Accurate and efficient target prediction using a potency-sensitive influence-relevance voter
Journal of Cheminformatics(2015)
摘要
Background A number of algorithms have been proposed to predict the biological targets of diverse molecules. Some are structure-based, but the most common are ligand-based and use chemical fingerprints and the notion of chemical similarity. These methods tend to be computationally faster than others, making them particularly attractive tools as the amount of available data grows. Results Using a ChEMBL-derived database covering 490,760 molecule-protein interactions and 3236 protein targets, we conduct a large-scale assessment of the performance of several target-prediction algorithms at predicting drug-target activity. We assess algorithm performance using three validation procedures: standard tenfold cross-validation, tenfold cross-validation in a simulated screen that includes random inactive molecules, and validation on an external test set composed of molecules not present in our database. Conclusions We present two improvements over current practice. First, using a modified version of the influence-relevance voter (IRV), we show that using molecule potency data can improve target prediction. Second, we demonstrate that random inactive molecules added during training can boost the accuracy of several algorithms in realistic target-prediction experiments. Our potency-sensitive version of the IRV (PS-IRV) obtains the best results on large test sets in most of the experiments. Models and software are publicly accessible through the chemoinformatics portal at http://chemdb.ics.uci.edu/
更多查看译文
关键词
Target-prediction,Large-scale,Fingerprints,Molecular potency,Random inactive molecules,Influence-relevance voter
AI 理解论文
溯源树
样例
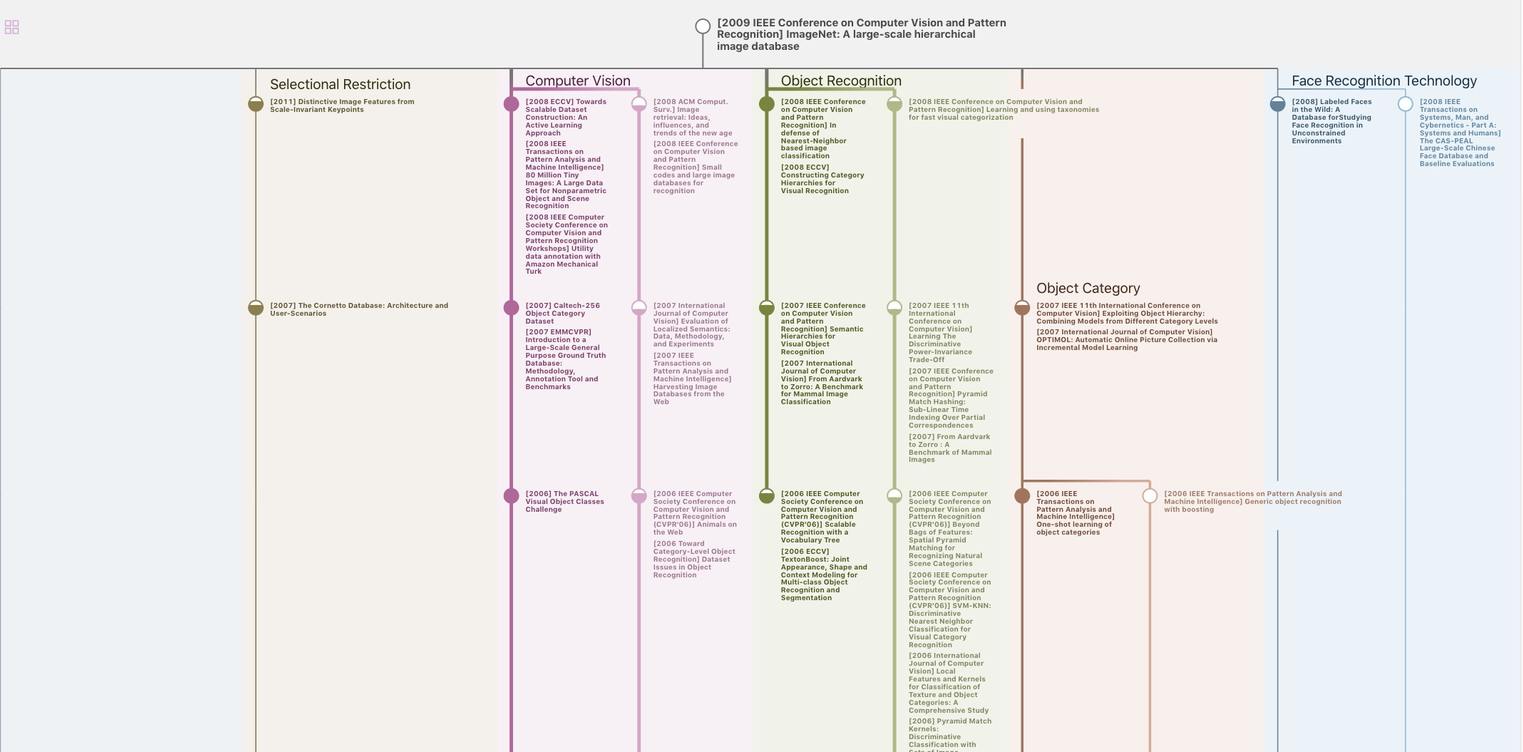
生成溯源树,研究论文发展脉络
Chat Paper
正在生成论文摘要