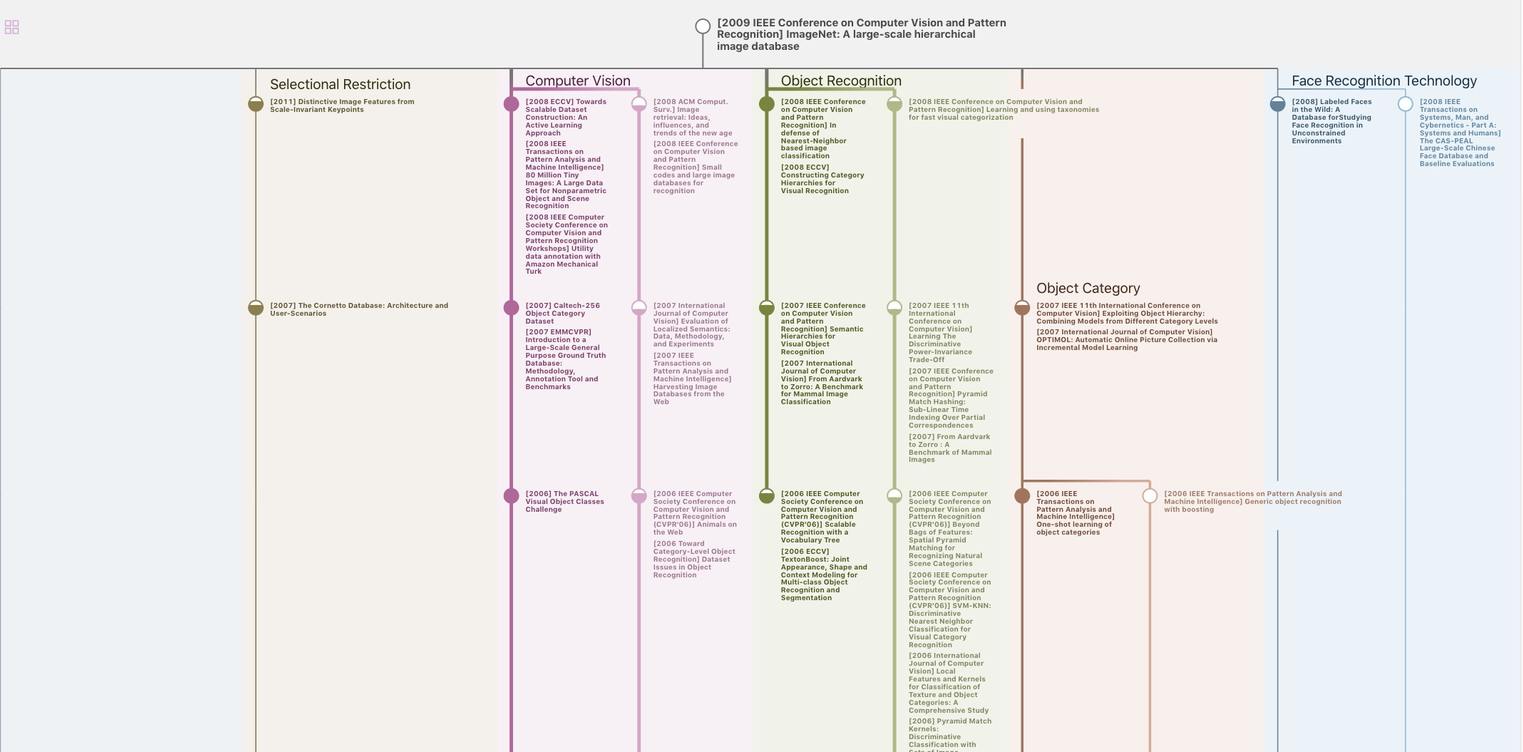
生成溯源树,研究论文发展脉络
Chat Paper
正在生成论文摘要