Optimal Trending and Filtering Using Monotonic Walk Models
mag(2014)
摘要
This paper discusses algorithms for filtering and recovering underlying trends from noisy data. The key assumption in this paper is that the trends are monotonic, e.g., describe system deterioration that accumulates irreversibly. A maximum a posteriori probability (MAP) estimate of the trend can be obtained using an empirical signal model (MAP prior). The overall problem statement is related to Kalman filtering setting. The main difference is that a one-sided exponentially distributed process noise is assumed instead of a Gaussian noise. We consider nonlinear filters obtained using firstor second-order monotonic walk trend models as the MAP estimation priors. A batch estimate is computed by solving a Quadratic Programming (QP) problem. A first-order monotonic walk prior results in an isotonic regression problem, a special QP problem for which very efficient algorithms exist. Analysis and simulations show that the approach works exceptionally well for trends with that have a small number of jumps in the trended variable or its increase rate and do not change between the jumps. Theoretical analysis of the smoothing and filtering behaviours of the proposed algorithms is presented to explain and complement the simulation results. We show that similar to other l1 regularized overdetermined systems, the solutions have certain sparsity properties.
更多查看译文
AI 理解论文
溯源树
样例
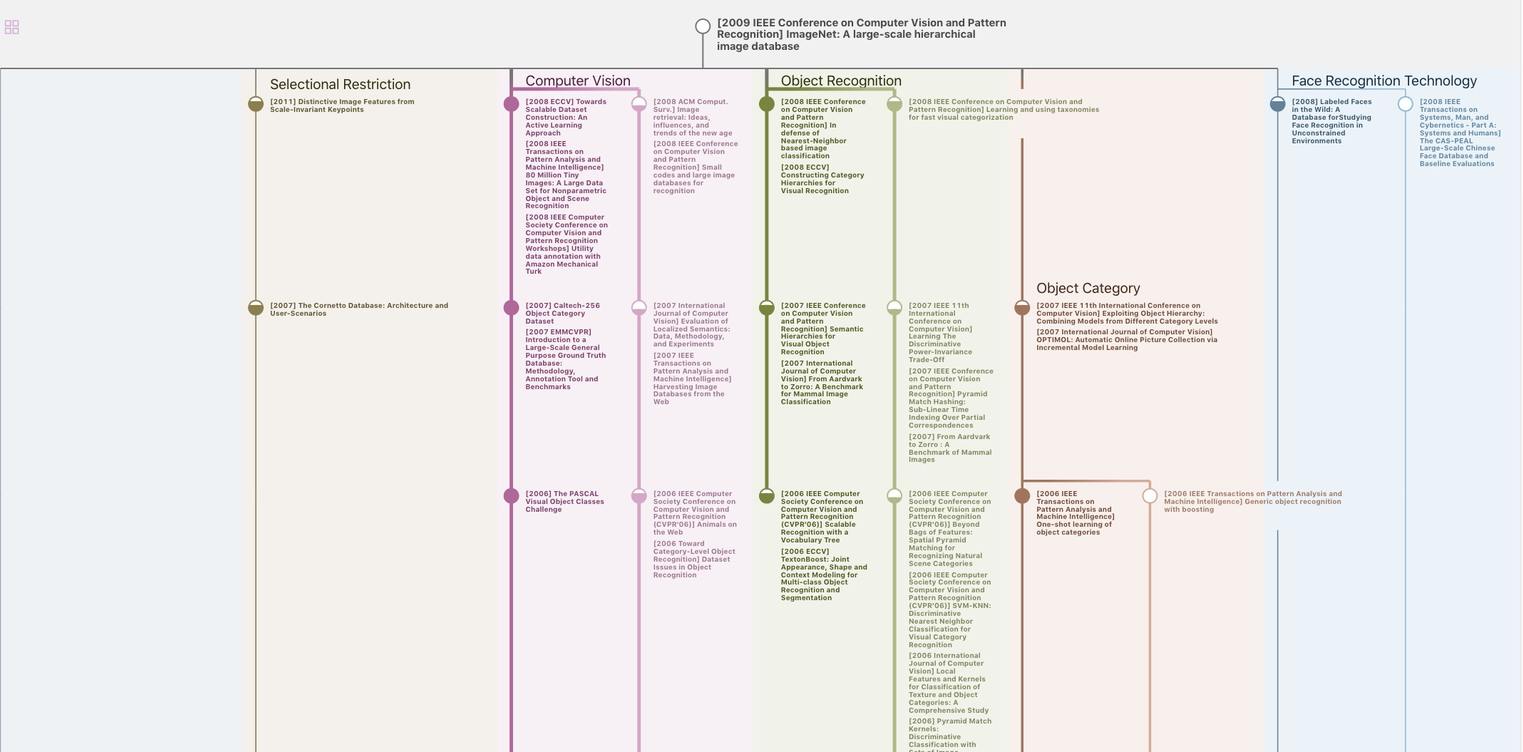
生成溯源树,研究论文发展脉络
Chat Paper
正在生成论文摘要