Adaptive learning with covariate shift-detection for motor imagery-based brain–computer interface
Soft Computing - A Fusion of Foundations, Methodologies and Applications(2015)
摘要
A common assumption in traditional supervised learning is the similar probability distribution of data between the training phase and the testing/operating phase. When transitioning from the training to testing phase, a shift in the probability distribution of input data is known as a covariate shift. Covariate shifts commonly arise in a wide range of real-world systems such as electroencephalogram-based brain–computer interfaces (BCIs). In such systems, there is a necessity for continuous monitoring of the process behavior, and tracking the state of the covariate shifts to decide about initiating adaptation in a timely manner. This paper presents a covariate shift-detection and -adaptation methodology, and its application to motor imagery-based BCIs. A covariate shift-detection test based on an exponential weighted moving average model is used to detect the covariate shift in the features extracted from motor imagery-based brain responses. Following the covariate shift-detection test, the methodology initiates an adaptation by updating the classifier during the testing/operating phase. The usefulness of the proposed method is evaluated using real-world BCI datasets (i.e. BCI competition IV dataset 2A and 2B). The results show a statistically significant improvement in the classification accuracy of the BCI system over traditional learning and semi-supervised learning methods.
更多查看译文
关键词
Adaptive learning, Brain–computer interfaces, Covariate shift-detection, Transductive learning
AI 理解论文
溯源树
样例
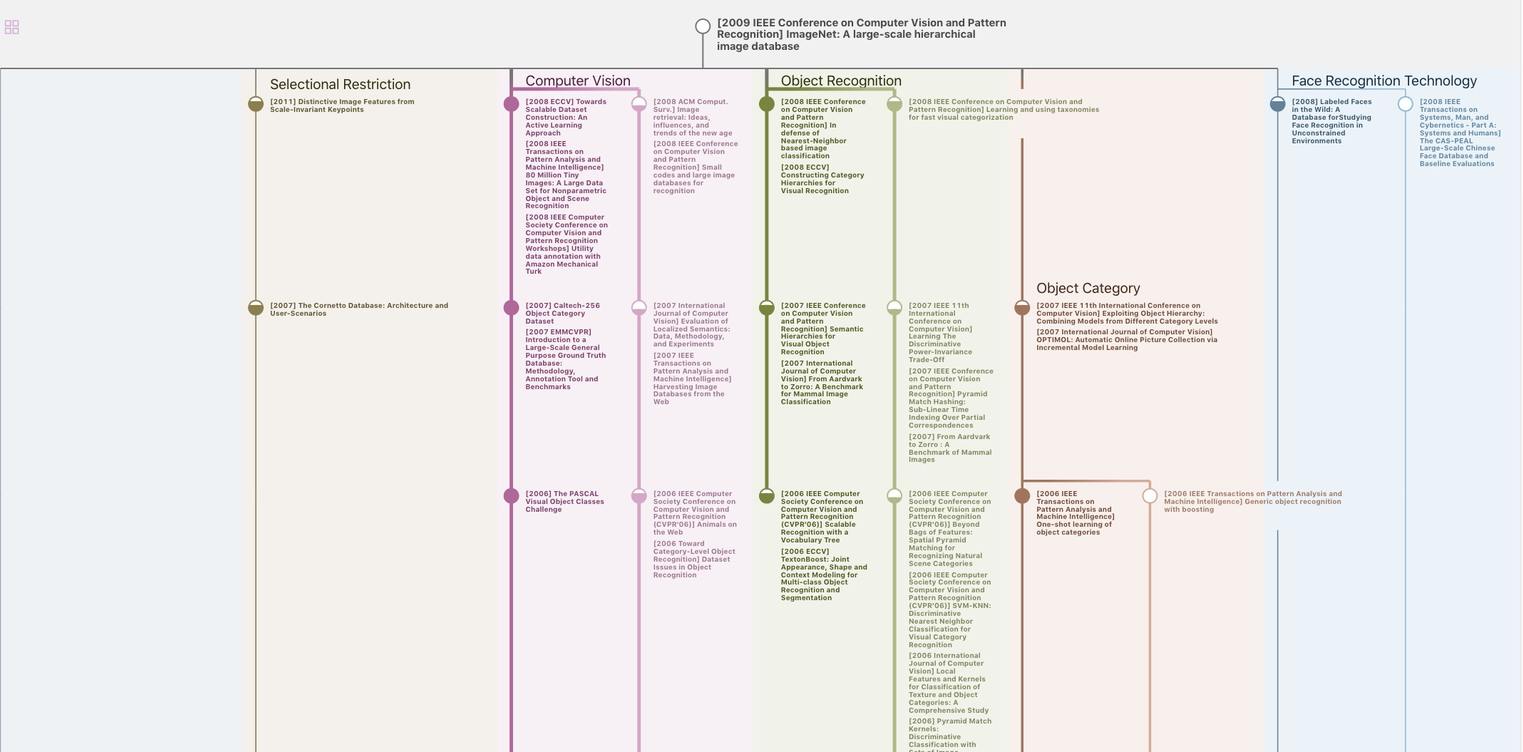
生成溯源树,研究论文发展脉络
Chat Paper
正在生成论文摘要