Scale-preserving long-term visual odometry for indoor navigation
Indoor Positioning and Indoor Navigation(2012)
摘要
We present a visual odometry system for indoor navigation with a focus on long-term robustness and consistency. As our work is targeting mobile phones, we employ monocular SLAM to jointly estimate a local map and the device's trajectory. We specifically address the problem of estimating the scale factor of both, the map and the trajectory. State-of-the-art solutions approach this problem with an Extended Kalman Filter (EKF), which estimates the scale by fusing inertial and visual data, but strongly relies on good initialization and takes time to converge. Each visual tracking failure introduces a new arbitrary scale factor, forcing the filter to re-converge. We propose a fast and robust method for scale initialization that exploits basic geometric properties of the learned local map. Using random projections, we efficiently compute geometric properties from the feature point cloud produced by the visual SLAM system. From these properties (e.g., corridor width or height) we estimate scale changes caused by tracking failures and update the EKF accordingly. As a result, previously achieved convergence is preserved despite re-initializations of the map. To minimize the time required to continue tracking after failure, we perform recovery and re-initialization in parallel. This increases the time available for recovery and hence the likelihood for success, thus allowing almost seamless tracking. Moreover, fewer re-initializations are necessary. We evaluate our approach using extensive and diverse indoor datasets. Results demonstrate that errors and convergence times for scale estimation are considerably reduced, thus ensuring consistent and accurate scale estimation. This enables long-term odometry despite of tracking failures which are inevitable in realistic scenarios.
更多查看译文
关键词
Kalman filters,SLAM (robots),distance measurement,indoor radio,mobile radio,navigation,nonlinear filters,EKF,extended Kalman filter,feature point cloud,indoor navigation,local map estimation,mobile phones,monocular SLAM,random projections,scale estimation,scale-preserving long-term visual odometry,visual SLAM system,visual data,visual odometry system,visual tracking failure
AI 理解论文
溯源树
样例
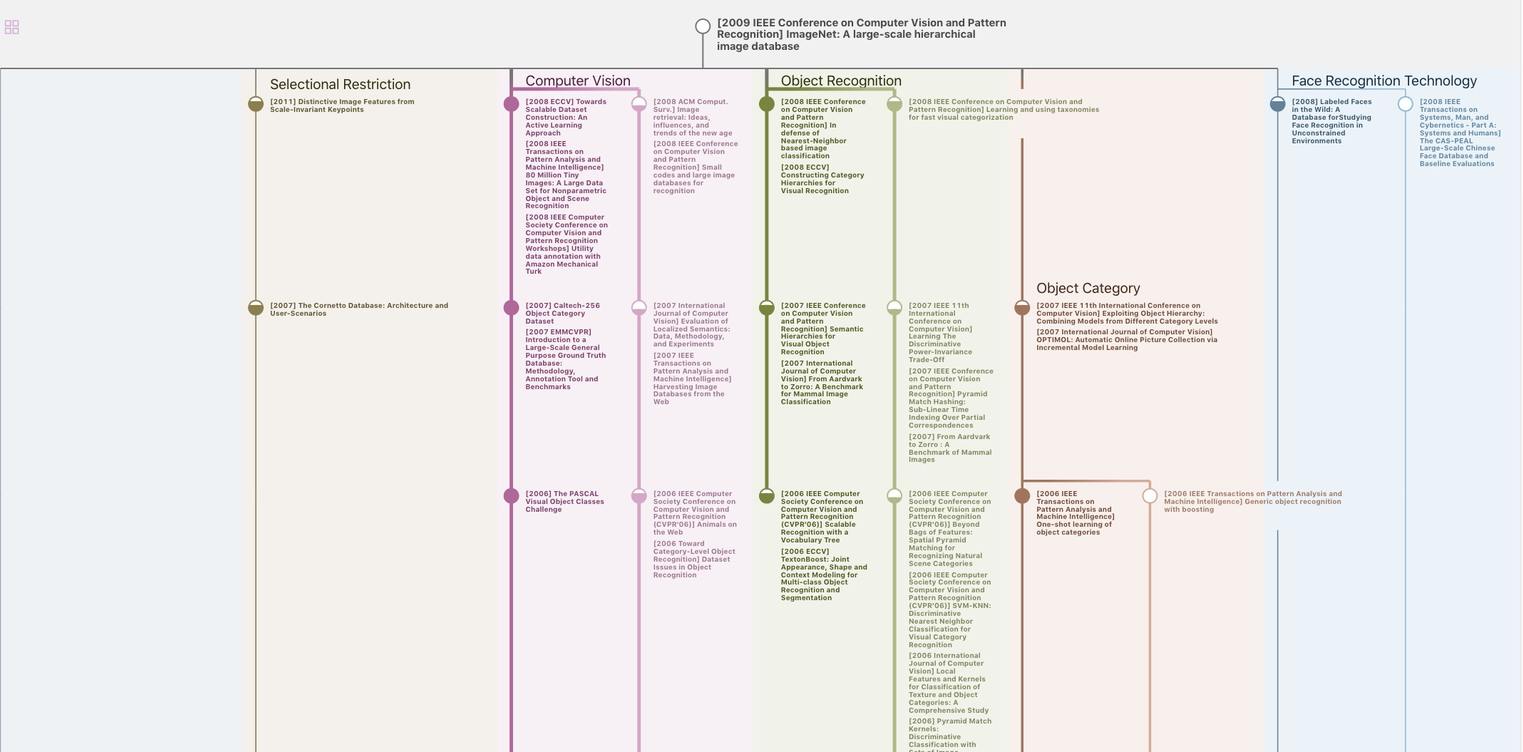
生成溯源树,研究论文发展脉络
Chat Paper
正在生成论文摘要