Verification Of Directed Self-Assembly (Dsa) Guide Patterns Through Machine Learning
ALTERNATIVE LITHOGRAPHIC TECHNOLOGIES VII(2015)
摘要
Verification of full-chip DSA guide patterns (GPs) through simulations is not practical due to long runtime. We develop a decision function (or functions), which receives n geometry parameters of a GP as inputs and predicts whether the GP faithfully produces desired contacts (good) or not (bad). We take a few sample GPs to construct the function; DSA simulations are performed for each GP to decide whether it is good or bad, and the decision is marked in n-dimensional space. The hyper-plane that separates good marks and bad marks in that space is determined through machine learning process, and corresponds to our decision function. We try a single global function that can be applied to any GP types, and a series of functions in which each function is customized for different GP type; they are then compared and assessed in 10nm technology.
更多查看译文
关键词
Directed self-assembly (DSA), verification, machine learning, SVM
AI 理解论文
溯源树
样例
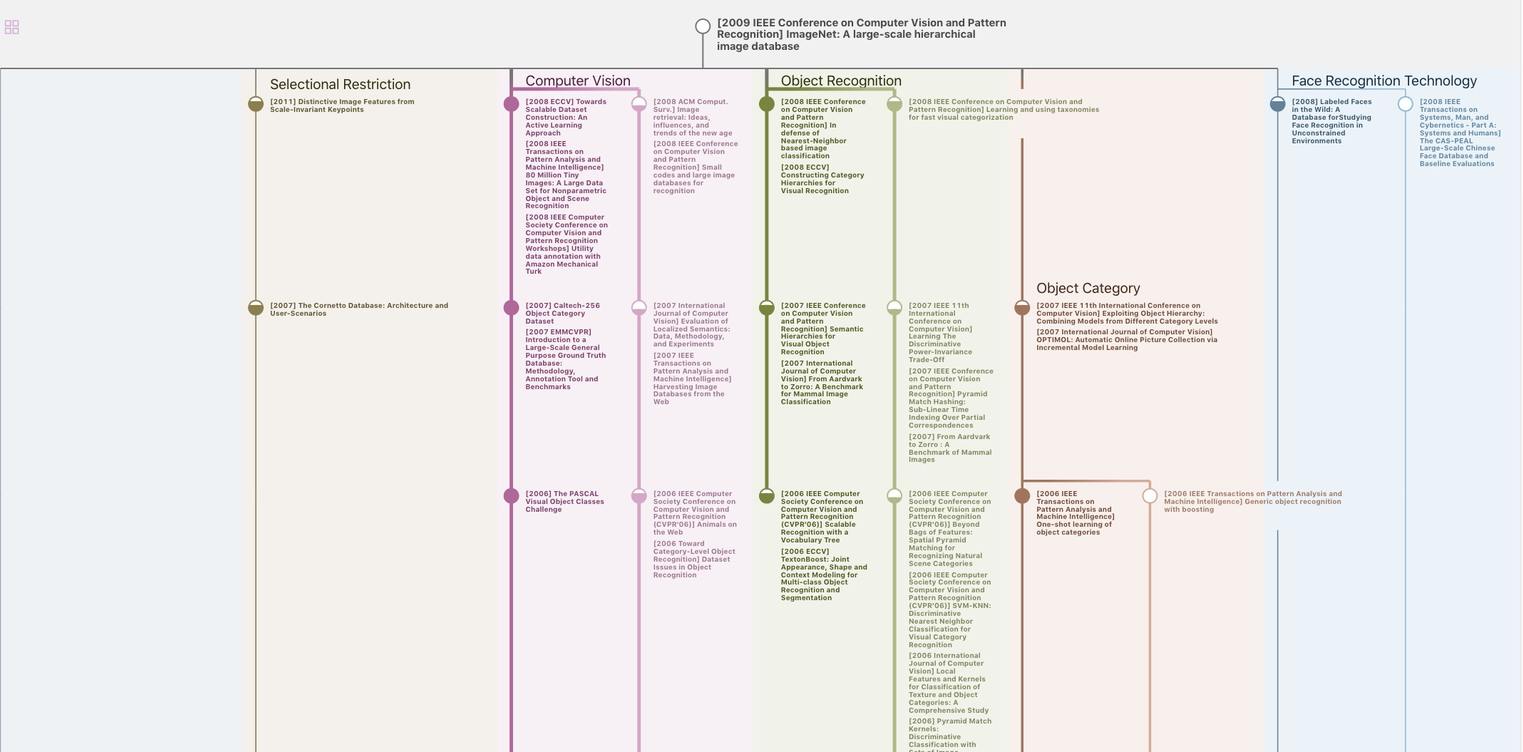
生成溯源树,研究论文发展脉络
Chat Paper
正在生成论文摘要