Using self-organizing maps to accelerate similarity search.
Bioorganic & Medicinal Chemistry(2012)
摘要
While self-organizing maps (SOM) have often been used to map and describe chemical space, this paper focuses on their use to accelerate similarity searches based on vectors of high-dimensional real-value descriptors for which classical, binary fingerprint-based similarity speed-up procedures do not apply. Fuzzy tricentric pharmacophore (FPT) and ISIDA substructure counts are herein explored examples. Similarity search speed-up was achieved by positioning compounds on a SOM, then searching for analogues only in the neurons neighbouring the ones in which the query compounds reside. Smaller neighbourhood means shorter virtual screening (VS) time, but lower analogue retrieval rates. An enhancement criterion, conciliating the opposite trends is defined. It depends on map definition and build-up protocol (training set choice, map size, convergence criteria,...). The main goal is to discover and validate SOMs of optimal quality with respect to this criterion. Increasing the size of the training set beyond a certain limit is shown to be unnecessary and even detrimental, suggesting that one SOM built on a relatively small but diverse training set may be an effective VS enhancer of a much larger database. Also, using an excessively large number of training iterations may lead to over-fitting. Gradual training with en-route checking of VS enhancement propensity is the best strategy to follow. Maps were successfully challenged to accelerate the large-scale VS of 12,000 queries against 160,000 compounds, and shown to provide a meaningful mapping of activity-annotated compounds in chemical space. (C) 2012 Elsevier Ltd. All rights reserved.
更多查看译文
关键词
SOM,VS,VLH,B,R,HR,FPT,DB,QS
AI 理解论文
溯源树
样例
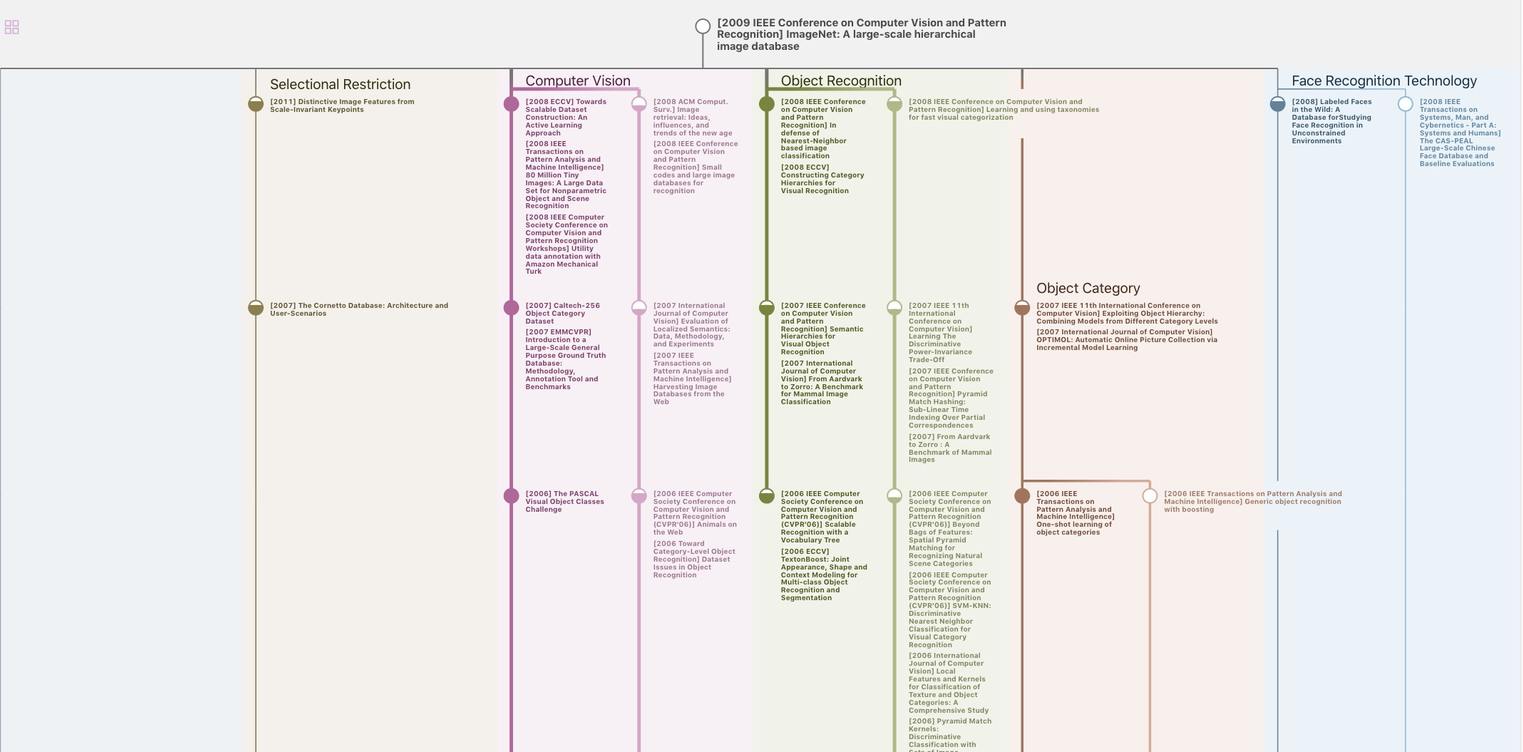
生成溯源树,研究论文发展脉络
Chat Paper
正在生成论文摘要