Adaptive search for sparse dynamic targets
Computational Advances in Multi-Sensor Adaptive Processing(2013)
摘要
We consider the problem of energy constrained and noise-limited search for targets that are sparsely distributed over a large area. We propose a multiple-stage search algorithm that accounts for complex time-varying target behavior such as transitions among neighboring cells and varying target amplitudes. This work extends the adaptive resource allocation policy (ARAP) introduced in [Bashan et al, 2008] to policies with T ≫ 2 stages. The proposed search strategy is driven by minimization of a surrogate function for energy constrained mean-squared error within locations containing targets. Exact optimization of the multi-stage objective function is infeasible, but myopic optimization yields a closed-form solution. We extend the myopic solution with non-myopic considerations that save a percentage of resources for exploring the scene at large. Empirical evidence suggests that the non-myopic policy performs significantly better than the myopic solution in terms of estimation error, probability of detection, and robustness to model mismatch. Moreover, the provided search policy has low computational complexity compared to state-of-the-art dynamic programming solutions.
更多查看译文
关键词
computational complexity,dynamic programming,mean square error methods,search problems,target tracking,adaptive resource allocation policy,adaptive search,closed-form solution,complex time-varying target behavior,computational complexity,detection probability,dynamic programming solutions,energy constrained mean-squared error,energy constrained problem,estimation error,multiple-stage search algorithm,multistage objective function,myopic optimization,myopic solution,neighboring cells,noise-limited search,nonmyopic considerations,search policy,sparse dynamic targets
AI 理解论文
溯源树
样例
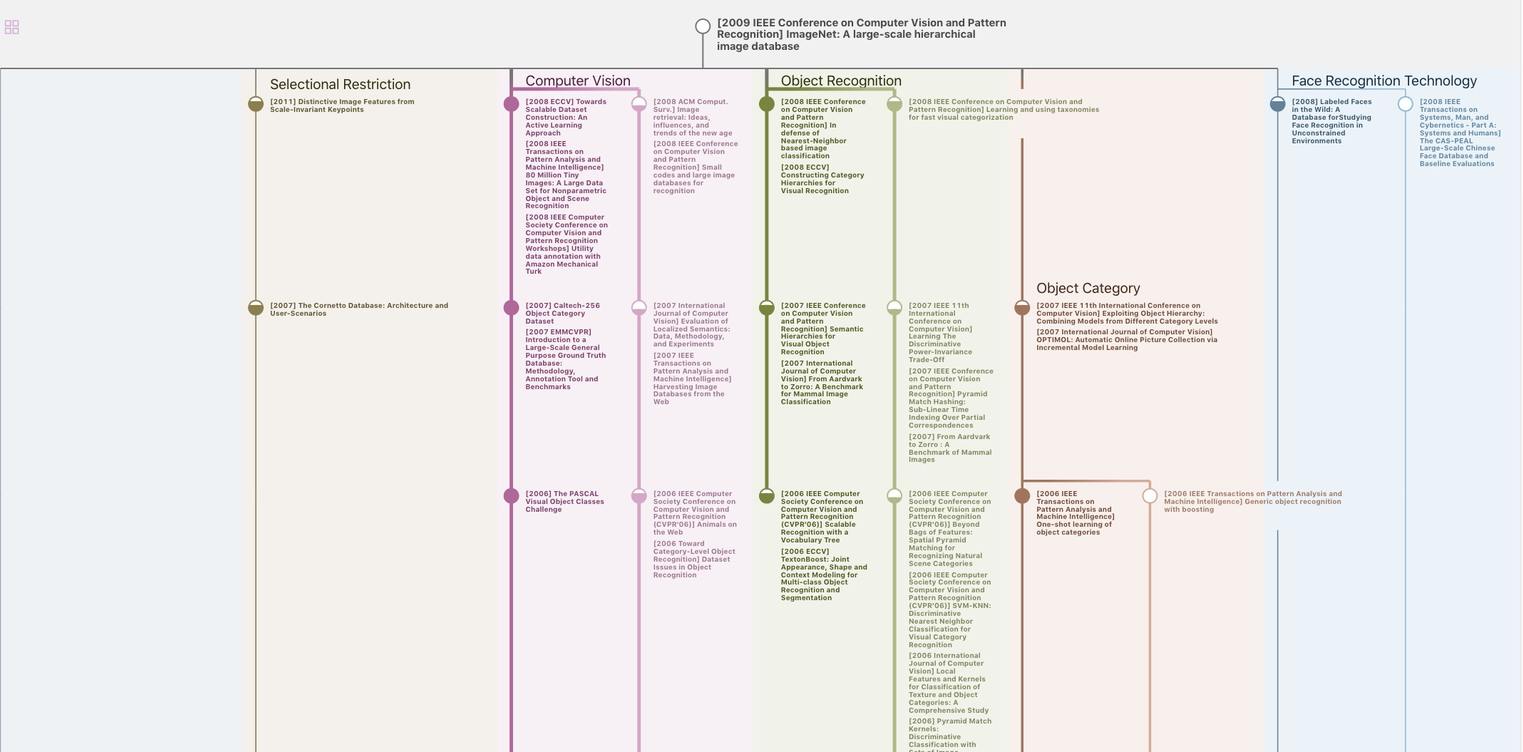
生成溯源树,研究论文发展脉络
Chat Paper
正在生成论文摘要