Multi-period marketing-mix optimization with response spike forecasting
IBM Journal of Research and Development(2014)
摘要
Optimizing the marketing budget and its distribution across various channels and target segments, along with the timing of each marketing action for maximum profit, are among the essential problems in marketing. A crucial requirement for effective decision making in direct marketing is an efficient optimization algorithm, coupled with reliable predictions on how the various marketing actions will impact consumers' purchase decisions. For maximizing mid-term revenues, while properly incorporating the temporal dynamics of individual consumers' mental states, we introduce a comprehensive optimization framework for obtaining a state-based marketing-mix policy, with a novel combination of machine learning, optimization, and simulation. Each consumer's purchase decision is modeled with a marked point process whose discrete-state approximation enables efficient optimization of high-dimensional decision variables, and whose discrete event simulation supplies accurate forecasts of the revenues and risks of the optimized policy. The key idea for ensuring the practicality of the proposed approach to marketing planning is a linear programming formulation that allows both handling of temporal dynamics and complex budget constraints across many periods, segments, and channels required in real marketing operations. A real-world case study based on an online retailing dataset implies high revenue gains when the simulation model can be relied on.
更多查看译文
关键词
Market research,Economics,Sales and marketing,Budgets,Decision making,Forecasting,Consumer behavior,Consumer purchases,Risk assessment
AI 理解论文
溯源树
样例
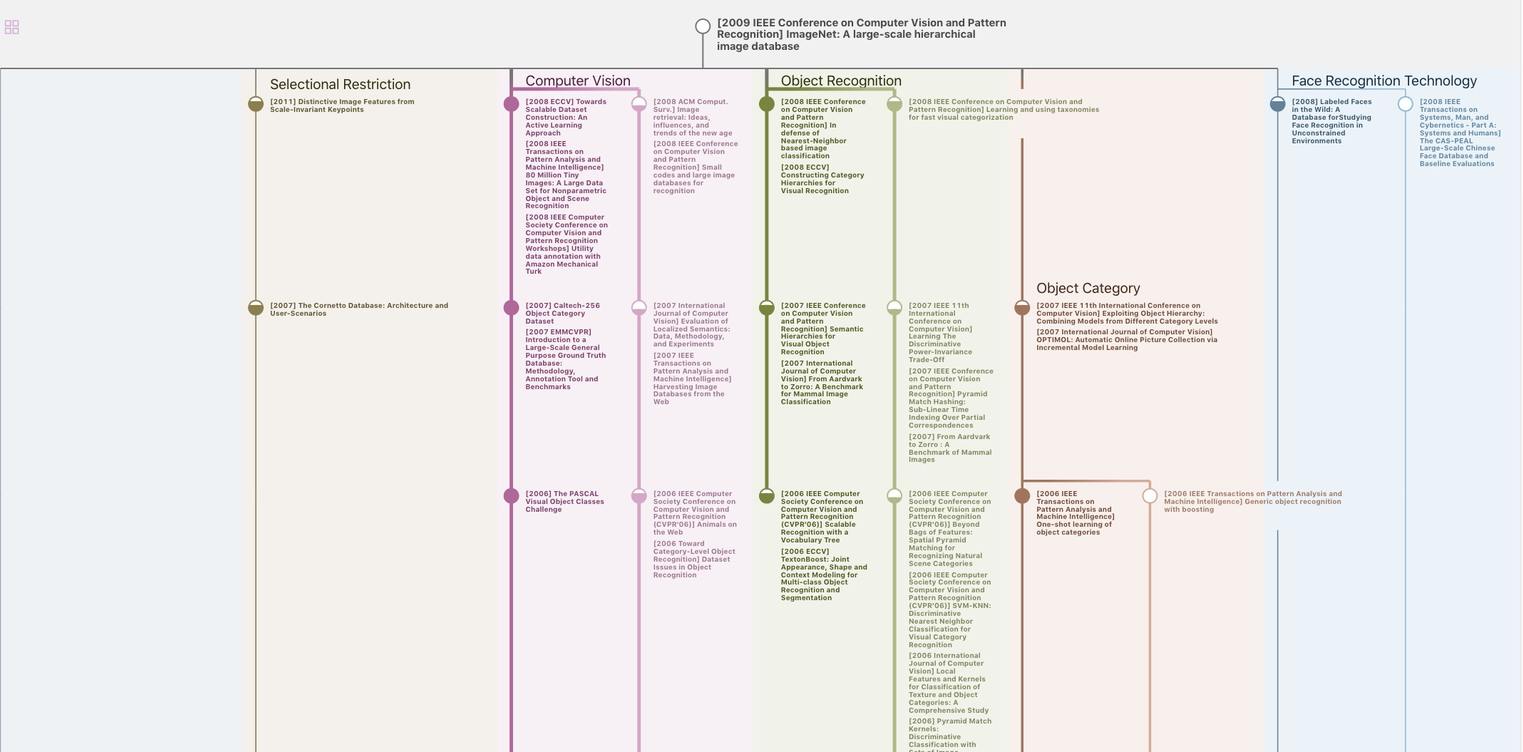
生成溯源树,研究论文发展脉络
Chat Paper
正在生成论文摘要