Underdetermined model-based blind source separation of reverberant speech mixtures using spatial cues in a variational Bayesian framework
Intelligent Signal Processing Conference 2013(2013)
摘要
In this paper, we propose a new method for underdetermined blind source separation of reverberant speech mixtures by classifying each time-frequency (T-F) point of the mixtures according to a combined variational Bayesian model of spatial cues, under sparse signal representation assumption. We model the T-F observations by a variational mixture of circularly-symmetric complex-Gaussians. The spatial cues, e.g. interaural level difference (ILD), interaural phase difference (IPD) and mixing vector cues, are modelled by a variational mixture of Gaussians. We then establish appropriate conjugate prior distributions for the parameters of all the mixtures to create a variational Bayesian framework. Using the Bayesian approach we then iteratively estimate the hyper-parameters for the prior distributions by optimizing the variational posterior distribution. The main advantage of this approach is that no prior knowledge of the number of sources is needed, and it will be automatically determined by the algorithm. The proposed approach does not suffer from overfitting problem, as opposed to the Expectation-Maximization (EM) algorithm, therefore it is not sensitive to initializations.
更多查看译文
关键词
gaussian distribution,blind source separation,expectation-maximisation algorithm,speech processing,variational techniques,circularly symmetric complex gaussians,expectation maximization algorithm,interaural level difference,interaural phase difference,mixing vector cues,reverberant speech mixtures,sparse signal representation,spatial cues,time frequency point,underdetermined model based blind source separation,variational bayesian framework,variational posterior distribution
AI 理解论文
溯源树
样例
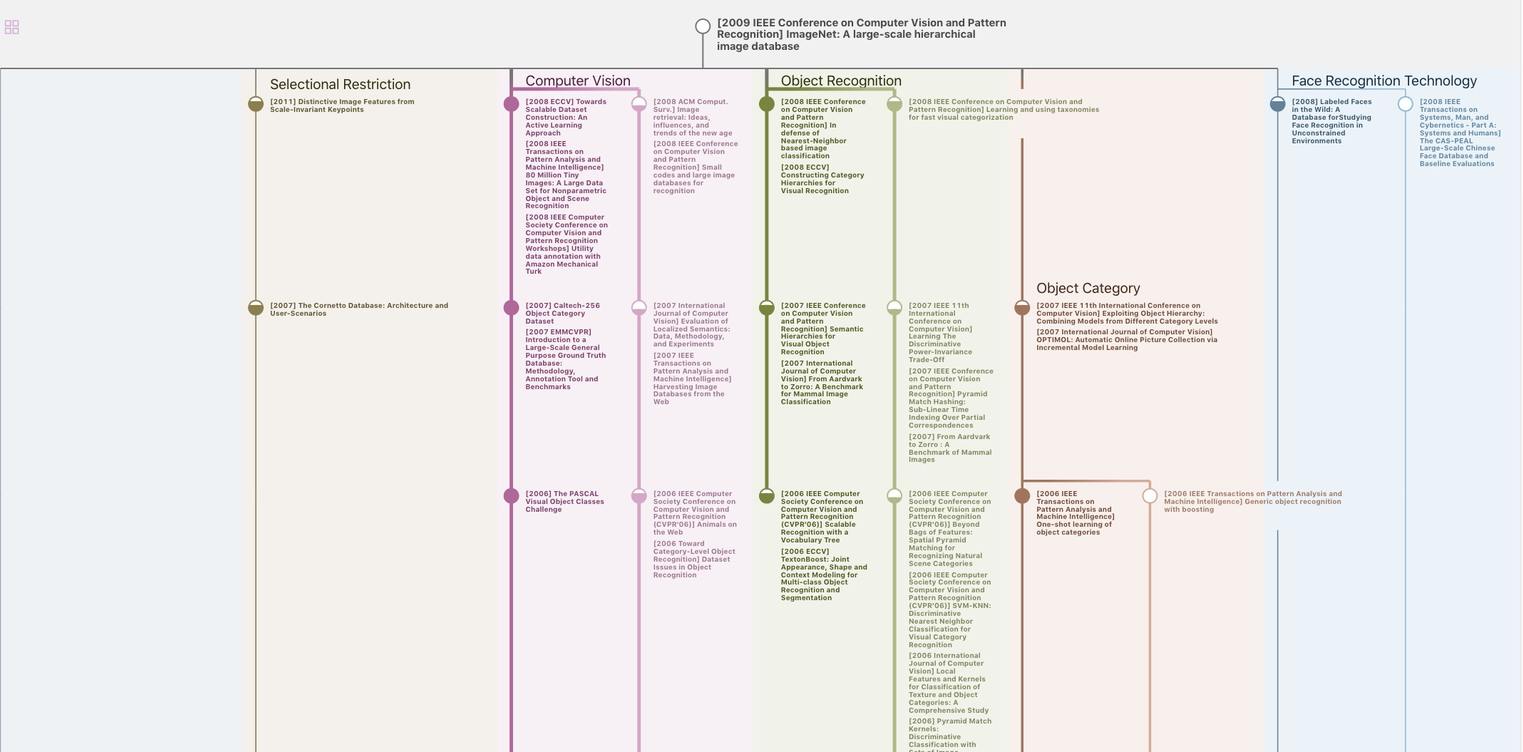
生成溯源树,研究论文发展脉络
Chat Paper
正在生成论文摘要