On stochastic gradient descent and quadratic mutual information for image registration
Image Processing(2013)
摘要
Mutual information (MI) is quite popular as a cost function for intensity based registration of images due to its ability to handle highly non-linear relationships between intensities of the two images. More recently, quadratic mutual information (QMI) has been proposed as an alternative measure that computes Euclidean distance instead of KL divergence between the joint and the product of the marginal densities of pixel intensities. In this paper, we examine the conditions under which QMI is advantageous over the classical MI measure, for the image registration problem. We show that QMI is a better cost function to use for optimization methods such as stochastic gradient descent. We show that the QMI cost function remains much smoother than the classical MI measure on stochastic subsampling of the image data. As a consequence, QMI has a higher probability of convergence, even for larger degrees of initial misalignment of the images.
更多查看译文
关键词
image registration,image sampling,optimisation,stochastic processes,Euclidean distance,QMI,image data,image registration,optimization methods,quadratic mutual information,stochastic gradient descent,stochastic subsampling,Mutual information,image registration
AI 理解论文
溯源树
样例
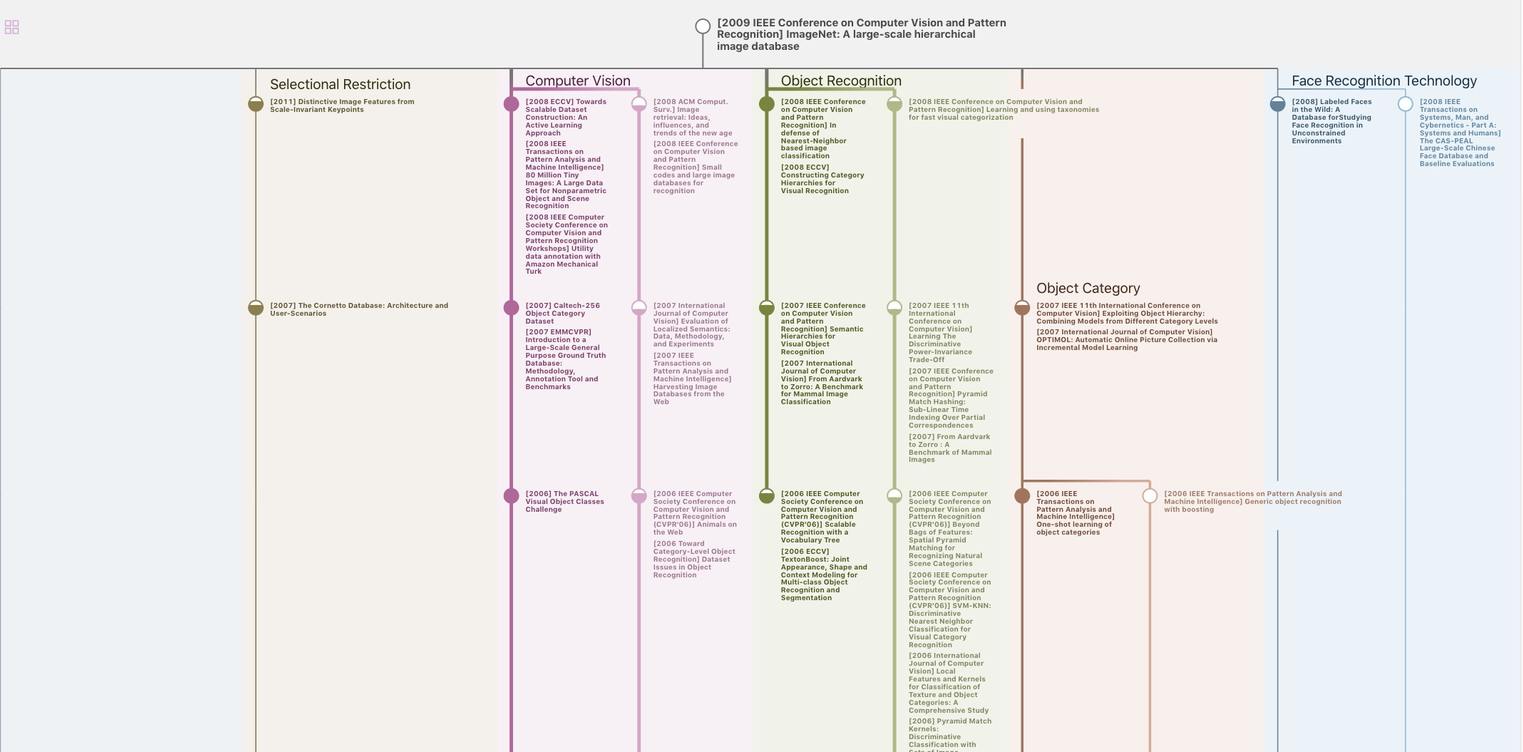
生成溯源树,研究论文发展脉络
Chat Paper
正在生成论文摘要