Sparse distance learning for object recognition combining RGB and depth information
Robotics and Automation(2011)
摘要
In this work we address joint object category and instance recognition in the context of RGB-D (depth) cameras. Motivated by local distance learning, where a novel view of an object is compared to individual views of previously seen objects, we define a view-to-object distance where a novel view is compared simultaneously to all views of a previous object. This novel distance is based on a weighted combination of feature differences between views. We show, through jointly learning per-view weights, that this measure leads to superior classification performance on object category and instance recognition. More importantly, the proposed distance allows us to find a sparse solution via Group-Lasso regularization, where a small subset of representative views of an object is identified and used, with the rest discarded. This significantly reduces computational cost without compromising recognition accuracy. We evaluate the proposed technique, Instance Distance Learning (IDL), on the RGB-D Object Dataset, which consists of 300 object instances in 51 everyday categories and about 250,000 views of objects with both RGB color and depth. We empirically compare IDL to several alternative state-of-the-art approaches and also validate the use of visual and shape cues and their combination.
更多查看译文
关键词
distance learning,image colour analysis,image sensors,object recognition,RGB color,RGB-D cameras,RGB-D object dataset,depth information,group-lasso regularization,instance distance learning,object category,object recognition,sparse distance learning,view-to-object distance
AI 理解论文
溯源树
样例
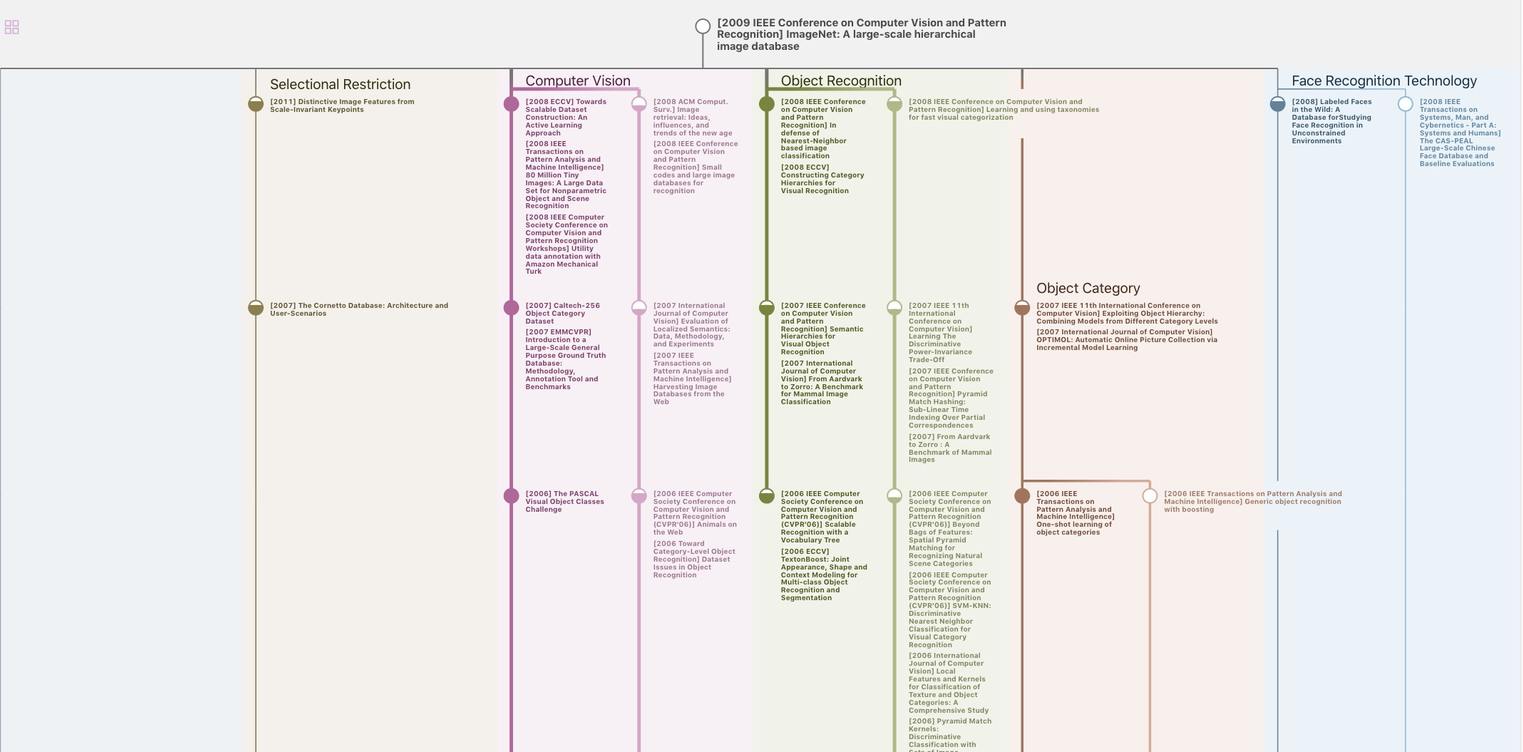
生成溯源树,研究论文发展脉络
Chat Paper
正在生成论文摘要