Interpretable knowledge acquisition for predicting DNA-binding domains using an evolutionary fuzzy classifier method
CSAE), 2011 IEEE International Conference(2011)
摘要
DNA-binding domains are functional proteins in a cell, which plays a vital role in various essential biological activities. It is desirable to predict and analyze novel proteins from protein sequences only using machine learning approaches. Numerous prediction methods were proposed by identifying informative features and designing effective classifiers. The support vector machine (SVM) is well recognized as an accurate and robust classifier. However, the block-box mechanism of SVM suffers from low interpretability for biologists. It is better to design a prediction method using interpretable features and prediction results. In this study, we propose an interpretable physicochemical property classifier (named iPPC) with an accurate and compact fuzzy rule base using a scatter partition of feature space for DNA-binding data analysis. In designing iPPC, the flexible membership function, fuzzy rule, and physicochemical properties selection are simultaneously optimized. An intelligent genetic algorithm IGA is used to efficiently solve the design problem with a large number of tuning parameters to maximize prediction accuracy, minimize the number of features selected, and minimize the number of fuzzy rules. Using benchmark datasets of DNA-binding domains, iPPC obtains the training accuracy of 81% and test accuracy of 79% with three fuzzy rules and two physicochemical properties. Compared with the decision tree method with a training accuracy of 77%, iPPC has a more compact and interpretable knowledge base. The two physicochemical properties are Number of hydrogen bond donors and Helix-coil equilibrium constant in the AAindex database.
更多查看译文
关键词
dna,biology computing,data analysis,fuzzy set theory,genetic algorithms,knowledge acquisition,knowledge based systems,learning (artificial intelligence),pattern classification,proteins,support vector machines,aaindex database,dna-binding data analysis,dna-binding domain prediction,helix-coil equilibrium constant,evolutionary fuzzy classifier method,flexible membership function,fuzzy rule base,hydrogen bond donors,ippc,intelligent genetic algorithm,interpretable physicochemical property classifier,knowledge base,machine learning approach,protein sequences,support vector machine,dna-binding,fuzzy classifier,genetic algorithm,knowledge acquistion,physicochemical properties,prediction,amino acids,protein sequence,feature selection,accuracy,dna binding domain,feature space,decision tree,bioinformatics,learning artificial intelligence,membership function,amino acid,biological activity,hydrogen bond,equilibrium constant,machine learning
AI 理解论文
溯源树
样例
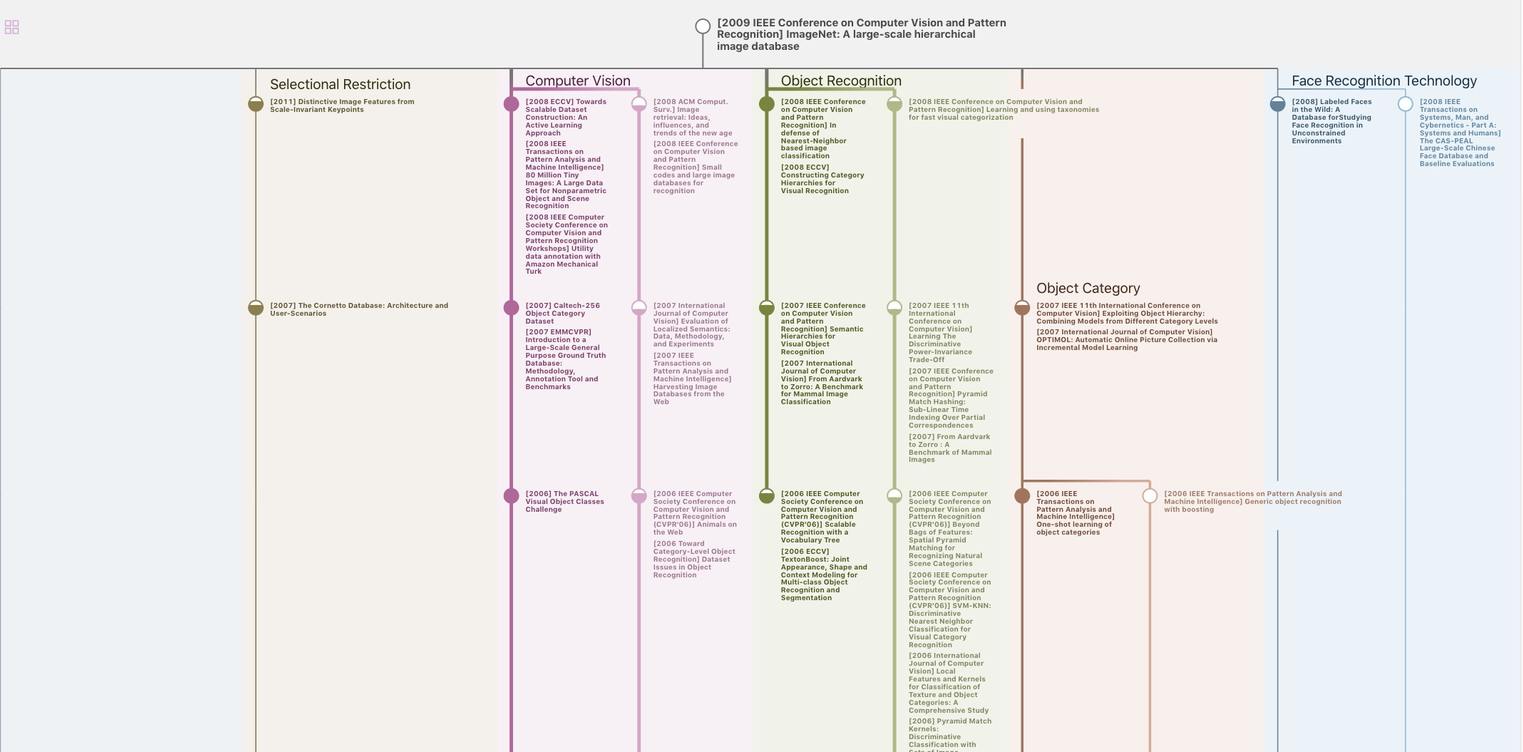
生成溯源树,研究论文发展脉络
Chat Paper
正在生成论文摘要