The principal correlation components estimator and its optimality
Statistical Papers(2015)
摘要
In regression analysis, the principal components regression estimator (PCRE) is often used to alleviate the effect of multicollinearity by deleting the principal components variables with smaller eigenvalues, but it has some drawbacks for subset selection. So another effective estimator based on the principal components, termed the principal correlation components estimator (PCCE), has been proposed, which selects the variables by considering the correlation coefficients between the principal components variables and the response variable. In this paper we investigate the property of the PCCE and its optimality. We prove that the PCCE is an admissible estimator and obtain the condition that the PCCE performs better than the PCRE under the balanced loss function (BLF). As an improvement of the least squares estimator (LSE), the conditions that the PCCE performs better than LSE under the BLF and the Pitman closeness criterion are derived in the paper. The empirical performance of the PCCE is demonstrated by several real and simulation data examples.
更多查看译文
关键词
Principal correlation components estimator,Least squares estimator,Principal components regression estimator,Admissible estimator,Balanced loss function,Pitman closeness criterion
AI 理解论文
溯源树
样例
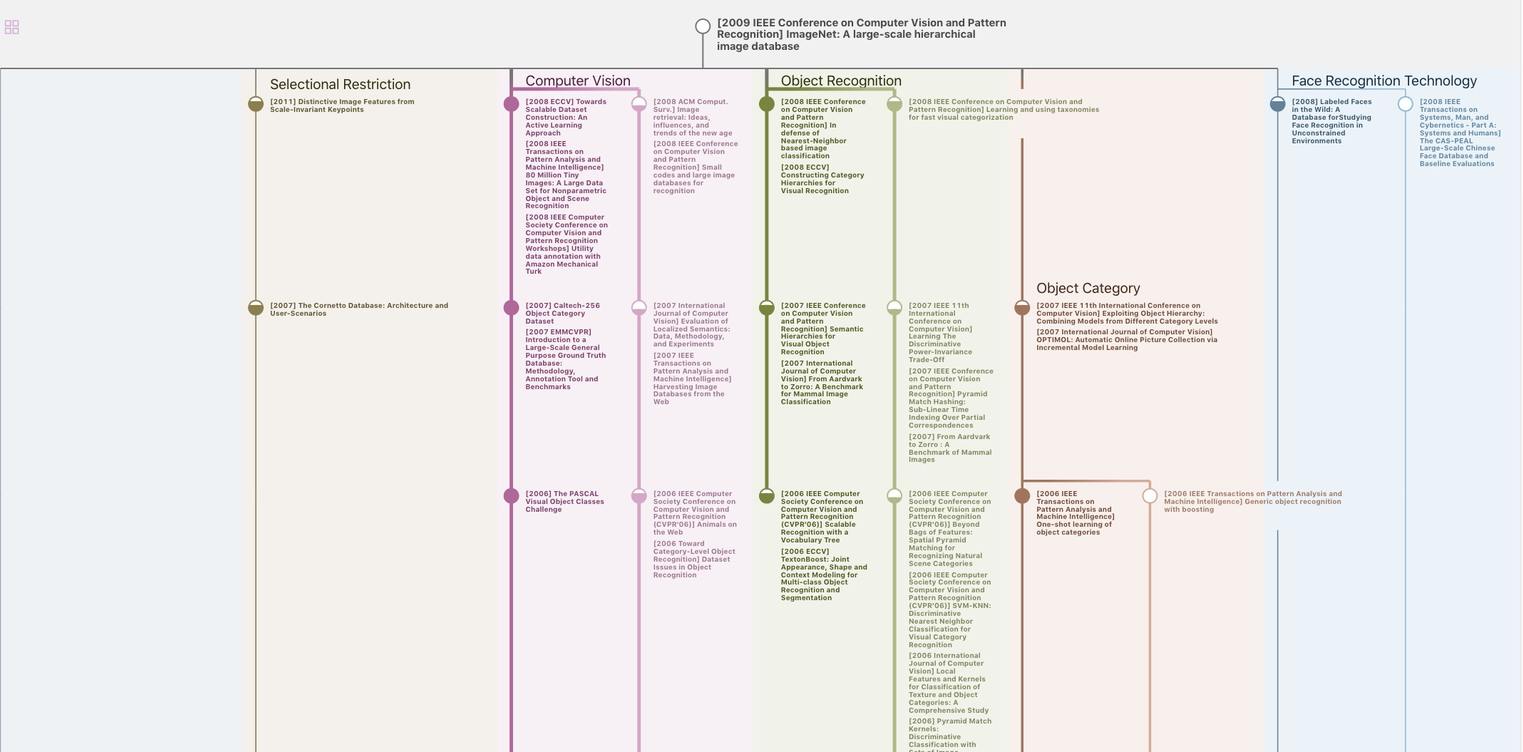
生成溯源树,研究论文发展脉络
Chat Paper
正在生成论文摘要