Subsampling-Based Compression And Flow Visualization
VISUALIZATION AND DATA ANALYSIS 2015(2015)
摘要
As computational capabilities increasingly outpace disk speeds on leading supercomputers, scientists will, in turn, be increasingly unable to save their simulation data at its native resolution. One solution to this problem is to compress these data sets as they are generated and visualize the compressed results afterwards. We explore this approach, specifically subsampling velocity data and the resulting errors for particle advection-based flow visualization. We compare three techniques: random selection of subsamples, selection at regular locations corresponding to multi-resolution reduction, and introduce a novel technique for informed selection of subsamples. Furthermore, we explore an adaptive system which exchanges the subsampling budget over parallel tasks, to ensure that subsampling occurs at the highest rate in the areas that need it most. We perform supercomputing runs to measure the effectiveness of the selection and adaptation techniques. Overall, we find that adaptation is very effective, and, among selection techniques, our informed selection provides the most accurate results, followed by the multi-resolution selection, and with the worst accuracy coming from random subsamples.
更多查看译文
关键词
In Situ,Sub-Sampling,Compression,Adaptive Budget,Flow Visualization
AI 理解论文
溯源树
样例
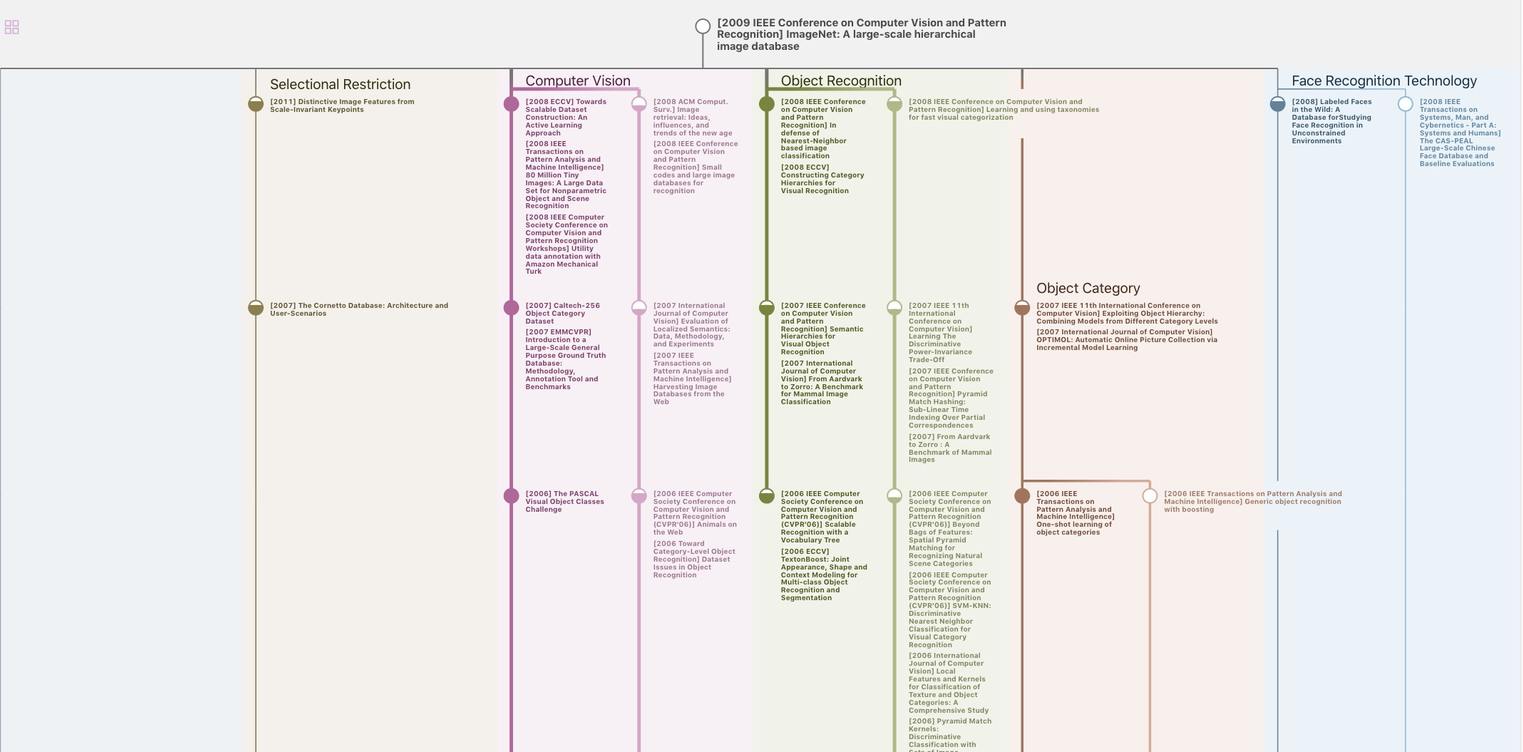
生成溯源树,研究论文发展脉络
Chat Paper
正在生成论文摘要