From variable density sampling to continuous sampling using Markov chains
mag(2013)
摘要
Since its discovery over the last decade, Compressed Sensing (CS) has been successfully applied to Magnetic Reso- nance Imaging (MRI). It has been shown to be a powerful way to reduce scanning time without sacrificing image quality. MR images are actually strongly compressible in a wavelet basis, the latter being largely incoherent with the k-space or spatial Fourier domain where acquisition is performed. Nevertheless, since its first application to MRI [1], the theoretical justification of actual k-space sampling strategies is questionable. Indeed, the vast majority of k-space sampling distributions have been heuris- tically designed (e.g., variable density) or driven by experimental feasibility considerations (e.g., random radial or spiral sampling to achieve smoothness k-space trajectory). In this paper, we try to reconcile very recent CS results with the MRI specificities (mag- netic field gradients) by enforcing the measurements, i.e. samples of k-space, to fit continuous trajectories. To this end, we propose random walk continuous sampling based on Markov chains and we compare the reconstruction quality of this scheme to the state- of-the art.
更多查看译文
关键词
random walks,markov chains,compressive sensing,mri,wavelets
AI 理解论文
溯源树
样例
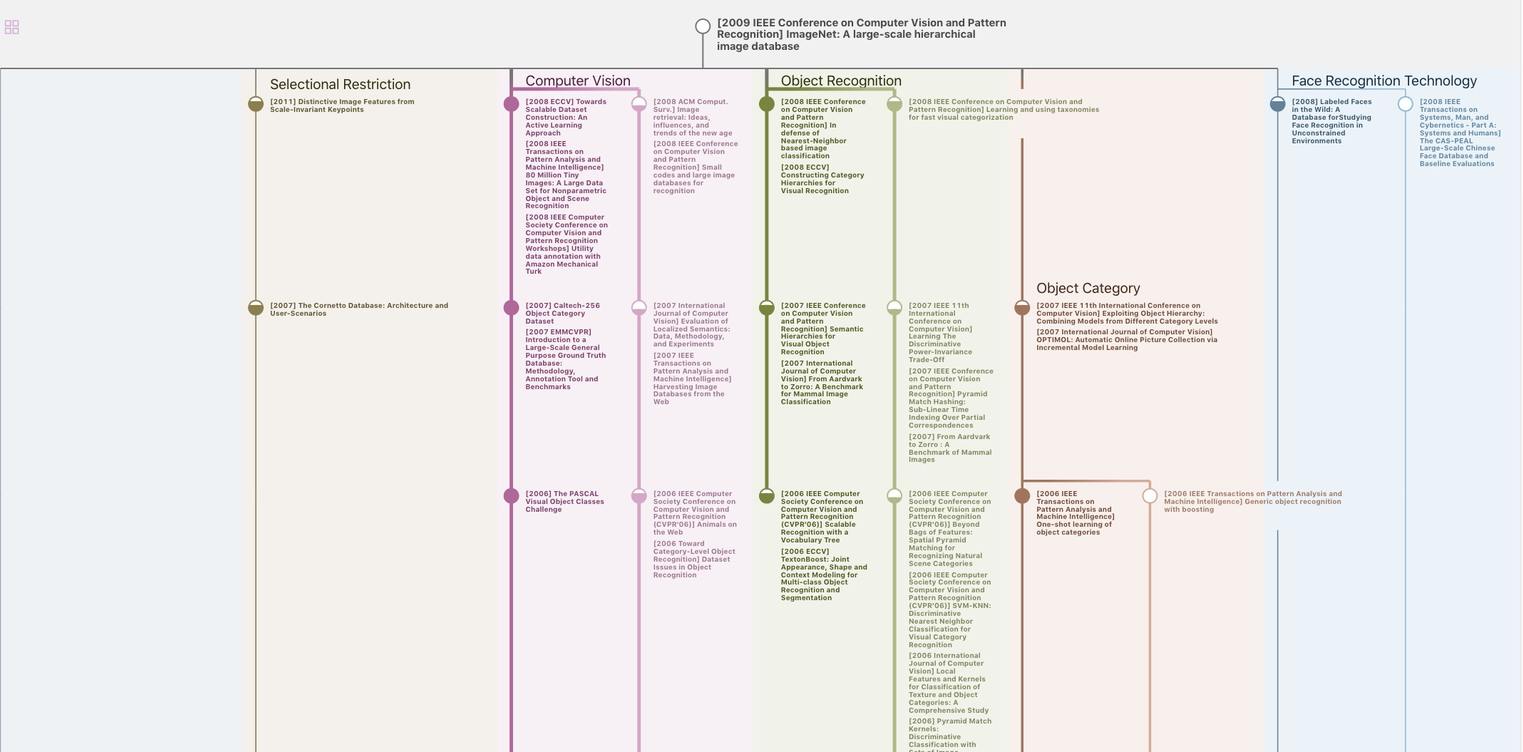
生成溯源树,研究论文发展脉络
Chat Paper
正在生成论文摘要