A Unified Algorithmic Framework for Block-Structured Optimization Involving Big Data: With applications in machine learning and signal processing
Signal Processing Magazine, IEEE(2016)
摘要
This article presents a powerful algorithmic framework for big data optimization, called the block successive upper-bound minimization (BSUM). The BSUM includes as special cases many well-known methods for analyzing massive data sets, such as the block coordinate descent (BCD) method, the convex-concave procedure (CCCP) method, the block coordinate proximal gradient (BCPG) method, the nonnegative matrix factorization (NMF) method, the expectation maximization (EM) method, etc. In this article, various features and properties of the BSUM are discussed from the viewpoint of design flexibility, computational efficiency, parallel/distributed implementation, and the required communication overhead. Illustrative examples from networking, signal processing, and machine learning are presented to demonstrate the practical performance of the BSUM framework.
更多查看译文
关键词
Signal processing algorithms,Optimization,Big data,Algorithm design and analysis,Approximation methods,Machine learning algorithms,Upper bound
AI 理解论文
溯源树
样例
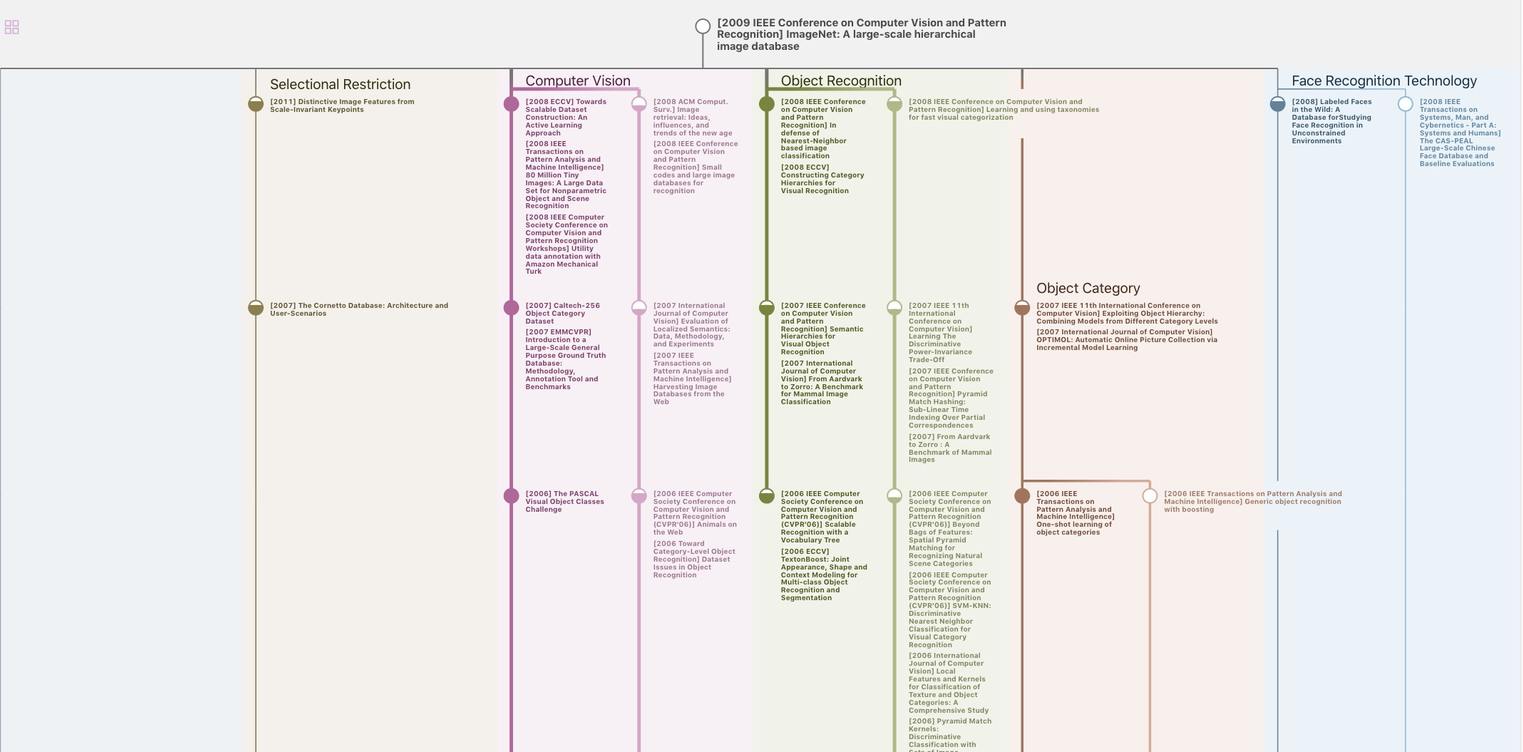
生成溯源树,研究论文发展脉络
Chat Paper
正在生成论文摘要