Abstract A53: Bayesian Network Inference Modeling Reveals Novel Regulators of Cell Cycle Progression and Survival
CLINICAL CANCER RESEARCH(2012)
摘要
Molecular networks governing responses to targeted therapies in cancer cells are complex dynamic systems that demonstrate non-intuitive behaviors. We applied a novel computational strategy to decipher causal relationships between signaling network components. Gene networks were constructed from global gene expression profiles to model G1-S cell cycle arrest as a consequence of inhibition of MEK, a key component of the oncogenic RAF-MEK-ERK signal transduction pathway. Simulation of causally driven models generated a ranked list of 12 testable hypotheses, which confirmed role of known cell cycle regulators (CCND1, CCNE2, and CDC25A) and identified novel drivers of G1-S transition (IER2, TRIB1, C14ORF133). Validation confirmed 11 out 12 predictions. Second model which inferred causal relationships between genes lead to discovery of TRIB1 (tribbles homolog of Drosophila) as a regulator of NFkB-dependent gene expression. TRIB1 also mediates cross-talk to the NFκB pathway by enhancing p100 and IκBα-phosphorylation and degradation thus regulating cell survival and expression of cytokines crucial for tumor-stroma interaction. In agreement with this, knock-down of TRIB1 results in induction of apoptosis and sensitizes cells to cell killing induced by the death-receptor agonist TRAIL via upregulation of TRAIL receptor, DR5. Thus TRIB1 is a central regulator of cell cycle and survival and represents a potential therapeutic target. Therefore, applying REFS (Reverse Engineering Forward Simulation) to analyze gene expression profiles maximizes our ability to extract knowledge from experimental data.
更多查看译文
AI 理解论文
溯源树
样例
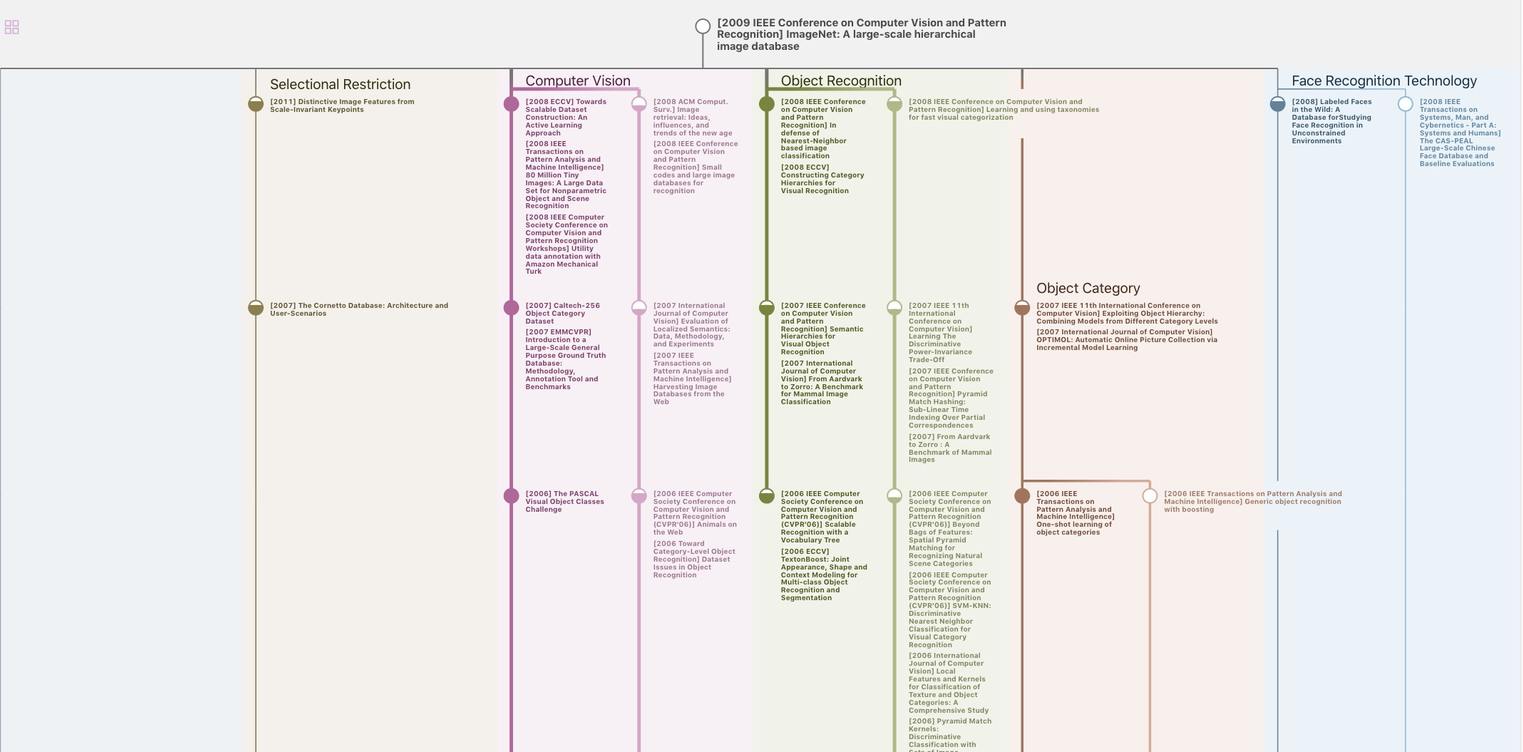
生成溯源树,研究论文发展脉络
Chat Paper
正在生成论文摘要