Using Decision Models to Decompose Anxiety-Related Bias in Threat Classification.
EMOTION(2016)
摘要
Individuals with high levels of anxiety show preferential processing of threatening information, and this cognitive bias is thought to be an integral component of anxiety disorders. In threat classification tasks, this bias manifests as high-anxiety participants being more likely to classify stimuli as threatening than their low-anxiety counterparts. However, it is unclear which cognitive mechanisms drive this bias in threat classification. To better understand this phenomenon, threat classification data were analyzed with 2 decision models: a signal detection model and a drift-diffusion model. Signal detection models can dissociate measures of discriminability and bias, and diffusion models can further dissociate bias due to response preparation from bias due to stimulus evaluation. Individuals in the study completed a trait anxiety measure and classified threatening and neutral words based on whether they deemed them threatening. Signal detection analysis showed that high-anxiety participants had a bias driven by a weaker threat criterion than low-anxiety participants, but no differences in discriminability. Drift-diffusion analysis further decomposed the threat bias to show that it is driven by both an expectation bias that the threat response was more likely to be correct, and a stimulus bias driven by a weaker criterion for evaluating the stimuli under consideration. These model-based analyses provide valuable insight and show that multiple cognitive mechanisms underlie differential threat processing in anxiety. Implications for theories of anxiety are discussed.
更多查看译文
关键词
trait anxiety,threat bias,signal detection theory,drift-diffusion model
AI 理解论文
溯源树
样例
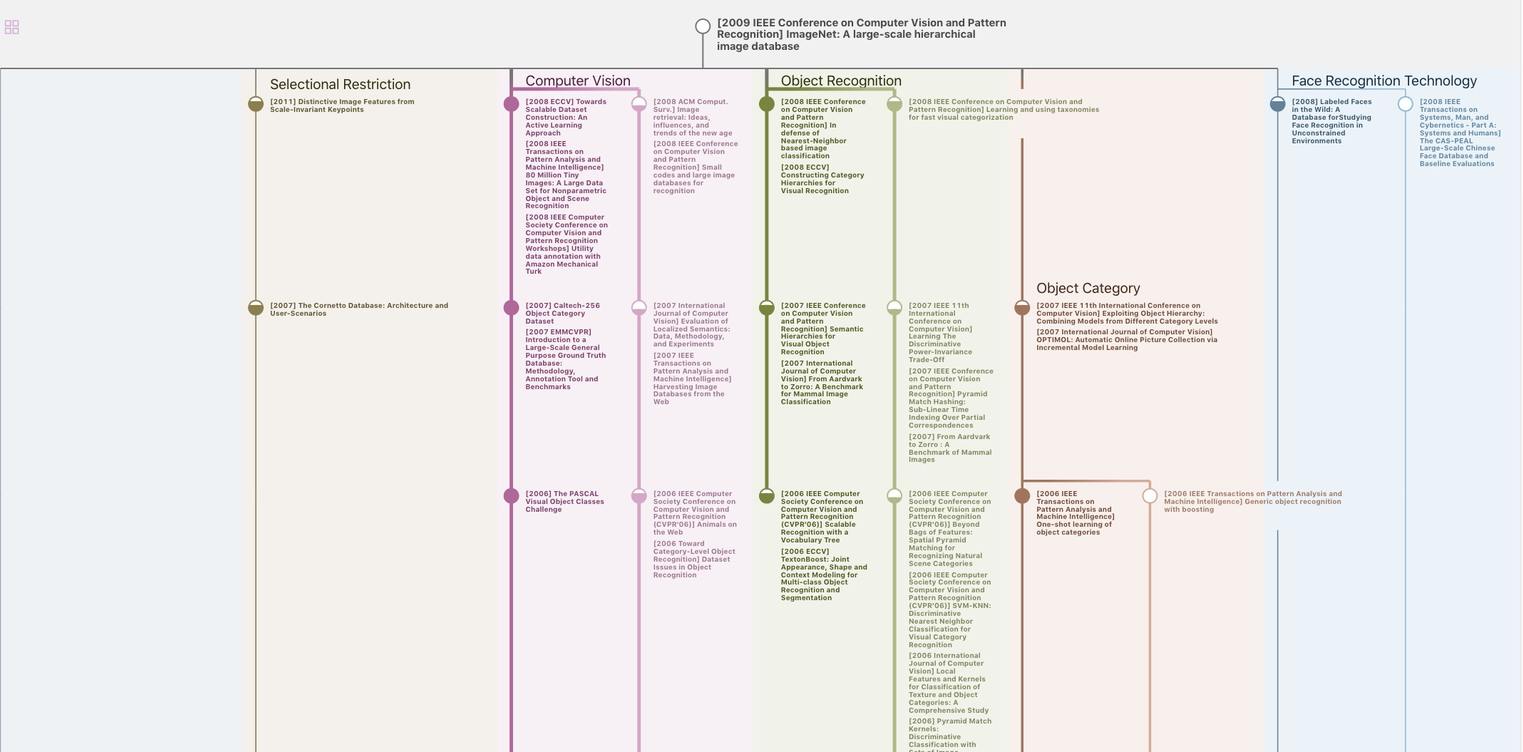
生成溯源树,研究论文发展脉络
Chat Paper
正在生成论文摘要