Marked Point Processes for Microarray Data Clustering
Studies in Classification Data Analysis and Knowledge Organization(2017)
摘要
Microarray technologies become a powerful technique for simultaneously monitoring expression patterns of thousands of genes under different conditions. However, it is important to identify gene groups that manifest similar expression profiles and are activated by similar conditions. ClusterMPP: Clustering by Marked Point Process is a new microarray data clustering algorithm performed in two steps. The first one detects cluster modes representing regions of high density observations in the raw space. Based on the simulation of a proposed Marked Point Process by the well-known Reversible Jump Markov Chain Monte Carlo algorithm, where we consider several movements like birth and death, this algorithm step identifies prototype observations of each cluster. The second step of ClusterMPP is the K nearest neighbors (KNN) assignation that affects the remaining observations to the corresponding clusters. We experiment ClusterMPP on several complex and scalable microarray datasets. The results show the efficiency of ClusterMPP compared to well-known microarray data clustering methods like K-means, Spectral Clustering, and Mean-Shift.
更多查看译文
关键词
Marked Point Process (MPP), Clustering Microarray Data, Reversible Jump Markov Chain Monte Carlo (RJMCMC), Prototype Observations, Cluster Modes
AI 理解论文
溯源树
样例
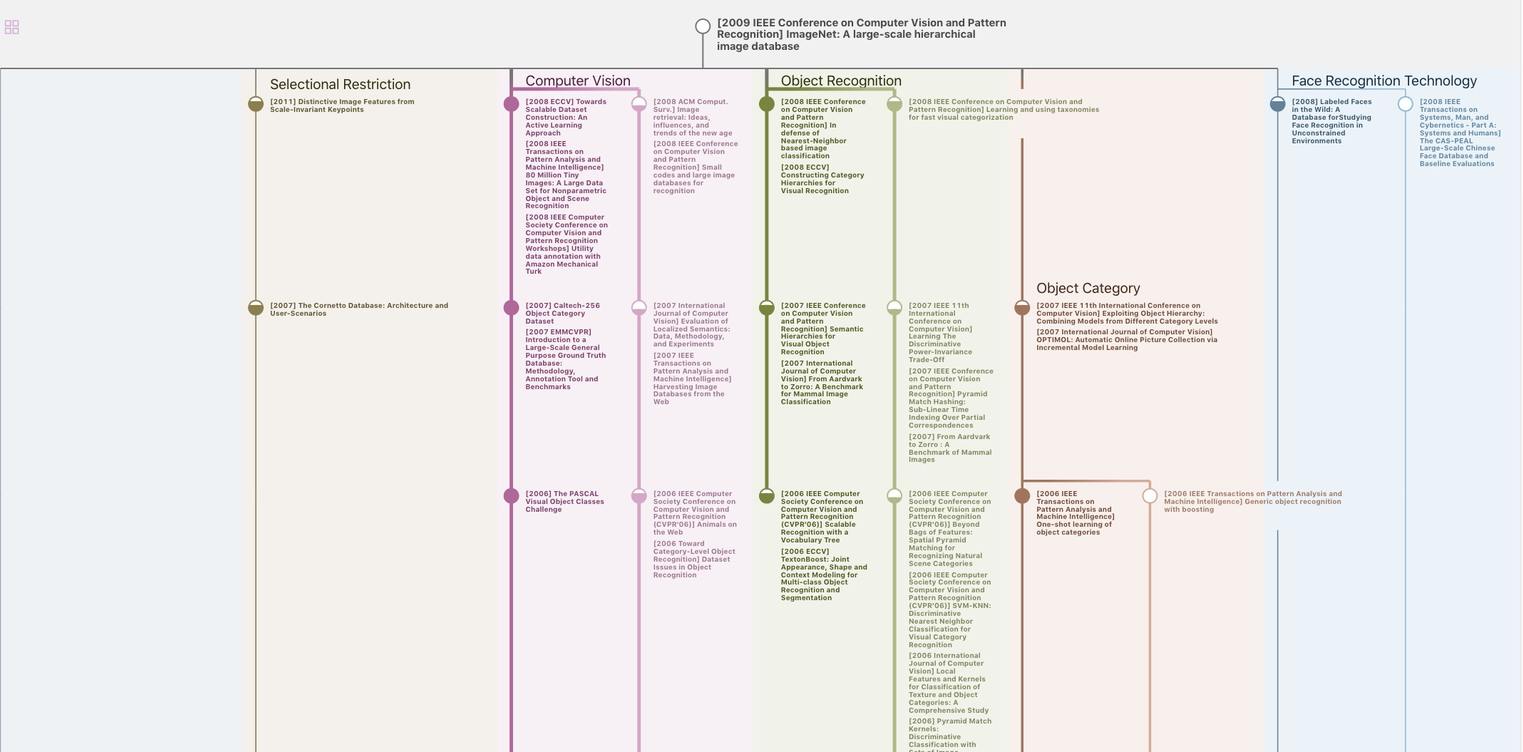
生成溯源树,研究论文发展脉络
Chat Paper
正在生成论文摘要