A dynamic ensemble approach to robust classification in the presence of missing data
Machine Learning(2015)
摘要
Many real-world datasets suffer from missing or incomplete data. In the healthcare setting, for example, certain patient measurement parameters, such as vitals and/or lab values, may be missing due to insufficient monitoring. When present, however, these features could be highly discriminative in predicting aspects of patient state. Therefore, it is desirable to incorporate these sparsely measured features into a predictive model. Training predictive algorithms on such datasets is complicated by the missing data. Overcoming this problem is usually achieved by first estimating values for the missing data, which is referred to as data imputation. Without strong prior knowledge about the relationship between features though, it is common to fill in missing values with their respective population mean or median. The accuracy of this approach is limited, however, and may simply inject noise into the data. We propose a two-stage machine learning algorithm that learns a dynamic classifier ensemble from an incomplete dataset without data imputation. The algorithm is very simple to implement and applicable across a wide range of problems. Our method first employs a variant of AdaBoost to learn a set of low-dimensional classifiers, each of which abstains from predicting if its dependent feature(s) are missing. Our novel contribution is the secondary dynamic ensemble learning stage in which the low-dimensional classifiers are combined using a dynamic weighting that depends on the pattern of measured features in the present input data. This allows the model to be resilient to missing data by adjusting the strength of certain classifiers to account for missing features. We apply our algorithm to early detection of hemodynamic instability in ICU patients. Providing an effective risk score of hemodynamic instability has the potential to give the clinician sufficient time to intervene, thereby reducing the chance of organ damage due to insufficient blood perfusion. We compare the results of our algorithm to other common missing data approaches, including mean imputation and multiple imputation methods, and discuss the advantages of the approach given the constraints of the application domain (e.g., high specificity to combat hospital alarm fatigue).
更多查看译文
关键词
Missing data,Ensemble methods,Hemodynamic instability
AI 理解论文
溯源树
样例
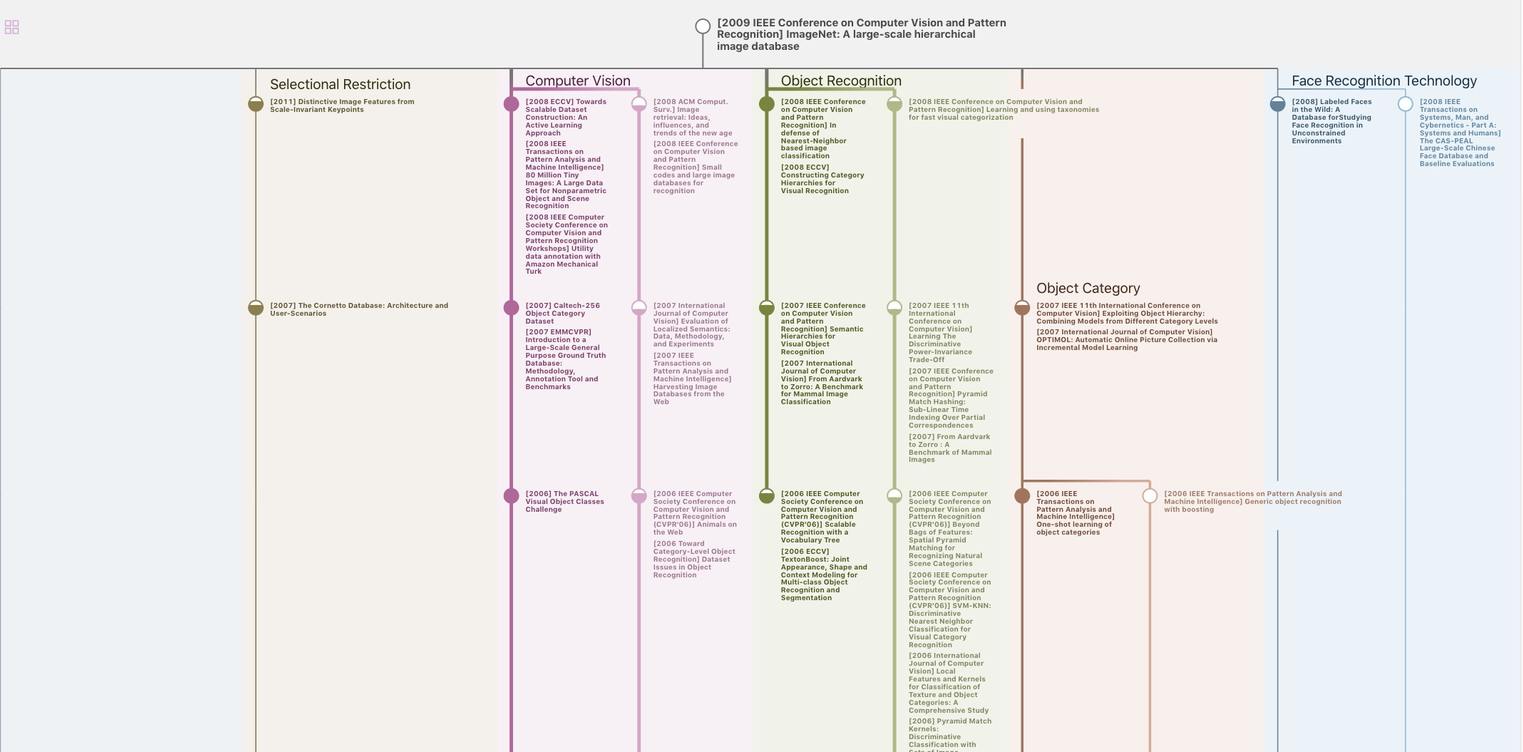
生成溯源树,研究论文发展脉络
Chat Paper
正在生成论文摘要