The Social Network (Of Protein Conformations)
PROCEEDINGS OF THE NATIONAL ACADEMY OF SCIENCES OF THE UNITED STATES OF AMERICA(2011)
摘要
Proteins possess thousands of degrees of freedom, and yet, they can rapidly and reliably find their way into well-defined folded configurations (1). In the cell, these folded proteins carry out highly specific motions critical to cargo transport and force transduction, energy generation, and catalysis. However, how is this possible given their incongruously large conformation spaces? Is their behavior intrinsically complex, where the nuanced details of all of the interatomic interactions are critical to their behavior, or can this motion be understood in terms of simpler collective behavior? In PNAS, Ceriotti et al. (2) examine this question by formulating an algorithm that attempts to uncover simple collective behavior in the conformation spaces of biomolecules in an automated fashion. Over the last few decades, a number of sophisticated computer simulation techniques have been developed that allow these conformation spaces to be explored, generating compendia of thousands or millions of conformational snapshots in a typical computer experiment. However, as computer hardware and clever software have advanced, making sense of these datasets has become harder and not easier. When it might have sufficed for a skilled structural biologist with a graphics terminal to extract insight into molecular mechanism from picoseconds to nanoseconds of molecular dynamics, it is no longer possible when these trajectories are microseconds to milliseconds in length or if the sampled ensembles represent a substantial fraction of the thermodynamically accessible conformation space. To make sense of these large datasets and learn something useful about the molecular motions that they represent, some form of data reduction is necessary. In one form or another, dimensionality reduction methods have been a workhorse of data visualization and analysis for centuries. By transforming a high-dimensional representation into a representation in only a few dimensions—generally two or three to facilitate human comprehension—these approaches can often provide useful insight …
更多查看译文
关键词
bioinformatics,biomedical research
AI 理解论文
溯源树
样例
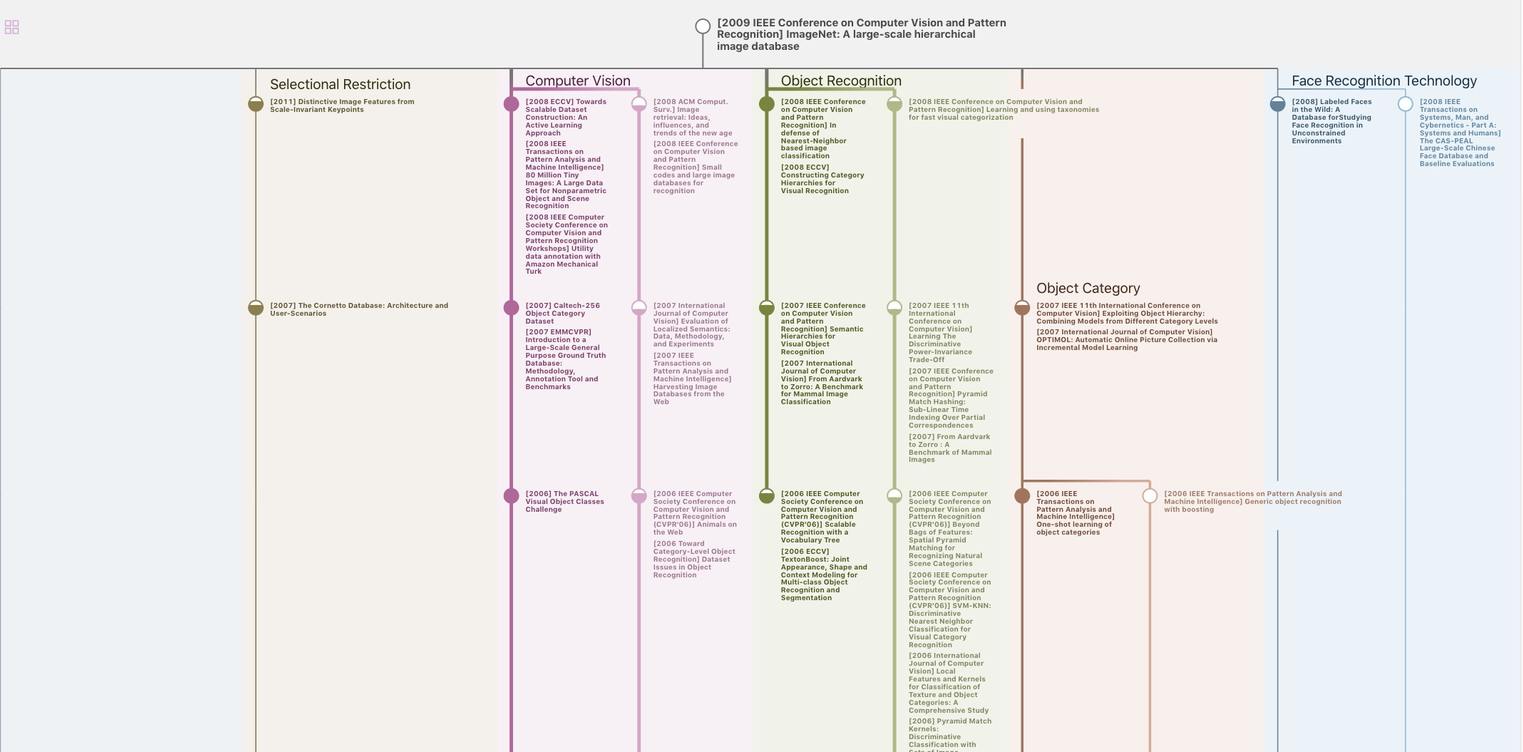
生成溯源树,研究论文发展脉络
Chat Paper
正在生成论文摘要