Applying long short-term memory recurrent neural networks to intrusion detection
South African Computer Journal(2018)
摘要
These days, web applications are used extensively. While organizations benefit from the new abilities they provide, the chance of being targeted is increased, which may cause massive system damage. It is thus important to detect web application attacks. Web intrusion detection systems (IDSs) are important for protecting systems from external users or internal attacks. There are however, many challenges that arise while developing a powerful IDS for unexpected and irregular attacks. Deep Learning approaches provide several methods, and they can detect known and unknown attacks. Long Short-Term Memory (LSTM) is a type of Recurrent Neural Network (RNN) and has the ability to remember values over arbitrary intervals. LSTM is a suitable method to classify and predict known and unknown intrusions. In this work, we propose a deep learning approach to construct an IDS. We apply LSTM RNNs and train the model using the CSIC 2010 HTTP dataset. An LSTM model using the Adam optimizer can construct an efficient IDS binary classifier with an accuracy rate of 0.9997.
更多查看译文
关键词
receiver operating characteristic,long short term memory,time series analysis,recurrent neural network,machine learning,intrusion detection systems
AI 理解论文
溯源树
样例
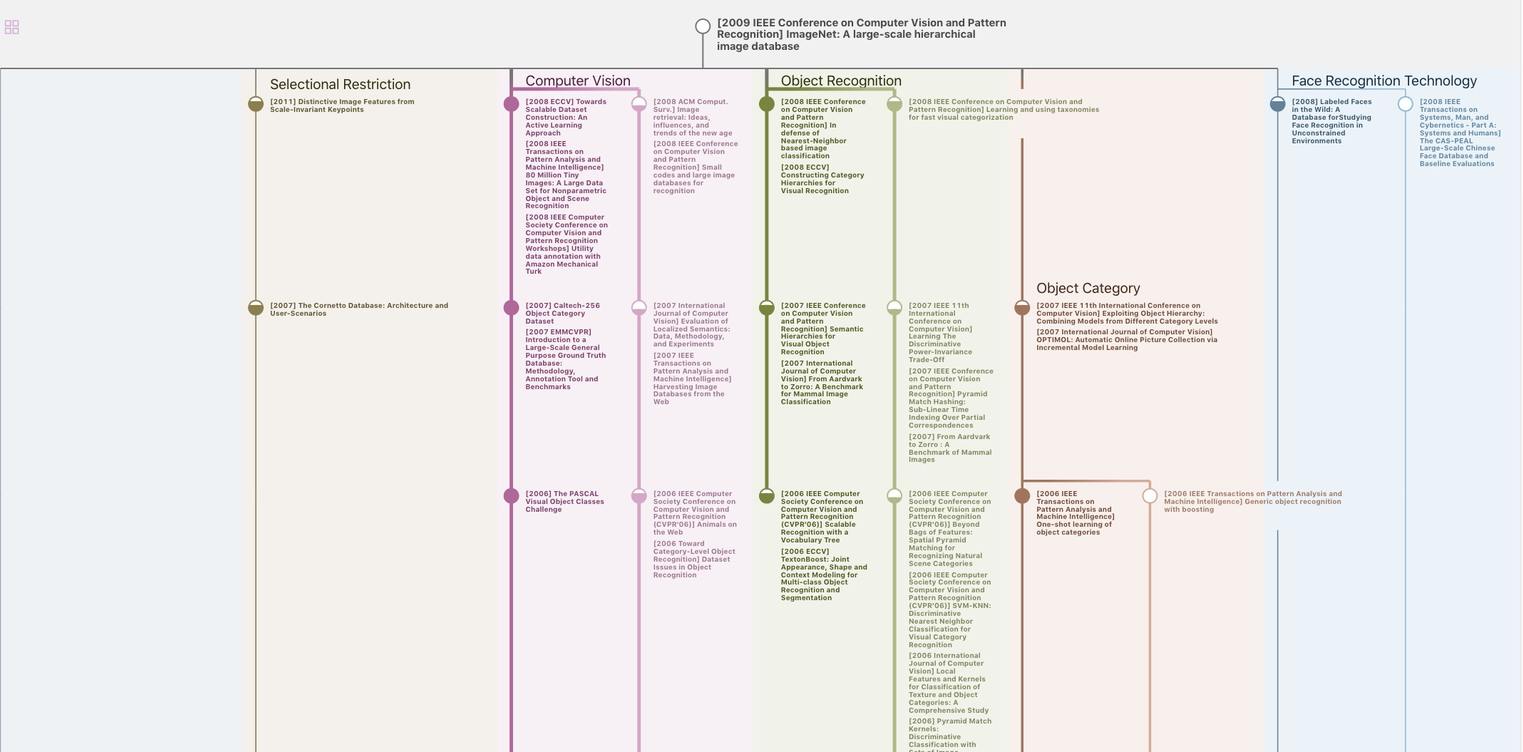
生成溯源树,研究论文发展脉络
Chat Paper
正在生成论文摘要